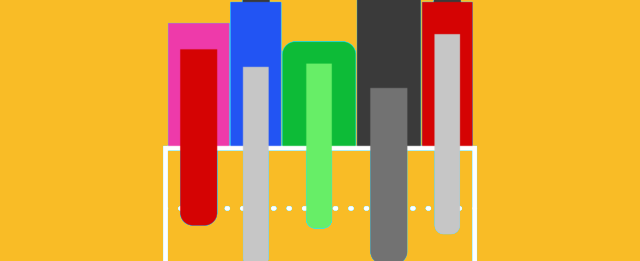
This is the second worknote on the research stream of the ODI’s ongoing Luminate-funded Covid-19 project. You can read our first worknote from the project here.
Intermediaries and private sector data
We’re researching how data intermediaries can help share more private sector data in response to Covid-19. Learning by doing, we’re talking to potential users of private sector data and finding businesses that can provide the data the users need.
We also know we can only do so much ourselves. We’ll create advice on what we’ve learned along the way and how others can help this flow of data between providers and users. We believe the Covid-19 response will need many types of data intermediaries, both large and small.
In our earlier research, we found that people were sharing data related to Covid-19 by creating lists or repositories of data. Some examples we found were:
- AWS Data Exchange data related to COVID-19
- Coronavirus Tech Handbook’s page on datasets
- Govlab’s list of data collaboratives in response to COVID-19
- EU Open Data Portal’s group for COVID-19 data
- Github’s COVID-19 Public Repository Data
These lists help data users by saving them time and effort in finding the data they need and they help providers by giving the data they offer a larger audience.
However, these lists are just one of the many ways people can help bring data from providers to users. Intermediaries can offer services such as:
- Lists of contacts for people who would share or use data
- Collections of data from providers and giving access to specific users
- Events where providers can meet with potential data users
- Lists detailing where datasets can be found (as seen above), from simple shared documents to large data portals
- Tools which combine and repurpose providers’ data for users, such as analytical insights or dashboards
- Finding suitable users for data providers or vice versa, performing the role of a “data matchmaker”
Data intermediaries can be an informal, even temporary, group of people offering a helping hand or they can be bigger, longer-lasting organisations with legal status and financial resources.
There’s particularly a need for more work in connecting those from the private sector organisations to potential users. Many companies want to help the fight against Covid-19 but lack connections to people who would use data they hold such as researchers, governments or media. Conversely, these users often lack the contacts or knowledge about what private sector data is out there or potentially available.
The importance of quality intermediaries in the flow of data is often overlooked. But if they work well they can bring together disparate groups and greatly help in getting data where it needs to be.
If you are from the private sector and want to share data that may help the Covid-19 response or would potentially use private sector data, then please get in touch.
Mobility
Are you using mobility data in response to the Covid-19 pandemic? Please fill out our short survey.
We are continuing to look into mobility data and how it is being used in response to the ongoing Covid-19 pandemic. Since the first work note, we have published a short blog post which detailed what mobility data is being published and how it is being used. To build on this work, we are constructing a number of user personas.
User personas are fictional representations of different users of a service, they can be used whenever somebody wants to design a service. We have chosen to use this technique in order to provide a framework to analyse the usefulness of different types of mobility data. For example, the Citymapper Index may be very useful for a journalist who just wants to provide an immediate snapshot of how many people are travelling in a major city. But how useful is this for somebody working in a local authority? Likely, not very – they need much richer data on who is moving, where they are moving to and from, and historic data so that it can be compared to an equivalent point in a pre-pandemic time. Different users have different needs, and we will present this complexity through simple personas.
These personas should help answer questions such as: who is the user of mobility data? What problem are they trying to overcome with the use of mobility data? What type of data do they need? What are their barriers to using this data?
So far, we have personas on the following potential users of mobility data. Personas are not designed to be exhaustive or totally representative of individual users, but instead can be used as talking points.
[table id=25 /]
If you fall into any of these categories then please get in touch with us. We want to speak to as many of you as possible. Similarly, if you are a (potential) user of mobility data and you don’t think you are represented here, then tell us!
Models
In the light of global governmental responses to Covid-19, computer models have been somewhat thrust into the spotlight. But what even is a computational model? How do they work? Should we trust them? We are working on an explainer to discuss these questions.
Although computer models each share their fundamental structure – being composed of code, data and documentation, their uses are enormously diverse. So too is the way they function, and their role in decision making. As such, the answer to the question ‘what even is a computational model?’ becomes rapidly divergent as you delve into more detail, requiring answers to questions like ‘what is a mathematical model?’, ‘what is machine learning?’ or ‘what does stochastic mean?’.
To fit in the kind of detail needed to give a good answer to the question, but also to keep things concise and manageable, we have chosen to split the explainer into two pieces.
The first will be introductory, answering the question ‘what is a model?’ before moving on to maths, code and computer models. It’s pitched at someone who has absolutely zero background knowledge and may be interested or concerned due to hearing about models so much in the media.
The second part is aimed more at people who do have some familiarity with models, perhaps because they work in an organisation that uses them, or they make decisions informed by models but they themselves aren’t the ones creating and maintaining them. It will be looking more at explaining types of models, interpreting models, and will delve into the importance of transparency and openness in modelling, to build trust and to increase modelling knowledge and skills.