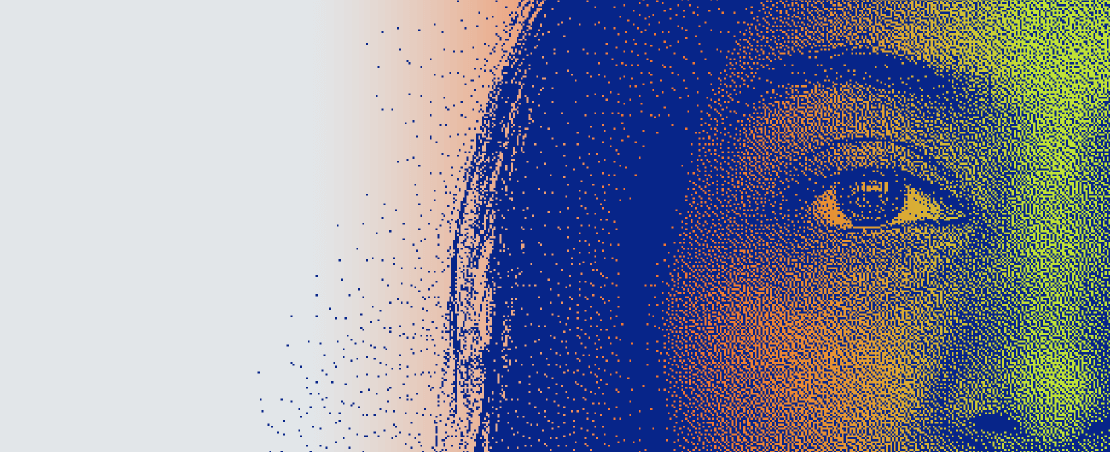
The age of foundation AI is characterised by models of large scale and high flexibility, capable of producing rich outputs. Recognising both the potential and the risks of these new models, the ODI has embarked on a programme of work on data-centric AI, designed to bring about an AI ecosystem grounded in responsible data practices. We’re exploring what policy interventions could be made to ensure these technologies are developed and deployed in ways that benefit everyone – people, communities and businesses. This is the fourth in a series of five pieces exploring these interventions.
Where does the data used to train foundation AI models come from?
Data is the foundation of AI systems. Across the AI lifecycle, data is collected, processed, curated, aggregated, and subsequently used in the models. Data is also essential for testing and benchmarking a model's performance, as well as for input once a model is in use.
Foundation AI is trained using a rich variety of types of data (eg tabular, images, voice) from varying sources (scraped from across the web, or from the service people interact with). The sources of data for AI are diverse, particularly for foundation models that require vast amounts. These sources can include data collected from the web, enterprise data, or a combination of both, and include vast amounts of text and image data from websites, collections of books, statistics, maps and images. Quality is essential, as models are as good as the datasets.
We're focusing here on foundation models, but much of this discussion and our recommended course of action will also apply to narrower, predictive or analytical AI. Any large AI scale datasets must be constructed in ways that respect people's rights. We address how the incoming UK Government should protect Intellectual Property and data protection and labour rights.
Why is broad access to data important in the context of foundation AI?
Traditionally, machine learning relied on manually crafted datasets, which are often timely to create or challenging to source. As the scale and demand for data have grown, there has been a shift toward collecting vast amounts of data from the web and relying more on crowdworkers for fine-tuning and prompting. To the current age of foundation models – web-scraped datasets such as CommonCrawl and LAION alongside access to public platform data from Wikipedia, Reddit and StackOverflow have been central. Open and broad access to data that can be used to AI is important to ensure a diverse, competitive ecosystem of AI developers. Andrew Ng emphasised that protecting open source is vital for the AI ecosystem to allow innovative startups to enter the market.
However, in the face of foundation AI, there are a growing number of barriers to broad and open access to public data.
