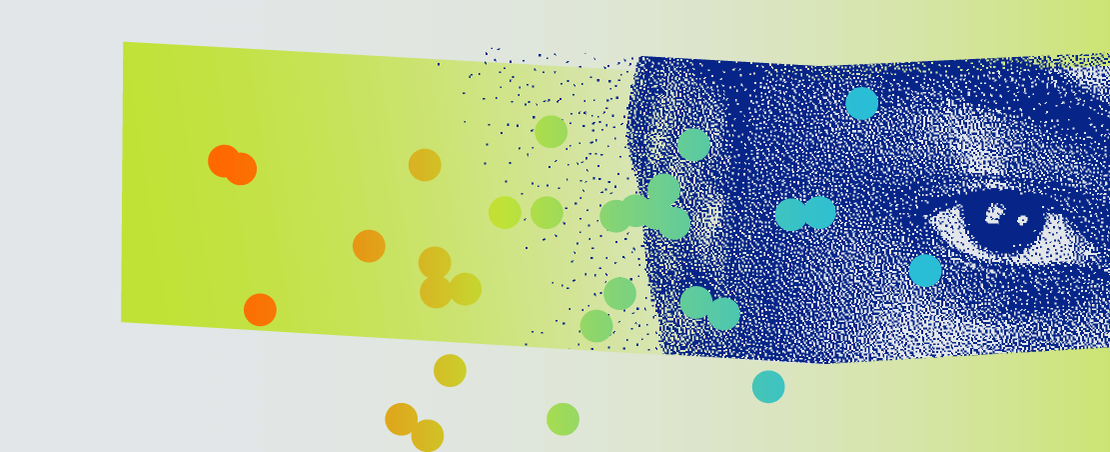
‘Without data, there is no AI’ has become a mantra for the ODI’s data-centric AI programme. As we embark on the 2nd year of the programme, we’re updating our description of the role of data in modern AI systems, based on the work we've done, as well as some general trends we've observed across the ecosystem.
Data's pivotal role in the AI lifecycle
Data is the cornerstone of all AI systems.
In October 2024, we published a taxonomy of the data involved in developing, using and monitoring AI. It is primarily designed to reflect how frontier models use data, but much of it also applies to narrower or predictive models too.
The taxonomy shows the necessity of high-quality data at every step of the AI lifecycle. It describes the different types and sources of data needed for:
- Developing AI systems, including existing data, training data, reference data, fine-tuning data, testing and validation data, benchmarks, synthetic data, and data about the data used to develop models.
- Deploying AI systems, including model weights, local data, prompts, and outputs from models.
- Monitoring AI systems, including data about models, data about model usage and performance in context, registers of model deployments, and data about the AI ecosystem.
The complexity of the data for AI ecosystem continues to grow. Spawning’s Do Not Train Registry is an example of a new dataset that’s emerged over this past year. The registry logs the data-use preferences for over 1.5bn individual works, and can thus be used by AI developers to ensure they respect the training preferences of the people who have generated the data.
In other ways, the data ecosystem still has a way to go. For example, while we’ve highlighted the need for governments to publish data about where AI models have been deployed in public services, the UK Government has only published basic details of nine algorithmic systems. Our research has shown how open government datasets are being fully utilised to support citizen queries, including through chatbots like ChatGPT.
We’ve now published an updated taxonomy. Over the next year, we hope to see the taxonomy used by more organisations developing, using and governing AI systems. We believe it will aid in making more informed and intentional decisions about how these different data types are collected, used, stewarded, and shared. As we’ve recently argued, a better understanding of the state of the data for AI ecosystem is needed to design more ambitious and targeted remedies.
Is the availability of training data reducing?
Advances in AI over the past five years have been characterised by the mass scraping of data from the web to train new models.
It has been likened to the Napster era of music, where technological advancement and new consumer tools came at the cost of intellectual property infringement and legal disputes. Getty Images, for example, is suing Stability AI for allegedly training its AI model on more than 12 million of its photos without permission or compensation.
In response to this, we’ve seen:
- Web publishers stopping their content being used for model training. This involves websites updating their terms of service, or using protocols such as ai.txt, NoML and TDM Reservation Protocol. A recent analysis of the popular C4 training dataset has found that “a rapid crescendo of data restrictions from web sources.. [has rendered] 28%+ of the most actively maintained, critical sources in C4, fully restricted from use”.
