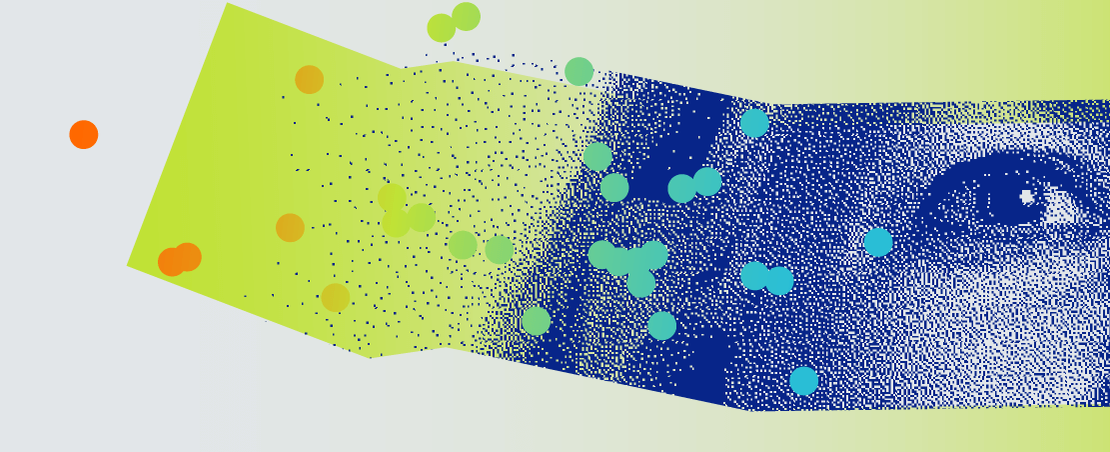
Last month, the ODI research team presented two sessions at the Association for the Advancement of Artificial Intelligence (AAAI) conference, a top academic conference for AI, in Philadelphia. We were excited to deliver the half-day tutorial and full-day workshop in collaboration with various organisations, including MLCommons, Google, MIT, RAi UK, Hugging Face, Responsible AI, and King’s College London.
The conference was an excellent opportunity to bring together experts at the cutting edge of two issues we care about - AI data transparency and data for AI safety. We developed a more decisive view of the current landscape, mainly what needs to happen next in these two crucial practice areas. AAAI is vast (with the 2024 conference in Vancouver attracting 5193 attendees), meaning the session participants contributed insights from various perspectives. This blog summarises our sessions and the key insights we gained from coalescing current researchers on these topics.
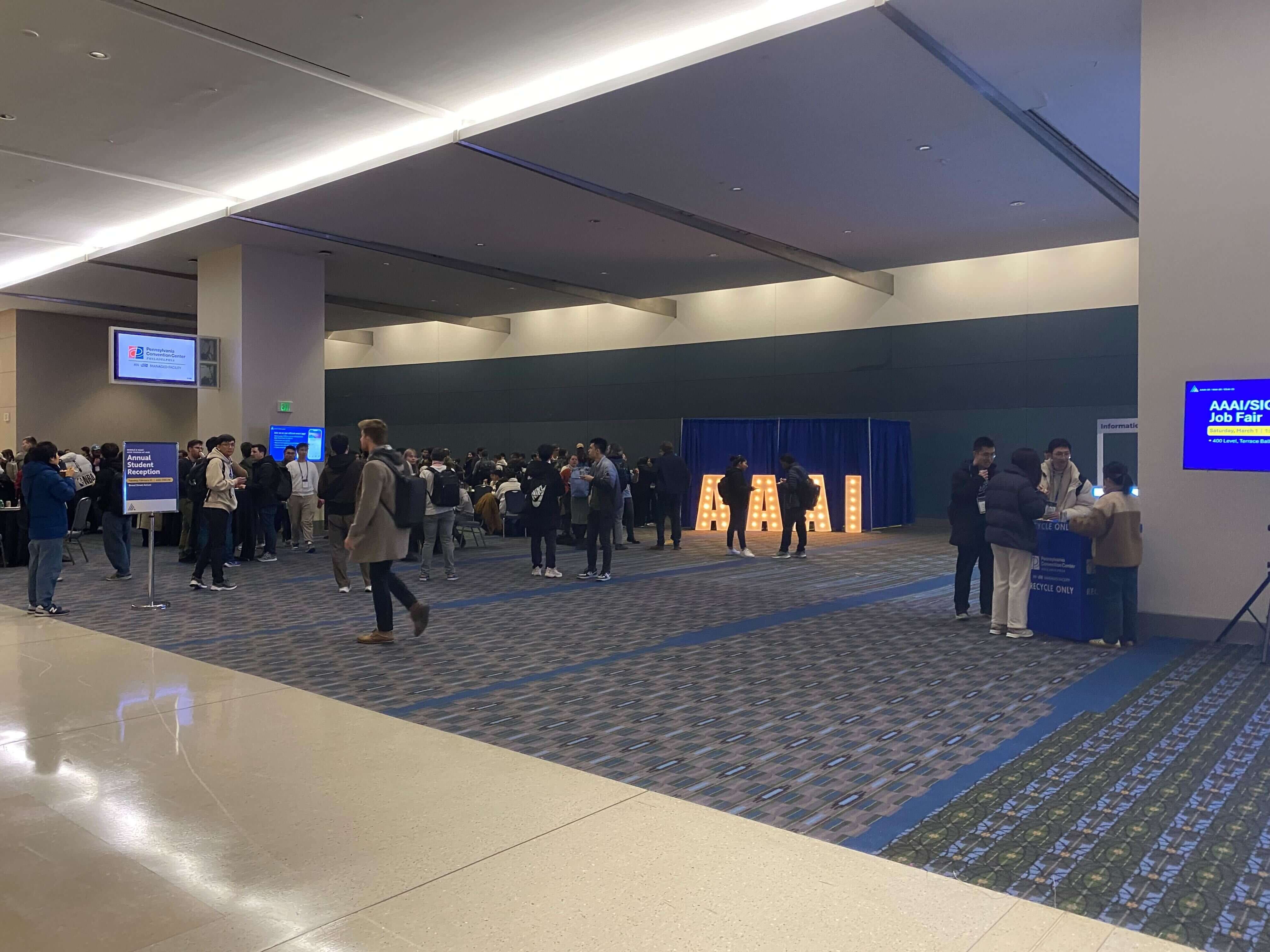
Steps towards widespread and impactful AI data transparency
Our half-day tutorial, “AI Data Transparency: The Past, the Present, and Beyond“ took place on 26 February. Presenters from IBM (Dr David Piorkowski), MIT (Shayne Longpre), Google (Dr Omar Benjelloun), Hugging Face (Dr Lucie-Aimée Kaffee), as well as King’s College London and the ODI (Professor Elena Simperl and Sophia Worth) shared their recent cutting-edge research and practice, forming an overview of the evolving landscape.
The first section addressed the current state of AI data transparency - because it is difficult to identify the main areas in which transparency is required for accountability and change without understanding where we are now. This section included a presentation by Shayne Longpre from MIT on the Foundation Model Transparency Index (FMTI). The FMTI demonstrates that AI transparency is still mostly lacking, with transparency about data among the worst-performing elements. We shared an exploratory ODI study that corroborated the FMTI, exploring data transparency within systems flagged in the AI Incident Database and highlighting the diversity of challenges accessing data transparency information beyond key foundation models. Shayne further outlined the crucial value of ‘creating transparency’ through auditing AI datasets to enable a clearer picture and better tracking of datasets across the AI landscape in work on the Data Provenance Initiative.
We next outlined emerging regulations on AI data transparency, particularly under the EU’s AI Act, which offers some far more stringent requirements (including the since-published most recent version of the Act’s code of practice), which will require significant change from many organisations. This has the potential to significantly shift the dial on data transparency in the near term, both in the EU and beyond.
