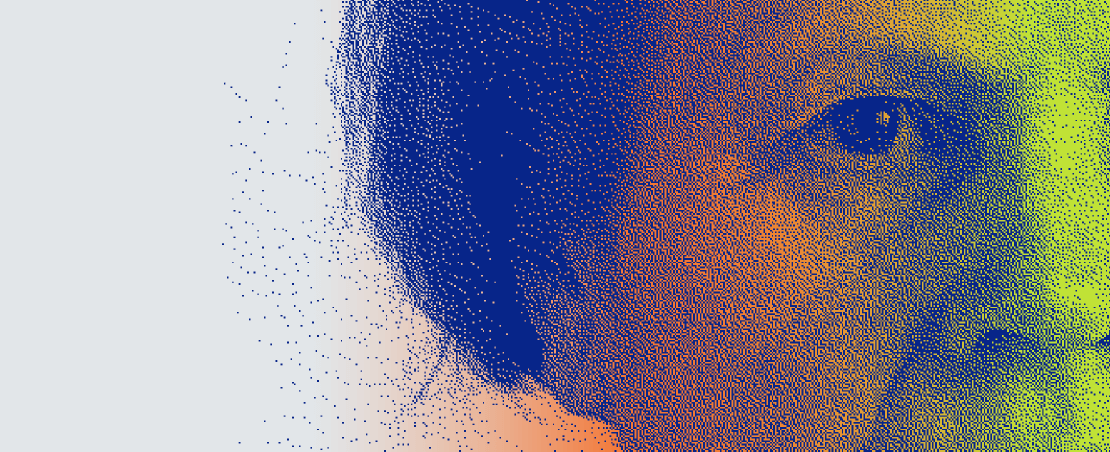
As AI systems become increasingly used in everyday work and life, understanding key aspects of how these systems have been created and how far to trust the outcomes is becoming more and more essential.
As we outline in an article we recently published in the Harvard Business Review, enormous, unwieldy and opaque data sources are used as the basis for producing the generative AI systems’ outcomes. The failure to publicly document the contents and usage of datasets hampers the ability of developers, researchers, ethicists, and lawmakers to address various issues such as biases, harmful content, copyright concerns, and risks to personal or sensitive data. This lack of documentation spans all data elements, including training and fine-tuning datasets, as well as the sourcing and labelling processes.
The demand for AI transparency has become increasingly recognised in recent years. This has led to parts of the AI community making significant progress and contributions to AI data transparency, including the increasing emergence and uptake of standardised transparency guidelines. For example, but not limited to, Hugging Face, a vast repository of AI models and datasets, promoting the use of Model Cards and Dataset Cards to its community of developers. In another example, the Croissant initiative, supported by major platforms like Tensorflow and Hugging Face, provides machine-readable metadata (information about the datasets) for machine learning (ML) datasets, improving their accessibility, discoverability, and reproducibility and also helping to improve the management and accountability of work with the datasets by AI practitioners. All these resources guide developers on documenting how a model dataset was created and what it contains as well as potential legal or ethical issues to consider when working with it. Lawmakers are also responding to increasing demands by proposing legislation that specifically addresses AI data transparency - a topic we discuss further in our first policy position (of five we will publish in total) on what is needed to build the strong data infrastructure needed to realise responsible AI.
Amidst the need for greater transparency in AI data practices, a lack of systematic monitoring methods persists across many systems. An October 2023 study of 10 key generative AI (‘foundation’) models by Stanford researchers highlighted that among general low transparency across AI system development, transparency about data is particularly poor. A recently released update to the study included several more models and noted slight improvement by some developers, but overall, there is still poor data transparency.
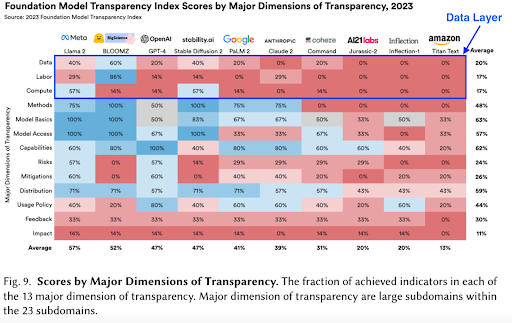
In a forthcoming study by members of our data-centric AI research team, we replicated the analysis on a wider range of 54 AI systems that are causing public concern, having been at the centre of AI incidents recorded in the Partnership of AI's AI Incidents Database. We found that only a minority of these AI systems provided identifiable information about their underlying models and data practices. Transparency scores (evaluated for those systems offering basic model transparency information) were low across all indicators including data size, data sources and curation, with each indicator present in less than 40% of the models evaluated. Almost none of the systems scored included information about the inclusion of copyrighted data, personal information in data, or the use of data licences.
To build on our findings, we are developing an AI data transparency index to provide a clearer picture of how data transparency varies across different types of system providers, based on a deeper understanding of the needs for such information. Investigating the need for data transparency within the ecosystem will build on current evidence, including recent Open Futures research on transparency documentation. Further research will focus on empowering non-specialists and communities with transparency information, and on understanding the barriers and opportunities for AI practitioners to communicate data transparency effectively.
While transparency cannot be considered a ‘silver bullet’ for addressing the ethical challenges associated with AI systems, or building trust, it is a prerequisite for informed decision-making and other forms of intervention like regulation. If you are interested in collaborating with us on our ongoing research and advocacy in this area or would like to discuss this work further, please get in touch.