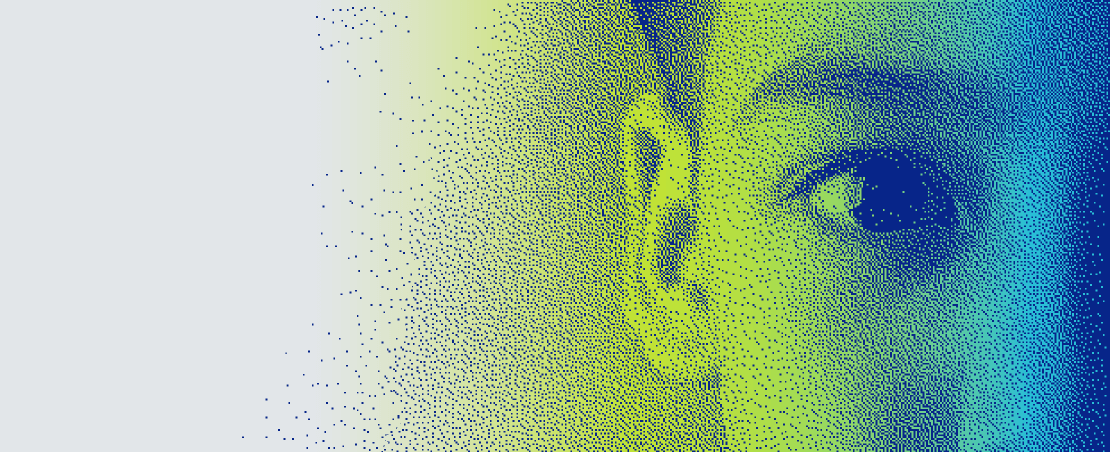
Generative AI has the potential to bridge the 'data divide' by making data and the tools to use it more accessible. These technologies can democratise information, allowing people without specialist skills to interact with and interpret data intuitively through conversational agents. However, improving AI and data literacy is essential to fully harness this potential, enabling users to make informed, data-enabled decisions. Indeed, all these tools work best with ‘humans in the loop’. To achieve these benefits, increased access to data is crucial, not only to accelerate innovation but also to enable society to realise the full value of data.
Despite this promise, there are substantial challenges within the AI ecosystem. The AI community currently relies on a handful of machine learning datasets, which lack robust governance frameworks. This poses significant risks, as inadequate data governance can lead to biases and unethical practices, undermining the trust and reliability of AI applications in critical areas such as healthcare, finance, and public services. The absence of standardisation and cohesive policy frameworks results in inconsistencies in data quality, transparency, and fairness, threatening the resilience of AI systems and risking a widescale collapse akin to a 'dot com moment' for AI.
Moreover, the lack of transparency about training data hampers efforts to address biases and ensure legal compliance. Standardised documentation, akin to financial reporting, is needed to enhance transparency and trust. Governments and stakeholders must act decisively, updating intellectual property laws, enforcing data protection, and ensuring broad data access. Empowering individuals in data sharing and use is vital to creating a transparent, equitable, and effective AI ecosystem.
Building on our recent manifesto, we're working on recommendations for policy interventions that will help build the strong data infrastructure essential for responsible AI, so watch this space over the coming weeks.