In the third section, we presented our findings from the AI Data Transparency Index, which offers an approach to assess how far model providers are generating meaningful and user-centric transparency, arguing for more work to develop minimum standards centred on user needs. This laid the groundwork for an introduction to the Factsheets methodology by Dr. David Piorkowski, one of its creators at IBM. This shows in depth how organisations can assess specific client and stakeholders’ transparency needs and work to address these on the ground.
Finally, we discussed the importance of building the infrastructure to support better data transparency with a hands-on demonstration of the Croissant standard by Dr. Omar Benjelloun at Google. A key objective of this standard is to make transparency information machine-readable so that it can be collated and searched.
In all, we explored data transparency as a foundational part of building accountability and helping developers, deployers, and users to play their part in ensuring AI is safe and responsible - not as an end in itself. It seems helpful to differentiate two key elements to consider: figuring out how to motivate transparency in feasible and effective ways (the infrastructure, policies, creating transparency, etc.) and ensuring that this information addresses the crucial needs it is designed to address.
Exploring Datasets and Evaluators of AI Safety
We co-developed and co-hosted the Workshop on Datasets and Evaluators for AI Safety on 3 March 2025 alongside King's College London and RAi UK. The workshop convened leading academic, industry, and policy experts to tackle AI safety challenges, specifically focusing on enhancing datasets, benchmarks, and evaluation methods.
In an invited talk, Dr Peter Mattson from MLCommons first highlighted the importance of developing reliable AI ecosystems, showcasing initiatives such as MLCommons’ Croissant metadata format and novel benchmarking efforts. Through presentations from leading experts Dr Lora Aroyo and Professor Gopal Ramchurn, we discussed the critical need for rigorous, real-world applicable benchmarks and evaluation methodologies. Later, Professor Virginia Dignum, in another invited talk, emphasised the importance of balancing immediate ethical concerns and long-term safety issues through structured, interdisciplinary governance strategies.
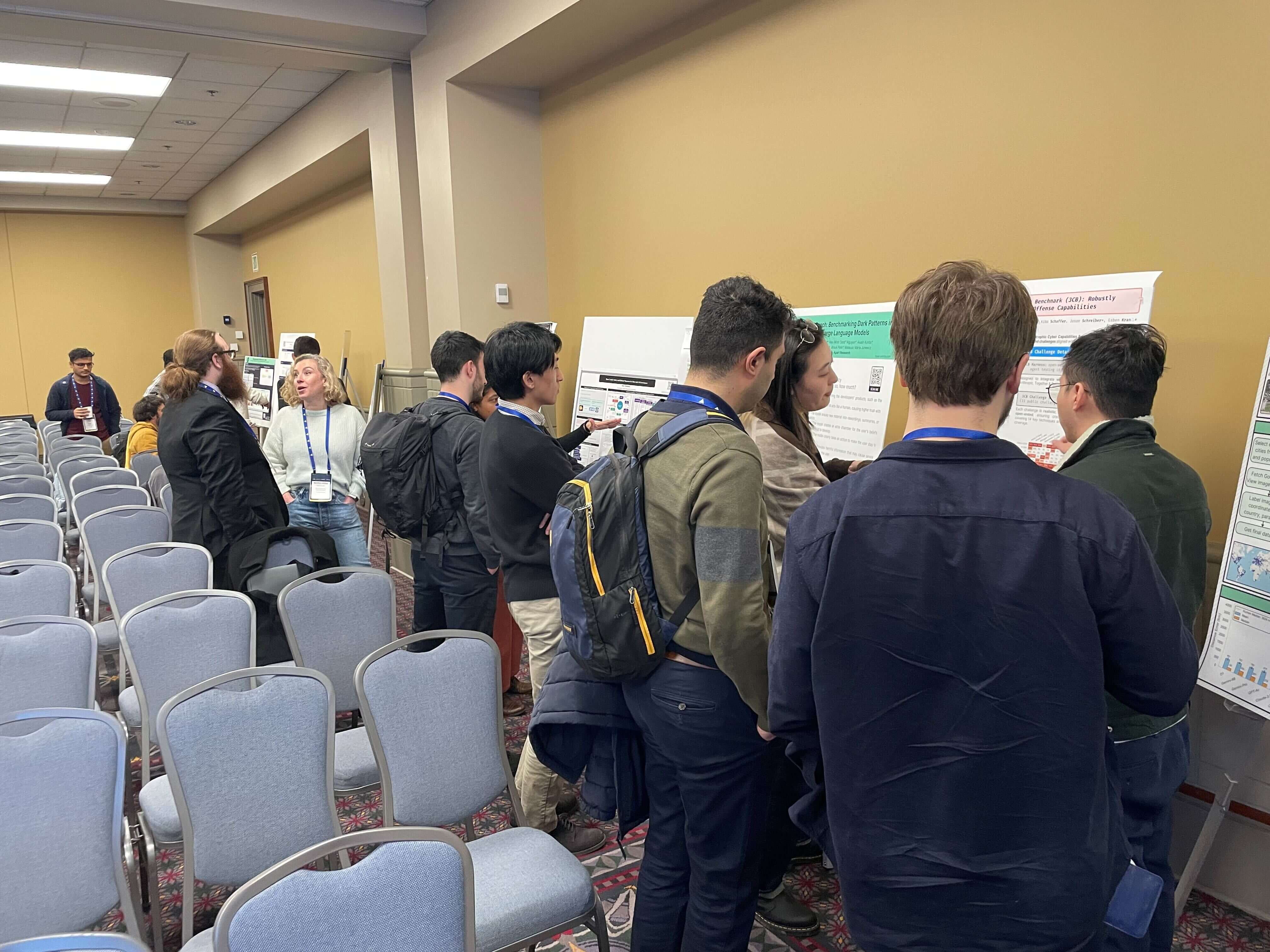
