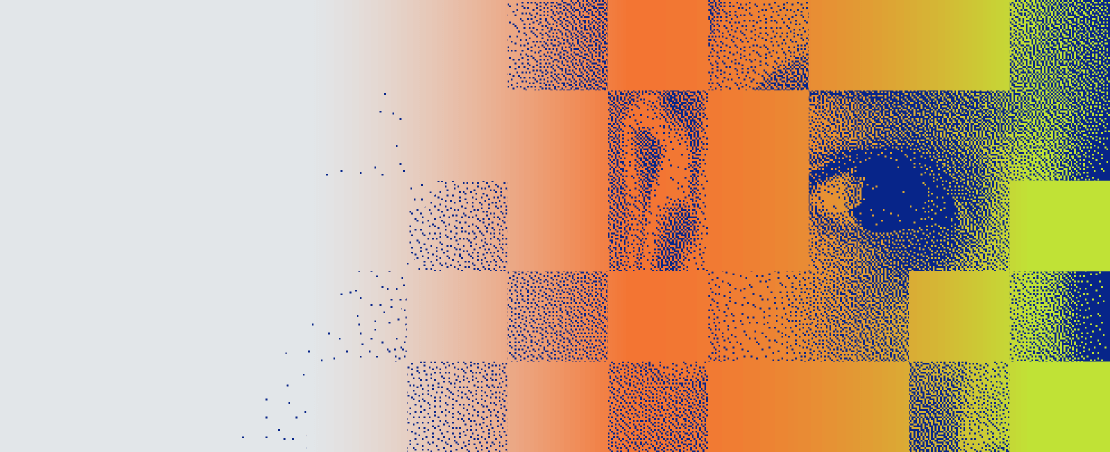
The General-Purpose AI Code of Practice (‘the Code’) represents an important move to shape a responsible artificial intelligence (AI) future. We have recently submitted two rounds of feedback on these guidelines, recommending that we focus on advancing data transparency as key to building trust and mitigating risks in AI.
Understanding the guidelines
The Code serves as a roadmap to make sure people design and use general-purpose AI systems responsibly. Its terms cover a lot of ground, from how to handle risks to the transparency needed for the ethical use of general-purpose AI technology. Promisingly, a key pillar of the Code’s aims is being as transparent as possible in documenting and sharing the data that AI models are built on.
Ensuring data is described clearly and made as transparent as possible via the Code isn't just something people should be obliged to do. Ultimately, it's the right thing to do. When people understand the data used in these systems, they have recourse to potentially trust them more and even provide feedback which may improve their outputs.
This is at the heart of how the Code can be made stronger. In our submissions, we emphasised the need to expand the scope of documentation requirements to include a range of other categories such as channels through which datasets have been distributed and the full legal entity name of any organisation involved in data annotation or other outsourced preprocessing services.
Moreover, we called for clarification of the key requirements, such as what is meant by a ‘general description’ of certain categories and how users may effectively describe multiple licences associated with data in combined datasets. Throughout the drafting of the Code, it became apparent that requiring licensing information about data once it has been ‘released’ is not always applicable—especially if providers have collected data themselves. We therefore advocated clarifying this in documentation guidelines.
Trust rests on being as transparent as possible
Our feedback also outlined how important it is to maintain accurate records of data to demonstrate the trustworthiness of AI systems. AI models rely on high-quality data and practitioners rely on metadata—or, data about the data, like lineage and provenance information—to develop and deploy AI systems responsibly. Without clear metadata, it's extremely difficult to verify the quality of a model's results or judge what risks it might perpetuate.
But this isn’t all, we also stressed that we need ways to record metadata that computers can read, like JSON-LD or RDF. Adhering to these languages helps to make metadata more accessible and machine-readable, by creating metadata that are structured, easy to access, and can be easily loaded onto other systems. This can allow developers, regulators, and other practitioners to examine these data, understand where it came from and make sure it can be assessed against established frameworks (eg, the UK Government Data Ethics Framework).
