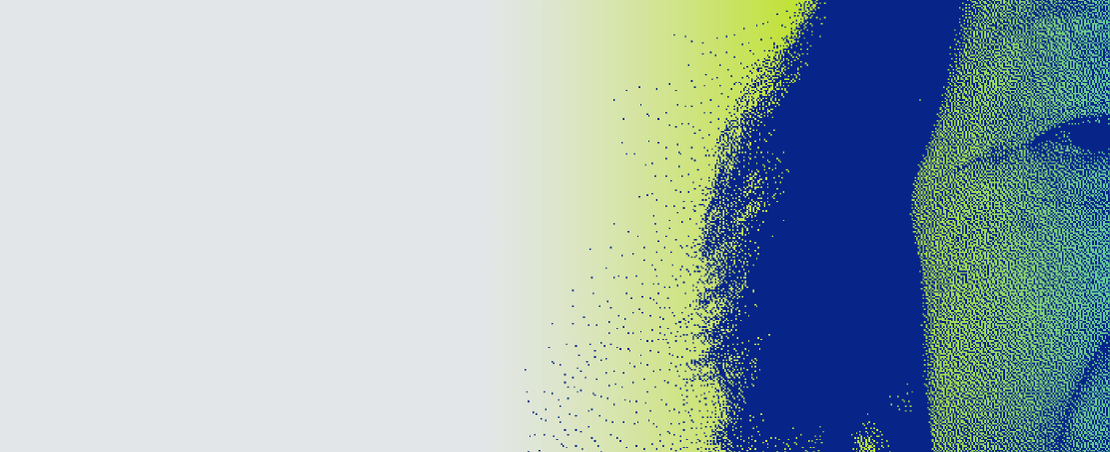
The age of foundation AI is characterised by models of large scale and high flexibility, capable of producing rich outputs. Recognising both the potential and the risks of these new models, the ODI has embarked on a programme of work on data-centric AI, designed to bring about an AI ecosystem grounded in responsible data practices. We’re exploring what policy interventions could be made to ensure these technologies are developed and deployed in ways that benefit everyone – people, communities and businesses. This is the conclusion of our five-part series exploring these policy interventions, and how they can help to positively shape the landscape.
Why is empowerment important in the context of data centric AI?
Achieving the economic and societal benefits of AI critically depends on having trust in the technology. There have been widespread calls for more participation in AI as a means to build trustworthy solutions by design rather than trying to gain that trust afterwards. Foundational models are a step change from earlier types of AI in terms of performance, risks and impacts - as such, conversations around when and how AI should be used need to leverage the expertise and opinions of a broader range of people and communities.
The last decades have shown time and time again that not empowering people to shape and participate in systems of collecting, sharing and using data will create mistrust. As we describe in our Theory of Change, there is a risk that failing to address people’s fears and legitimate concerns – such as about who has access to data and how this data might be used – will prevent us from realising the potential of data-centric technologies, including AI.
AI and data are intrinsically linked – without data there is no AI. Access to large amounts of data has become crucial for the development of AI - much of this data is created by the public and includes user-generated content scraped from the internet. Moreover, generative AI systems interact with consumers at a scale that predictive or analytical AI haven’t - every time we ask a tool like ChatGPT or Midjourney to generate content for us, we provide instructions in the form of prompts. Those prompts and the feedback we provide to the tools capture what we're interested in, what we work on, and what we plan to do. They help improve how foundational models work, so we need to make sure that the benefits of these improvements are spread equitably.
To give people some autonomy over how their prompts and preferences are used, some chatbots have introduced user controls, such as the ability to turn off ‘conversation’ history and export data out of their system. However, the failures of the notice and consent mechanism are well documented. Constant requests to consent to data collection and processing has created consent fatigue. When users do consent they are not fully informed, nor aware of what they are consenting to. A choice between opt-in/opt-out is often insufficient for genuine empowerment as it doesn’t provide the opportunity for people to shape or control systems.
Data generated through the use of AI platforms is only one source of data for foundational AI. Right now, AI companies are looking to get access to large datasets – particularly valuable is data from online communities because it is highly curated and as such better quality than most Internet content. Some companies are licensing and supplying this data to generate revenue, but have met resistance from contributors. For example, the Reddit community has engaged in blackouts and subsequent closure of subreddits over the platform selling their data to AI firms. Reddit has subsequently taken over several subreddits and signed deals with Google and OpenAI. DeviantArt had to reverse its decision to use artists’ work to train AI models by default; instead, users can now actively consent to such use. StackOverflow has gone as far as blocking users who deleted their contributions in protest over the sale of their data to OpenAI. Clearly, the withdrawal of this data can have knock-on effects to AI companies who need it.
We need to move beyond transparency and accountability to a world where people can meaningfully participate in how data is used by the government, industry and beyond. Empowering people and communities in the context of AI means enabling them to shape how algorithms and the underlying data are designed, deployed and used for societal, environmental, and economic benefit.