- Owners of large corpuses of data agreeing licensing deals with AI firms. Many holders of large, valuable sources of AI training data, such as news outlets, music labels and movie studios, have now come to agreements with AI companies. Associated Press has signed a deal with OpenAI for access to its archive of news stories; Google’s deal with Reddit for access to its forum data is said to be worth $60m per year.
- New data marketplaces emerging to unite supply and demand for training data. This includes Human Native AI, Dataset Providers Alliance, Scale.ai, and Valyu. Some of these marketplaces describe themselves as enabling the long tail of smaller data/rights holders ‘to monetise their content’.
- Rifts among communities who intended for their works to be widely consumed. In 2023, many of Reddit’s biggest forums ‘went dark’ in protest over the platform’s plans to enable AI developers to access the mass of forum conversations they’d played a vital role in creating. The Wikipedia community is divided on whether large models should be allowed to train on Wikipedia content.
Together, these developments could reduce the availability of web data to train foundation models over time. The Open Source Initiative has said that an AI ecosystem overly reliant on licensing deals may end up less diverse and competitive, as small firms and academics do not have the financial means to enter into bilateral agreements to licence data. Creative Commons has expressed concern that there could be ‘a net loss for the commons’ and some have questioned whether we’re about to enter a ‘data winter’.
To maintain broad access to the web’s abundance of data, we must ensure there’s a fair exchange between producers of data and those using it to train foundational and frontier models. Over this past year, we’ve proposed how people should be given more of a say in the use of data for AI, and argued for governments to update our intellectual property regime to reward human creativity while also driving new research and innovation.
Moving forward
Since publishing our original case for ‘without data, there is no AI’, we’ve seen the AI data ecosystem evolve rapidly.
Over the next year, we expect to see:
- Deployment of foundation models to address business use cases, using enterprise data. We’re shifting from a major period of foundation model development, characterised by training on data scraped from the web, to the application of these models using businesses’ own data. Cohere, for example, has described its new focus on delivering ‘private deployment’ and ‘deep customization’ for its clients. We expect to see more scrutiny of the terms of data access between AI firms and the enterprises using their models, with a focus on topics like memorisation risk and whether enterprise data should be used to improve the underlying model for other customers.
- Greater recognition of the value of access to accurate, frequently-updated and factual data. Architectures such as retrieval augmented generation are now used by AI systems to retrieve up-to-date information in response to user queries (as opposed to generating the response from the trained model alone). Wikipedia has long acted as the “factual netting that holds the whole digital world together”, and it’ll play a similar role across AI systems going forward. Other important reference data includes: predefined thesauri (such as Wordnet); mathematical proofs (such as Lean); and knowledge graphs (such as Wikidata). We must maintain broad access to this data.
- Use of synthetic training data. Synthetic data is data that is created algorithmically, often using models or simulations, rather than originating from real-world sources. According to recent analysis of the data used to train a sample of large foundation models, the use of synthetic data has risen from around 10 million tokens to more than 100 billion tokens between 2017 and 2018. However, there’s concern in some parts of the AI community about model collapse, where a model gradually degrades through ingesting too much synthetic data, especially data the model may have generated itself.
- More purpose-built training datasets. To date, much of the data scraped from the web to train foundation models - artworks, music recordings, forum posts, etc - was originally produced for human consumption. One way for the AI community to bypass some of the associated issues (such as copyright claims and the consumption of personal data) is to build new datasets specifically for model training. There’s already important work underway to do this (such as that led by MLCommons), as well as examples of large, ‘clean’ datasets (such as Public Domain 12M) and tooling to help build AI-ready data (such as Argilla). We believe this work can and should be a community effort; incorporating diverse perspectives into these processes will help to assess data’s biases, limitations and other areas for improvement.
- Advances in trustworthy data practices, particularly on AI data transparency. As we’ve pointed out before, most leading AI firms have refused to disclose details about the data they’ve used to train their models. We’re hopeful that the next wave of AI development and use will involve drastically improved transparency about the composition of training data. We’re seeing strong progress being made by the developer community, including via efforts such as Dataset Cards, Dataset Nutrition Labels, Data Provenance Initiative and Croissant, as well as increasingly granular mandatory filing templates from governments. We hope our own work with the AI Data Transparency Index will help to deliver meaningful and user-centric data transparency across the ecosystem.
If you’d like to work with our data-centric AI programme, get in touch.