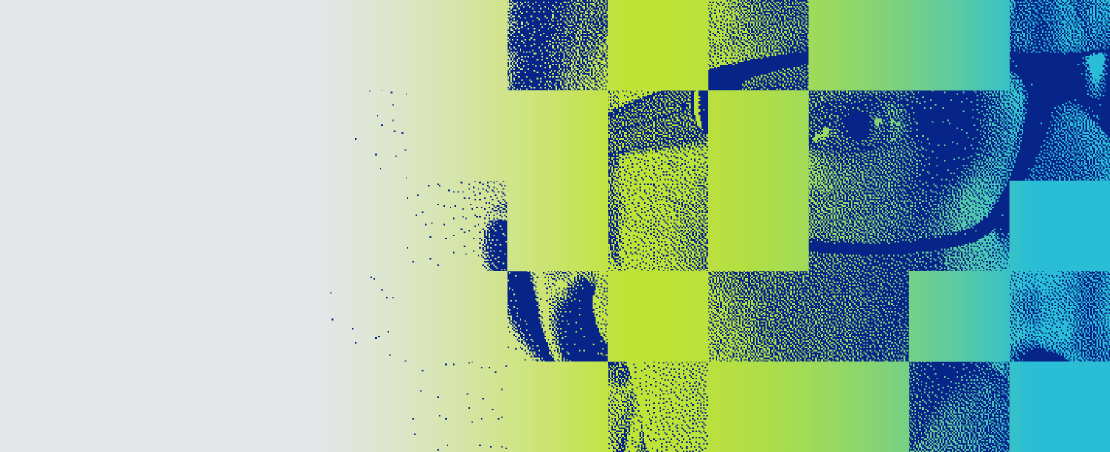
Over the past 18 months, the UK – along with many other countries – has been wrestling to understand what legislation and regulation are needed to govern generative AI and other rapidly evolving technologies. The age of foundation AI is characterised by models of large scale and high flexibility, underpinning systems capable of having complex interactions and producing rich outputs. There are risks, too, as have been reflected in the UK-hosted AI Safety Summit at Bletchley Park, and the establishment of the AI Safety Institute. Recognising both the potential and the risks, the ODI has embarked on a programme of work on Data-centric AI designed to bring about an AI ecosystem grounded in responsible data practices.
Governments have a significant role to play here, from introducing new laws to govern the use of data to train AI, to stimulating investment and innovation in data assurance and sharing, to using data and AI themselves - in a transparent manner - to deliver public services. As part of our work, we have explored what policy interventions need to be made to ensure that these new technologies are developed and deployed in a way that benefits everyone - people, communities and businesses.
This is the first in a series of five pieces exploring these policy interventions, and how they can help to positively shape the landscape.
What is transparency for training data?
Training data is the data used to train an artificial intelligence (AI) model. According to the assessments of the Stanford Foundation Model Transparency Index, transparency for training data involves disclosing things such as:
- The size of the dataset
- The source of the data
- Who created the data
- How the data was created
- How the dataset has been augmented - and for what purpose
- How the dataset has been filtered (e.g. for harmful content)
- Whether the dataset includes copyrighted data
- What licence the data can be used under
- Any personal information included in the data
We’re talking primarily here about training data, fine-tuning and preference data, as well as other data artefacts – we’ll focus on the availability of training data itself in a later policy principle.
Why is training data transparency important?
Most leading AI firms have refused to disclose details about the data they’ve used to train and test AI models. The Stanford Foundation Model Transparency index, which assesses the major foundational models that provide the backbone of many AI tools and services, demonstrated that transparency regarding the data used was very low compared to other aspects of transparency. In documentation published at the launch of its GPT-4 model, OpenAI stated that it wouldn’t share detailed information about ‘dataset construction’ and other aspects of the model’s development due to 'the competitive landscape and the safety implications of large-scale models' – a decision that was roundly criticised by a number of leading researchers.
Which data is used to build AI systems is important; but how well those developing, deploying and using AI systems understand biases, limitations, and legal obligations associated with the use of this data is equally crucial to ensure systems are implemented responsibly. Further downstream, the users of AI systems and those impacted by their use are far more likely to trust them if they understand how they’ve been developed. In theory, should the system be explained correctly, ‘the user should know when to trust the system’s predictions and when to apply their own judgement’.
However, in their analysis, a Washington Post investigation concluded that ‘many companies do not document the contents of their training data – even internally - for fear of finding personal information about identifiable individuals, copyrighted material and other data grabbed without consent’. Hence, when Scarlett Johannson publicly called out OpenAI for allegedly using her voice in a new chatbot – she specifically called for ‘resolution in the form of transparency’. The Data Provenance Explorer explores how most AI development happens through fine-tuning and few shot learning of pre-trained models. In fact, in the UK, most tech providers and companies using AI will be mostly probably fine-tuning – rather than training. Transparency of fine-tuning data is key, yet it is often just as opaque as training data.
