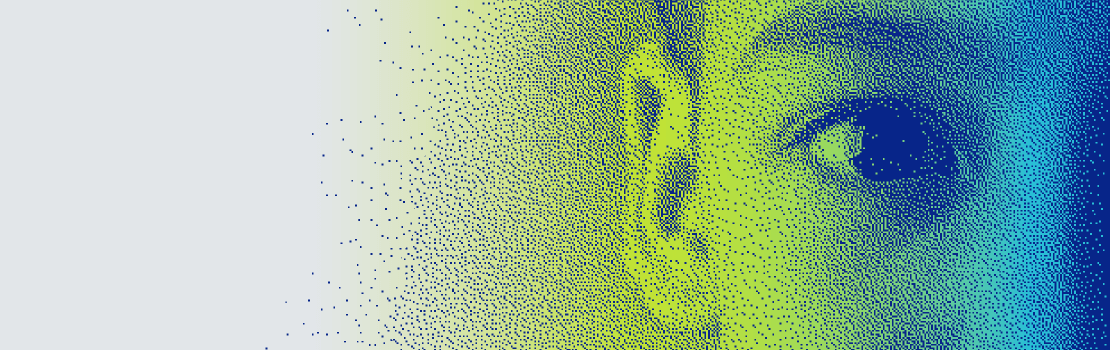
Join us for this webinar with Lora Aroyo, Research Scientist at Google Research in NYC, chaired by the ODI’s Director of Research Professor Elena Simperl.
In this session, Lora Aroya highlights the importance of culturally-aware and society-centred research on the data used in training and evaluating machine models and fostering responsible AI deployment in diverse sociocultural contexts. She presents a number of data-centric use cases that illustrate the inherent ambiguity of content and natural diversity of human perspectives. These cause unavoidable disagreement that needs to be treated as signal and not noise.
Lora will present her research for 30 minutes, and this will be followed by a Q&A and discussion.
This webinar is perfect for anyone who wants to gain expertise on data-centric AI, and is interested in the importance of data in creating safe, trustworthy and responsible AI.
This session is the first in our Data-Centric AI webinar series.
Can't come to the event, but would like to see the recording? Then join our research email list and we'll email you as soon as it is released, along with updates of all our latest research.
Please note:
The Zoom link for the event will be emailed to all bookers.
This event will be filmed, if you do not wish to be filmed please keep your camera turned off.
Session abstract: the many faces of responsible AI
Conventional machine learning paradigms often rely on binary distinctions between positive and negative examples, disregarding the nuanced subjectivity that permeates real-world tasks and content. This simplistic dichotomy has served us well so far, but because it obscures the inherent diversity in human perspectives and opinions, as well as the inherent ambiguity of content and tasks, it poses limitations on model performance aligned with real-world expectations. This becomes even more critical when we study the impact and potential multifaceted risks associated with the adoption of emerging generative AI capabilities across different cultures and geographies. To address this, we argue that to achieve robust and responsible AI systems we need to shift our focus away from a single point of truth and weave in a diversity of perspectives in the data used by AI systems to ensure the trust, safety and reliability of model outputs.
In this session, Lora Aroya presents a number of data-centric use cases that illustrate the inherent ambiguity of content and natural diversity of human perspectives that cause unavoidable disagreement that needs to be treated as signal and not noise. This leads to a call for action to establish culturally-aware and society-centered research on impacts of data quality and data diversity for the purposes of training and evaluating ML models and fostering responsible AI deployment in diverse sociocultural contexts.
Speakers' biographies
Lora Aroyo
Research Scientist, Google
I am a research scientist at Google Research NYC where I work on Data Excellence for AI. My team DEER (Data Excellence for Evaluating Responsibly) is part of the Responsible AI (RAI) organization. Our work is focused on developing metrics and methodologies to measure the quality of human-labeled or machine-generated data. The specific scope of this work is for gathering and evaluation of adversarial data for Safety evaluation of Generative AI systems. I received MSc in Computer Science from Sofia University, Bulgaria, and PhD from Twente University, The Netherlands. I am currently serving as a co-chair of the steering committee for the AAAI HCOMP conference series and I am a member of the DataPerf working group at MLCommons for benchmarking data-centric AI. Check out our data-centric challenge Adversarial Nibbler supported by Kaggle, Hugging Face and MLCommons. Prior to joining Google, I was a computer science professor heading the User-Centric Data Science research group at the VU University Amsterdam. Our team invented the CrowdTruth crowdsourcing method jointly with the Watson team at IBM. This method has been applied in various domains such as digital humanities, medical and online multimedia. I also guided the human-in-the-loop strategies as a Chief Scientist at a NY-based startup Tagasauris. Some of my prior community contributions include president of the User Modeling Society, program co-chair of The Web Conference 2023, member of the ACM SIGCHI conferences board. For a list of my publications, please see my profile on Google Scholar.
Elena Simperl
Director of Research, ODI
Elena Simperl is the ODI’s Director of Research and a Professor of Computer Science at King’s College London. She is also a Fellow of the British Computer Society, a Fellow of the Royal Society of Arts, a senior member of the Society for the Study of AI and Simulation of Behaviour, and a Hans Fischer Senior Fellow.
Elena’s research is in human-centric AI, exploring socio-technical questions around the management, use, and governance of data in AI applications. According to AMiner, she is in the top 100 most influential scholars in knowledge engineering of the last decade. She also features in the Women in AI 2000 ranking.
In her 15-year career, she has led 14 national and international research projects, contributing to another 26. She leads the ODI’s programme of research on data-centric AI, which studies and designs the socio-technical data infrastructure of AI models and applications. Elena chaired several conferences in artificial intelligence, social computing, and data innovation. She is the president of the Semantic Web Science Association.
Elena is passionate about ensuring that AI technologies and applications allow everyone to take advantage of their opportunities, whether that is by making AI more participatory by design, investing in novel AI literacy interventions, or paying more attention to the stewardship and governance of data in AI.