Genuine empowerment will take many forms and cover the entire AI lifecycle. From decisions about whether AI should be used, to labour unions for data workers, from those generating public data to protecting those ensuring its safety. This piece, therefore, should be read in conjunction with our previous interventions in data protection and labour rights, and broad access to data, which both include further recommendations on AI, data and empowerment through rights.
In the remainder of this post, we will focus on a slice of this work – how individuals and communities can be empowered to actively contribute to and shape AI models, and how data they have a stake in is used.
How can people be empowered to affect AI data?
The Ada Lovelace Institute has adapted Arnstein's ‘ladder of participation’ for data stewardship, which details the different degrees of participation, from being informed or consulted about how data is used through to empowered to make decisions about data use. In our work on participatory data, we see participation happening at different levels of the data ecosystem:
- the data level (e.g. creating or contributing to datasets in citizen science, or withdrawing consent or blocking data access)
- the organisational level (e.g. making decisions about how data is governed through data cooperatives),
- the policy layer (e.g. engage with policy makers about data use through citizen engagement).
Just as generative AI has changed how we think about data and technology, empowerment in the context of data and AI can be different. For example, a recent study suggests that direct engagement in foundation models is hard to facilitate, given the power asymmetries in their development (i.e. big companies vs small communities trying to contribute); however, engagement in domain-specific technical infrastructure and governance shows promise.
The Collective Intelligence Project have outlined the different ways that people can be engaged in AI development, corresponding to the different rungs of the ‘ladder of participation’:
- Making AI more accessible and enabling society more broadly to benefit from it, for example by bridging the digital divide with generative AI tools.
- Co-designing AI systems by facilitating engagement during development, for example Wikibench enables people and communities to design evaluation datasets to ensure it is assessed on their needs.
- Expanding the AI ecosystem through more accessible funding and data, including initiatives like Aya, which crowdsource new datasets to support underrepresented languages.
- Directly involving the public in the governance of AI, for example through data institutions like data trusts or via citizen panels.
Current policy status in the UK and elsewhere
Participation has a long history in the UK, meaning that it is well-placed to become a leader on participatory data and AI with a strong civil society sector who value it, organisations who facilitate citizen involvement, and a history of cross-sector projects looking at user rights.
Several amendments to the Data Protection and Digital Information (DPDI) Bill – which was not passed because of the 2024 election – defined ‘data communities’ in the form of intermediaries that could be assigned data subject's rights and be able to exercise them on their behalf, including negotiating access to their data for AI developers. However there has been criticism that it was 'co-designed with industry, for industry, in order to maximise the economic benefits', at the cost of the involvement of civil society and wider public benefit.
The 2023 AI Fringe: People’s Panel on AI brought together a representative group of members of the public to attend, observe and discuss key events from the Fringe. One key suggestion from the panel was a form of citizen engagement, similar to jury service, which could provide 'guidance, recommendations or judgements about AI' to industry and government.
The lead to the 2024 UK General Election has brought greater attention to public participation, following proposals by Labour, the Liberal Democrats and the Greens to explore the use of citizen assemblies to consult on significant policy issues, including AI.
Beyond the UK, the European Commission continues to drive a ’human-centric’ data agenda that cuts across its broad data policy work, discussed in prior parts of this series. The Data Governance Act seeks to enable individuals to share their data voluntarily for the benefit of society through trusted organisations adhering to EU values and principles. It calls these ‘data altruism organisations’. To which degree these efforts will be successful cannot yet be determined, but they all aim to build controlled environments under which data for AI development could be shared.
The Canadian Government ran a public consultation on AI, which was criticised for ‘not fulfilling key purposes of a consultation, such as transparency, democratic engagement and public education' and therefore falling short on citizen empowerment. In 2023, Brazil introduced new draft AI legislation which would include the ‘The right to human participation in decisions about AI systems’. In 2023, US Public AI Assembly explored public attitudes regarding risk and uses of AI across multiple domains including administrative records, health records, browser history, and facial recognition. The City of Amsterdam has used both citizen dialogues on the future of AI in Amsterdam as well as citizen council providing input into the design and use of an algorithm for a social assistance programme.
It seems policy-led participation around data and AI is not yet well developed. Where participation does exist, it is usually towards the lower end of Arnstein's ladder. Higher levels of empowerment involve sharing power to shape or contribute to decisions, which can be difficult in a government context. But there are initiatives emerging from civil society, industry and beyond that policy-makers can learn from.
Proposals from civil society, industry and other non-government actors
Beyond government action, there has been a wide range of activity from industry, the third sector and beyond. These proposals to empower people in the context of data and AI have broadly three different aims: enabling control, embedding the public decision making in AI models, and contributing data to AI models.
Enabling control
As well as long standing ecosystem of technical approaches to data empowerment, there are also some newer approaches emerging to empower people to control how data is used to train AI (sometimes referred to as ‘consent layers for AI’ or ‘preference signalling’).
