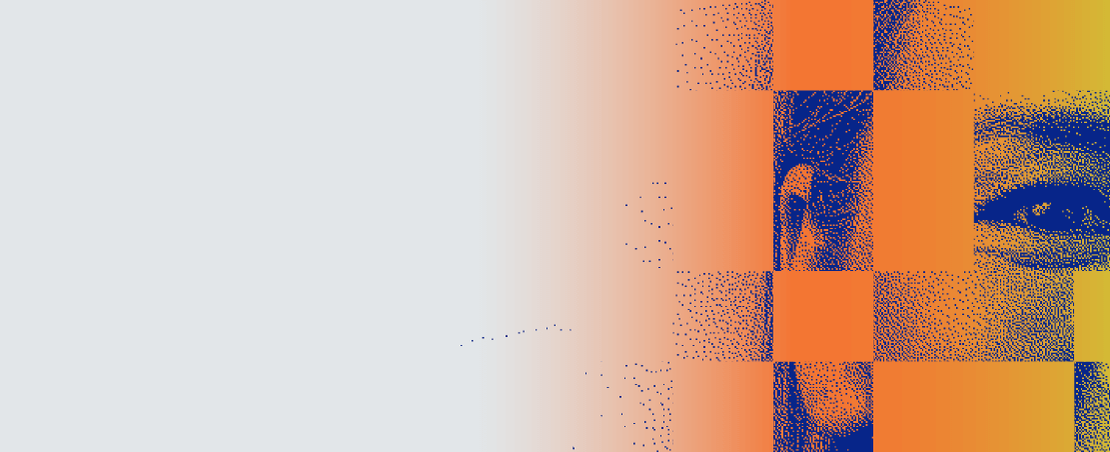
While AI may often seem to exist abstractly in cyberspace, it is also driving major supply chains of both materials and labour. In this report, we focus on the landscape of work and workers behind AI data, which is often invisible to decision makers and the public. The invisibility of AI data work is a major challenge, since it limits the emergence of debate and setting of best practices that support model developers, researchers, innovators, policymakers and others to play their role in ensuring responsible and effective data work across these AI supply chains.
AI data work enhances and contextualises the datasets used in machine learning. For example, it can be used to moderate whether data scraped from the internet contains harmful images or language, or to prepare datasets of images that accurately represent the presence or absence of a certain disease.
Data work has been the subject of much concern due to significant issues around labour rights. In this report, we take a broader view of data work, outlining its role in AI supply chains through a ‘taxonomy’ of data work, and further exploring how its use is evolving within the changing AI landscape. We particularly explore:
- The dependency of the nature of data work required on the application domain, creating application-specific trade-offs and responsibilities for decision-makers;
- The emergence of new tools to support data work, using AI innovations to support data workers, and to ensure data work can keep pace with evolving expectations on AI systems;
- Emerging standards being set for improving transparency about data work, among widespread lack of transparency.
This research has been conducted as part of our Data-Centric AI programme, and was inspired by our Humans in the Loop panel session at the Data Empowerment Fund event in November 2024. We hope this helps readers to understand the importance and evolution of data work in the rapidly developing landscape of AI. With a relative absence of best practices and standard setting in this space, we want to motivate the further development of practical tools, benchmarks and guidance for labelling data in a responsible way - and future work outlining the roles in achieving this across the AI supply chain for developers, researchers, civil society, AI data suppliers, innovators providing data work services, multilaterals, the media and beyond.