Lawmakers and regulators need to be able to assess the data upon which these models are built to ensure they comply with legislation. As put by Eryk Salvaggio, ‘flying a commercial airliner full of untested experimental fuel is negligence. Rules asking airlines to tell us what’s in the fuel tank do not hamper innovation. Deploying models in the public sphere without oversight is negligence too’.
Current policy status in the UK and elsewhere
The UK currently emphasises a flexible, sector-specific approach to AI regulation rather than a singular, overarching framework like the EU AI Act. This reflects the UK's historical approach to regulating emerging technologies. However, this stance could change under a new government. In 2023, the UK Government established the AI Safety Institute to focus on ‘advanced AI safety for the public interest’. One of its key roles is facilitating information exchange with national and international entities, adhering to existing privacy and data regulations. This includes sharing data on training and fine-tuning AI systems, which is crucial for the Institute's function of conducting AI system evaluations.
In March 2024, a private members' bill was introduced in the House of Lords requiring AI providers to share information about their training data with a central 'AI Authority,' ensure informed consent when gathering training data, and undergo mandatory audits. However, the bill did not progress after Parliament was prorogued in May 2024. AI is likely to be a talking point in the 2024 General Election, with the Labour Party signalling previously that it would mandate AI firms to share their test data with the UK government if it comes to power.
While the UK has taken a flexible approach, other jurisdictions like the US, EU, and Japan have different stances. In the US, the 2023 Federal Trade Commission ordered OpenAI to document all data sources used for training its models. The proposed AI Foundation Model Transparency Act calls for the FTC to establish standards for publicising training data information. The EU AI Act mandates detailed summaries of training data content to ensure transparency and protect rights holders. Japan's draft AI principle calls for data collection method transparency and data source traceability.
Proposals from civil society, industry and other non-government actors
Industry players are addressing transparency issues independently of regulatory approaches. Data governance frameworks are emerging, including fairness audits and dataset transparency. Developers are creating training data documentation tools like Hugging Face's Model Cards and Dataset Cards, Dataset Nutrition Labels, and the Data Provenance Initiative. Market-oriented solutions are also being developed, such as Adobe's transparency about its AI training content and the Data & Trust Alliance's Data Provenance Standards. Civil society organisations, like the Mozilla Foundation and Fairly Trained, are campaigning for regulatory changes to ensure transparency and fairness in AI training data use. In ‘Safe before Sale’, the Ada Lovelace Institute has argued that ‘regulators should compel mandatory model and dataset documentation and disclosure for the pre-training and fine-tuning of foundation models’.
Steps to take
In our recently launched policy manifesto - that received cross party support -, the ODI called on the UK Government to specifically and explicitly consider data in their principles for AI regulation.
Legislation like the proposed Private Members Bill, which puts obligations on AI developers to be transparent about their data, and provides regulators with the necessary powers to hold them to account (as part of an ‘AI Authority’, or as part of existing bodies and regulator), would be an ambitious but worthwhile goal for the new, incoming UK government. We recommend that the incoming UK Government:
- Encourages the adoption of dataset transparency tools and frameworks that are emerging from the AI community. Existing work on Fairly Trained, Dataset Cards and Nutrition labels should be more widely adopted in organisations building AI services - and the government should lead by example in adopting these transparency tools and frameworks. Further supporting the Croissant standard development, of which the ODI co-chairs the group, is also vital. There also needs to be consideration as to how these documentation practices – that are primarily aimed at the developer community – can also be adopted and empower non-technical specialists, organisations and communities.
- Bolsters the AI Safety Initiative to design new mandatory reporting requirements and standards. While developments from the developer community are welcome, it is important that this information about training, testing and fine-tuning data is made available by model developers in consistent, standardised ways, so that regulators and others can easily interpret it and compare the way different models have been trained. Academics Saffron Huang and Divya Siddarth have described the need for new standards-setting bodies to 'determine appropriate venues and forms of information release'. Any incoming government should capitalise on the international cooperation fostered by the AI Safety Summits and ensure that the UK regime connects with best practice from around the world.
- Doesn’t see training data as a singular, static artefact. As the researcher Margaret Mitchell has pointed out, even when companies have published information on the training data they’ve used, they’ve tended only to focus on ‘fine tuning’ data. This is important, as it’s the larger, messier ‘pre-training’ datasets that are most likely to include harmful content or copyrighted material. We need companies to publish detailed information on the composition and provenance of both. Going forward, we should also expect access to information about various types of data used to train and apply AI systems, including proprietary or local data used in the process of reinforcement learning, retrieval augmentation and deploying models.
At the ODI, we’re keen to provide insights and resources to policymakers working towards increasing transparency around the data used to train AI models, particularly to develop new, open standards or exploring ways to document the use of proprietary or local data. We will publish further proposed interventions in the coming weeks, focusing on intellectual property, data protection, the availability of data and participatory data practices.