Later, two panels featuring speakers from industry and academia (including Dr Lora Aroyo, Ankit Jain, Natan Vidra, Ken Fricklas, Dr Marko Grobelnik, Dr Angelo Dalli, Rajat Ghosh, Prof. Gopal Ramchurn, Srija Chakraborty, and Dr Sean McGregor) highlighted critical gaps in evaluating the safety of evolving multimodal and agentic AI systems that present challenges beyond current frameworks. Specific issues raised included the risks of AI systems becoming increasingly opaque, vulnerable to adversarial misuse, and complex to hold accountable due to limited interpretability. Proposed solutions focused on developing evaluators capable of handling complex AI workflows, standardising personalised safety filters to mitigate targeted harms, and mandating transparent citation practices to enhance user trust.
Our next steps include collaborating on the development of improved evaluators and datasets, potentially aligned with MLCommons standards. Our ongoing work at the ODI on areas like responsible data stewardship and AI data transparency from our Data-centric AI programme will directly support these efforts.
Conclusions and looking forward
An agenda for meaningful AI data transparency moving forward needs continued monitoring to raise attention to where transparency, and therefore accountability, is currently lacking - and clear standards set both through regulation and other forms of incentive to achieve this. At the ODI, we want to contribute towards building evidence about the real-world needs for transparency that will generate accountability to ensure transparency efforts are providing information in ways that will help the public, journalists, policymakers, developers and other decision makers to address their roles in responsible AI. We further aim to collaborate with our partners to build the infrastructure to ensure this is implemented.
In the context of AI safety, our immediate next steps involve pursuing collaborations aimed at developing rigorous evaluators and enhanced datasets, specifically targeting transparency and reliability issues across the AI lifecycle. Building on insights from MLCommons' benchmarking initiatives and the structured governance approaches advocated by Virginia Dignum, ODI intends to focus on data-centric strategies to address emerging risks in multimodal and agentic AI systems. We invite researchers and organisations interested in standardising robust, transparent methodologies for AI evaluation, particularly concerning dataset quality, adversarial robustness, and interpretability, to collaborate with us in shaping responsible, reliable AI practices going forward.
If you would like to collaborate with us on any of these topics, please don’t hesitate to email us at [email protected].