Access to large-scale datasets are becoming increasingly expensive, with costs expected to explode as demand continues to increase. This is partly because the usefulness of datasets is more to do with quality, rather than size and hence heavily reliant on expert human-curation. Some web publishers are also starting to restrict access to data, with nearly 14% of the most popular websites blocking Common Crawl’s bot – often to protect intellectual property, and potentially in order to strike lucrative private deals directly with AI companies. This closing of data favours large organisations who already have stockpiles of data, have the financial means to go to court and can enter into bilateral agreements to licence data. These strategies are inaccessible to small competitors and academics. As such, the next wave of LLMs risks being built by private companies on closed datasets. Also, monitoring the performance of foundation models remains challenging due to the shortage of publicly accessible data and benchmarks.
There are significant concerns that the era of open-access datasets might be ending and we are approaching a so-called ‘data winter’.If this ‘data winter’ comes, and open access to data declineS, Creative Commons has expressed concern that there could be ‘a net loss for the commons... overly limiting to expression’. For instance, based on current trends in access to social media platform data, closing down public access to data could force those developing AI models to licence data at a high cost directly from data holders or purchase it from expensive data brokers.
As well as affecting AI development, the closing of historically open datasets has further knock-on effects to researching the commons. In many cases, there is no alternative for some of these datasets – such as CommonCrawl and Wikipedia – meaning further limits on research on uses of large public data: tackling web censorship, history of science research or public and political advocacy.
Open source organisations are vital in supporting the ecosystem to resist the closing of data. For example, Clement Delangue, CEO of Hugging Face, testified before US Congress on the need for ‘ethical openness’ in AI development which would allow researchers beyond a few large tech companies to access the technology. Reuse of data is vital to preserving broadly-accessed datasets, as ‘making a data set available for further research and development activity may help keep it up to date as other researchers/developers are likely to contribute with new data’.
Current policy status in the UK and elsewhere
In September 2023, the UK’s Competition & Markets Authority published a set of principles for foundation AI models, including calling out a necessity for 'access to data, compute, expertise and capital without undue restriction'.
The UK Government has a track record in investing in data infrastructure that enables wide use and sharing of data, including that it holds itself. The UK Data Service, for example, is a national research infrastructure that provides trusted access and training to use a large collection of economic, population and social research data – funded by the Economic and Social Research Council. There are a number of other investments in building data infrastructure made by UKRI and the other UK research councils, alongside Smart Data Research UK.
In the health sector, Health Data Research UK drives a number of initiatives to increase the sharing and use of data. INSIGHT, for example, was supported by HDR UK, and is now the world’s largest ophthalmic database of more than 25m retinal images, and is driving innovation using AI to diagnose degenerative disease. In a similar space, the nine EPSRC-funded AI Hubs for Real Data demonstrate the importance of public funding to data-centric AI infrastructure.
Launched in June 2024, the Labour Party’s Manifesto included a proposal for a National Data Library to centralise existing research programmes and support the development of the artificial intelligence sector. It is based on UK under-productivity – Britain has the third largest data pool, but it is growing at nearly half the speed of Germany and France.
The European Commission EC) has long driven access to public data through its Public Sector Information Directive (now called the Open Data Directive). It has also led on identifying high-value datasets that governments should focus on enabling access to, and maintains an official portal for European data. The EC has also created a rich ecosystem of initiatives to stimulate the sharing of private sector data, for example the Common European Data Spaces, Data Spaces Support Centre and the European Data Innovation Board. The EC is currently consulting on competition and generative AI, which includes an interest in the availability of data. The EU’s proposed Artificial Intelligence Act (AIA) is slated to bring more clarity to the use of text data for AI, while the Digital Services Act (DSA) would bring researchers increased access to social media data.
In the US, the FTC has expressed concern that ‘companies’ control over data may create barriers to entry or expansion that prevent fair competition from fully flourishing'. The US Department of Commerce has launched a new AI and Open Government Data Assets Working Group, which will modernise public data to be AI-ready. The French AI commission has recommended the creation of an International Fund for Public Interest AI with an annual budget of €500m to finance open and public interest AI. Presumably this would include provisions for making data available.
