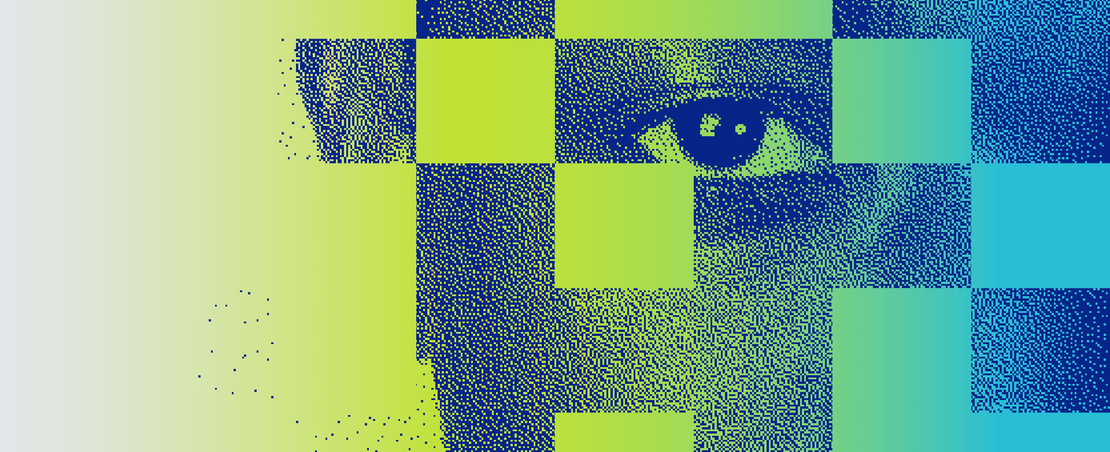
The transformative potential of Artificial Intelligence (AI) and Machine Learning (ML) systems continues to impact society, propelled by advancements in Natural Language Processing (NLP) and Computer Vision (CV). These technologies have seen significant progress in recent years, leading to a surge in their applications and capabilities. These technologies, which leverage vast datasets to learn patterns and through predictive models, herald an era of enhanced automation and decision-making capabilities. However, the performance of AI models is linked not only to engineering aspects but also to the data quality and the governance frameworks that guide how this data is collected, used and managed.
This report synthesises insights from our first two reports in the Understanding data governance in AI series, detailing AI data governance considerations according to the five pillars of data governance. It addresses gaps in current practices, finding several key insights on gaps in data access, documentation, and ethical considerations. This final report offers areas for further work by policymakers, practitioners, and researchers, promoting a holistic approach to data governance throughout the AI lifecycle.