These new approaches show the range of what empowerment means to different people and communities in practice. For example, some of these approaches are designed for transparency and to facilitate individuals’ contribution of data, while others support individuals in refusal. Some examples include:
- New web publishing protocols, such as ai.txt, NoML, W3C’s TDM Reservation Protocol, Adobe’s Do Not Train metadata tag.
- New technical tools, such as Nightshade, Glaze and Data Levers and other methods to limit web scraping or block crawlers.
- New transparency services like ‘Have I been trained’ and ‘Exposing AI’ that help co-generators to understand if data about them, or their content, has been used to train AI models.
- New types of data licences, such as RAILs and the Data Science Law Lab’s non-extractive licence. Te Hiku Media has developed the Kaitiakitanga Licence for 'indigenous people's retention of mana over data and other intellectual property in a Western construct'.
- New platforms and marketplaces for data/content, such as MetaLabel and UbuntuAI.
Embedding the public decision making in AI models
Most work on enabling the public to contribute towards the development of AI models in the area of AI alignment.
OpenAI has run a grant programme on ‘democratic inputs to AI’, which led to them forming a ‘Collective Alignment’ team, consisting of researchers and engineers. This team will 'implement a system for collecting and encoding public input on model behaviour into our systems'.
In October 2023, Anthropic published the results of its own alignment work with Collective Intelligence and Polis to 'curate an AI constitution' based on the opinions of 1000 Americans. The final constitution focused more on objectivity, impartiality, and accessibility and when used to train an AI model, it was ‘slightly less biased and equally as capable as the standard Anthropic model'. Recursive Public is an experiment to identify areas of consensus and disagreement among the international AI community, policymakers and the general public.
Pilots of WeBuildAI, a collective participatory framework, found that using the framework led to improvements in the perceived fairness of decision making, public awareness of the algorithmic technology in use, as well as the organisation’s awareness of the algorithm’s impact.
Contributing data to AI models
Another way that we have seen individuals and communities engaged is through contributions to datasets. These contributions can take many forms and serve many purposes, for instance, reflecting communities' lived experiences, helping scientists and policy makers, or collectively deciding on the scope of new data analyses. An example is citizen science - projects such as FoldIt or any of the Zooniverse projects are designed from the outset to create better datasets for AI training, for instance by annotating images, audio or video content that algorithms find hard to process.
While participation is not widely spread, existing success stories show the way forward.
Communities around platforms like Wikipedia have hundreds of thousands of contributors from around the world. Data from these platforms is available under open licences for many purposes, including AI development. Solutions like Wikibench allow the community to participate directly in shaping the data that goes into AI models that Wikipedia uses, for instance, to identify malicious editors.
Projects such as BLOOM and BigCode are exploring collaborative methods for data and AI development. There are new participatory methods of data collection and model training specifically focused on language data, such as CommonVoice, Aya and FLAIR. While Karya runs a data annotation platform which pays a fair wage to its contributors. These initiatives seek to create datasets of underrepresented languages, to ultimately empower communities around the world to realise the value of AI.
Steps to take
Our recently launched Policy Manifesto, which received UK cross-party support, argued for 'empowering people and communities to help shape how data is used for society, the environment, the economy and the public good'.
We expect that the new UK government will continue to work on plans to empower research, innovation and industry with data for AI, and do so in a responsible way. We recommend that the incoming UK government:
- Strengthens individual controls over data, building on the success of data portability in the banking sector, the Government must explore regulatory changes which support people to have more control over data. This could include building on the UK GDPR to provide more individualised control over data in the era of AI. This regulation must engage with the characteristics of how data is used for AI to ensure it functions in the interests of people and communities as well as industry, and should go beyond training data to include prompts and various forms of feedback.
- Meaningfully utilises participatory methods to involve the public in shaping the future of data and AI in the UK, especially when it comes to the terms of use of critical national data assets and other sensitive data. Involving people through dialogue and engagement to decide how AI is regulated and used by public bodies in the UK is a good place to start. The Government can learn from current examples of citizen assemblies and forums to explore the viability of genuine delegation and control over decision making.
- Supports the thriving ecosystem of participation in the UK, and works with them to improve current practice. There is a lot of expertise and innovation happening in the UK. This landscape can support efforts to improve public engagement for new data and AI regulation, including by contributing to decisions about AI as well as generating new datasets needed for AI innovation. This will also require understanding where and how engagement methods in AI lifecycles are most effective, which should be investigated through consistent funding for participatory initiatives and recognised participatory research.
At the ODI, we’re keen to provide insights and resources to policymakers working towards creating fair and inclusive data licensing models and governance frameworks. This blog is part of a series of policy interventions, which you can explore here.
If we’ve missed any examples of data empowerment or you’d like to chat with us about our work on data-centric AI please get in touch at [email protected].