What's more, we could make the Code's current guidelines better by making sure datasets adhere to new metadata standards specifically for AI datasets like Croissant. These standards aim to make AI dataset descriptions the same across the board, which makes it simpler to share, reuse, and review them. Lining up with these standards wouldn't just improve the ease with which practitioners work together, it could also spark new developments by making it easier to find datasets and work collaboratively.
However, given the resource constraints often faced by small and medium-sized enterprises (SMEs), we recommended that the Code allow these organisations to report approximate figures for some requirements rather than requiring exhaustive metrics. This approach may lower compliance costs for smaller players while still championing transparency goals through proportionate, achievable requirements.
Putting ethical data governance first
Ethical issues around data gathering and handling played a key role in our input. The Code needs to demand detailed transparency about where training data comes from, how it's licensed, and how it's processed. This is especially true when datasets come from external providers or through outsourcing deals to drive accountability and inform relevant sociotechnical research. Furthermore, we proposed spelling out licensing terms in more detail, which means naming who owns the rights, what uses are allowed, and any limits. This depth of information lets downstream users feel more sure about whether datasets are okay to use for their specific projects.
Beyond contextual information about where the data comes from and what the limitations on its use are, we also highlighted the importance of embedding ethical questions in the Code’s criteria. Ethical assessments in data documentation means reporting on how entities gathered data, who took part, and if the methods followed established ethical guidelines (eg, UNESCO’s Recommendations on the Ethics of Artificial Intelligence). By adding these suggestions, the Code can help to tackle the spread of biases, make sure different groups are represented, and protect the rights of people whose data is used.
Data transparency can help address systemic risks
Systemic risks in AI are largely in the data, whether in the data itself (biases, gaps in documentation, lack of validation) or in the processes around the data (lack of transparency, lack of accountability). Our feedback asked the Code to acknowledge this by prioritising the identification and mitigation of data risks.
We suggested a taxonomy of systemic risks ranked by real-world impact, frequency and severity. This way the most critical risks (e.g. biased datasets in hiring algorithms or datasets used in disinformation campaigns) are addressed first. The Code could also require bias detection and mitigation techniques to be included in the documentation. Having this information communicated in standardised metadata allows stakeholders to track, measure and counteract biases, fostering fair and equitable AI outcomes.
Complying with current standards and documentation practices
We suggested adopting machine-readable schemas—such as the TESS vocabulary developed by the MLCommons community—to document and share testing processes. This would allow clear comparisons and analysis of safety and performance data across multiple models. In this way, the Code can further encourage consistent validation methodologies that increase trust and transparency by establishing a standard framework for reporting results.
We also encouraged adhering to the Robot Exclusion Protocol, a widely accepted standard by which website operators specify—usually through a “robots.txt” file—which parts of their site automated crawlers may or may not access. Asking for compliance with these requests helps promote a culture of consent and good-faith data gathering practices, reinforcing responsible data governance throughout AI development pipelines.
The biggest opportunity for improvement is making data and metadata available to all stakeholders. Open standards are key here to enable collaboration across the AI ecosystem. Given this, we encouraged reference to open metadata standards within the Code, so datasets can be indexed and findable through search engines or integrated into existing repositories like Hugging Face or Kaggle.
This would help to reduce the barriers to data access. As noted earlier, reducing these barriers would be especially valuable for smaller organisations and researchers who don’t have the resources to build their own data pipelines. Thus, we also advocated for modular documentation frameworks that can be adapted to the risks and complexity of each model. So all providers, big or small, can meet transparency requirements without being overwhelmed.
Looking to the future
The General-Purpose AI Code of Practice is a big step towards a more transparent and accountable AI world. By putting data transparency and governance at the heart of it, it provides a way to mitigate risks and innovate.
At the ODI, we were pleased to have been involved in this and will continue to promote best practices in data use and governance. A transparent AI ecosystem is not only possible but necessary for technology to serve the public interest.