Proposals from civil society, industry and other non-government actors
A number of initiatives have been started by AI firms and developer communities to build new, AI-ready datasets.In March 2024, researchers launched Common Corpus, claiming it to be 'the largest available AI dataset for LLMs composed purely of public domain content'. Common Voice is a publicly available voice dataset built by thousands of volunteer contributors on the belief that “that large, publicly available voice datasets will foster innovation and healthy commercial competition in machine-learning based speech technology”. The Lacuna Fund has already supported the construction of datasets for agriculture and natural language processing, and has recently announced a new wave of projects related to climate change. The MLCommons Datasets Working Group creates and hosts public datasets that are “large, actively maintained, and permissively licensed - especially for commercial use”.
Hugging Face now hosts over 80,000 datasets and includes restricted access to ‘Gated Datasets’. It has been described alongside Kaggle and OpenML as an example of the new wave of ‘community data hubs’ and ‘standardised data loading infrastructure’ being built to serve the AI industry. Mechanisms such as synthetic data – data created automatically, using AI and other tools– can also be used when the original data is not representative and needs to be rebalanced, or when it's sensitive and can't be shared, or when it's too costly to collect.
The AI Now Institute and other European think tanks have published an open letter to the European Commission, arguing that 'firms with access to proprietary and curated datasets enjoy a competitive edge', causing concentration and limiting competition in the AI market.
Others have proposed new ways to open up access to data held by private firms. Saffron Huang and Divya Siddarth suggest that '[AI] companies could create, as a norm or a rule, gold standard datasets usable by other entities'. The Ada Lovelace Institute has discussed the potential to 'mandate research access to Big Tech data stores, to encourage a more diverse AI development ecosystem'. OpenFuture has developed a blueprint for Public Data Commons, which would act as trusted intermediaries to make private sector data available for public interest sharing and enable public value to be generated. Several open data initiatives in the scientific field demonstrate the impact of open repositories which follow FAIR principles with structured catalogues of data and standardised data formats.
There are also new proposals around governments’ role as a provider of data for AI. Stability AI has argued for nation states to have its own national image generator, one that reflects national values, with datasets provided by the government and public institutions. The Bennett Institute has proposed a ‘national data trust’ where 'data from national sources, such as the BBC and the British Library, would be entrusted. The Tony Blair Institute has been talking about data trusts too, as a form of new institution to increase access to NHS data for research and innovation.
Steps to take
In order to protect the broad access to data for AI research and innovation, we recommend that the incoming UK Government:
- Support the creation and improvement of AI-scale datasets. As outlined in our policy manifesto, we advocate for improved data infrastructure for AI and the preparation of AI-ready data. This includes government actions to create and regulate high-quality datasets, ensuring that these datasets are accessible, reliable, and usable, and published according to high and agreed-upon standards. The UK government should support and protect data infrastructure to ensure financial sustainability, with funding prioritised for organisations and communities that create and evaluate well-curated datasets while exploring ways to prevent previously open datasets from becoming restricted. Additionally, we call for robust infrastructure to enable AI systems to use data responsibly, including mechanisms for assurance and quality assessment.
- Explore new approaches to opening up access to public sector data. The UK’s vast body of open, shared and closed data needs to be better capitalised on, using FAIR (findability, accessibility, interoperability, and reusability) principles to shape broad access to high-value data. Data institutions to responsibly steward this public data should also be sustainably supported through funding and infrastructure.
- Open up access to private sector data. The UK government should explore cross-sector approaches to opening up access to private sector data for AI – building on the progress of initiatives such as SMART data to capitalise on the UK tech sectors potential. The government should also be supporting research into techno-legal approaches, such as revisiting licensing as a core part of the foundation AI research agenda. Further advancements in synthetic data, if used responsibly, can fill gaps where data cannot typically be accessed.
At the ODI, we’re keen to provide insights and resources to policymakers working towards creating a fair intellectual property regime in response to foundation AI. We will publish our final proposed intervention focused on empowering individuals in data and AI shortly.