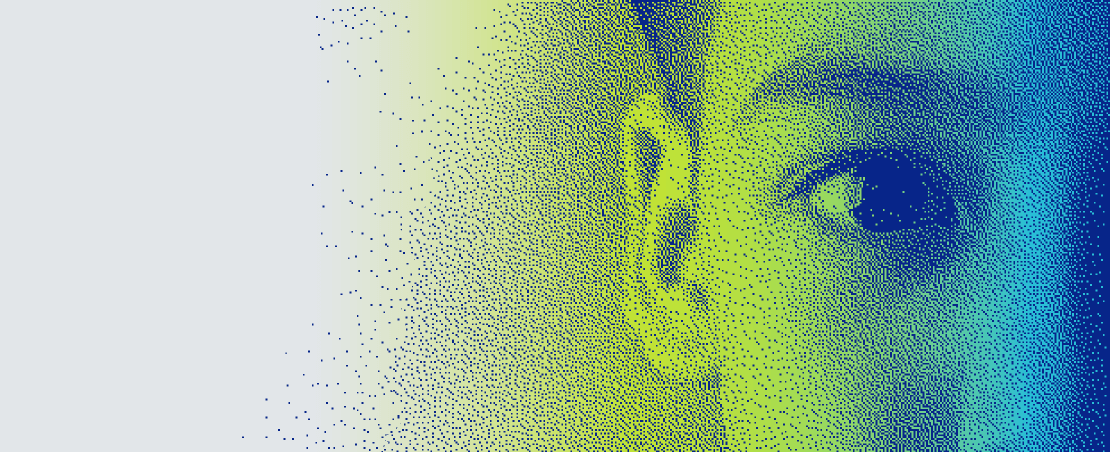
Artificial Intelligence (AI) technologies are transforming industries by leveraging large datasets to create predictive models through Machine Learning (ML), Natural Language Processing (NLP), and Computer Vision (CV). While these advancements enhance automation and decision-making, the performance and ethical deployment of these systems rely heavily on the governance of the underlying data.
As part of the ODI’s data-centric AI programme, this series examines the literature to highlight the critical role of robust data governance frameworks throughout AI development. Firstly, we focus on defining the AI data lifecycle stages, and secondly, the actors involved and the interactions between them, with both of these areas setting the key context for our main focus on AI data governance considerations that we cover in the final report.
Current AI data governance practices often lack standardisation, resulting in issues like poor data quality, biases, and security vulnerabilities, which erode trust in AI technologies. Our series introduces a data-centric approach to investigating these questions, emphasising key pillars of responsible governance like data quality as the foundation for effective and ethical AI systems, which is crucial for reducing risks and fostering public trust in the use of AI across sectors like healthcare, finance, and public services.
The first report, A lifecycle perspective, outlines the journey of data within AI systems, seeking to define the key stages from collection and preprocessing to training and deployment. It lays the foundation for understanding the importance of managing data at each stage to ensure ethical and effective AI/ML system development.
The second report, Exploring the ecosystem, explores the broader network of data interactions and the roles of various stakeholders. It highlights the importance of collaboration among, often multidisciplinary, practitioners who develop AI/ML systems, including data scientists, engineers, domain experts, and other stakeholders all contributing to understanding and governing data in different ways.
The third report, Mapping governance, synthesises insights from the first two, detailing AI data governance considerations according to the five pillars of data governance. It addresses gaps in current practices, finding several key insights on gaps in data access, documentation, and ethical considerations. This final report offers areas for further work by policymakers, practitioners, and researchers, promoting a holistic approach to data governance throughout the AI lifecycle.
Overall, we find several key recommendations for improving data governance in AI:
- Standardise documentation: Implement standardised documentation templates and guidelines to enhance consistency and facilitate knowledge sharing, improving transparency and accountability across the AI data lifecycle.
- Adopt FAIR principles: Implement the Findability, Accessibility, Interoperability, and Reusability (FAIR) principles in documentation practices to ensure datasets and models are discoverable and usable, promoting better data management and sharing.
- Interactive and automated documentation: Integrate documentation into existing tools and workflows to maintain accuracy and relevance, supporting continuous improvement and fostering collaboration among data science teams.
- Role-specific documentation: Explore and develop role-specific guidelines and training programs to ensure all stakeholders understand their documentation responsibilities, enhancing the overall efficiency and accuracy of the governance process.
- Incompleteness in mapping governance practices: Further research is needed to investigate data management practices during the evaluation and training stages, ensuring data integrity and security while developing reliable AI models.
- Downstream lifecycle stages: Implement robust data validation frameworks to continuously monitor and correct anomalies during the downstream stages of the AI data lifecycle.
- Limited guidance on data access governance: Explore advanced encryption techniques and secure data-access protocols to protect sensitive data during the evaluation and training stages, reducing the risk of data leaks.
- The practitioner landscape: Use role-based and attribute-based access controls to manage data accessibility, ensuring only authorised personnel can access sensitive data and maintaining data integrity and security.
- Beyond machine learning: a focus on broader AI systems: Renew focus on the development and governance of non-ML knowledge-based systems, ensuring that these systems are also integrated with robust data governance frameworks to enhance their reliability and ethical deployment.
Our research builds on initiatives like BigScience and BigCode to further understand how robust data governance enhances the responsible, data-centric development of AI/ML systems. We aim to contribute to the ongoing discourse on this growing area of data-centric AI, advocating for systems that are technologically advanced, ethically sound, and socially beneficial.
We invite stakeholders to engage with our findings and contribute to the discourse on responsible AI development. Our goal is to clarify AI data governance to support the development of systems that are technologically advanced, ethically sound, and beneficial to society. This series is a resource for anyone involved in or affected by AI technologies, providing valuable insights into responsible AI development.