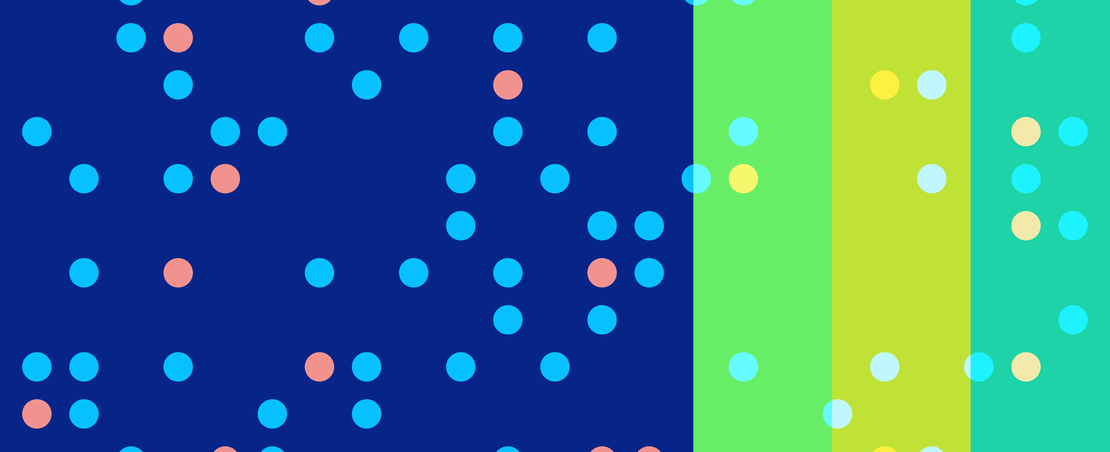
"Without data, there is no AI" has become a mantra for the ODI, and prominently featured in framing the ODI Summit event in November 2023. It actually predates its use here; it's a concept that has circulated within Artificial Intelligence (AI). For us at the ODI, it refers to the data infrastructure of AI – including data assets, tools, standards, practices, and communities. It is a call to look at data and other socio-technical foundations of AI to better understand their design, outcomes and implications.
We have recently launched our Data-centric AI research programme, where we aim to unpack many of these topics. In the coming months, we’ll publish a series of blogs, articles and short papers as our work progresses. Today – 22nd December 2023 – we are publishing our first short paper, looking at data's fundamental role in AI, exploring some of the complexities surrounding recent developments, and covering the themes outlined in this blog.
The AI lifecycle and data's pivotal role
Data is the cornerstone of AI systems, guiding every stage from conception to operation; it provides the information that a machine learning model is trained on and learns from. It is collected, wrangled, curated, aggregated and then used in models. Data is used to test and benchmark a model’s success, and data is inputted for utilisation once a model is operational.
Data sources for AI vary, especially those used in foundational models that require immense quantities. They can include web-crawled data, enterprise data, or a combination and fall into a number of broad categories, including:
- Textual data: CommonCrawl's extensive archive is used in training models like GPT-3.
- Visual data: Tools like Stable Diffusion, trained on billions of internet-scraped images, have raised ethical concerns.
- Synthetic data: Used to enhance dataset diversity, especially in contexts where historical data is inadequate.
Key challenges
The scale and complexity of the use of data within artificial intelligence, combined with obfuscation – i.e. so-called black-box algorithms – can make AI unknowable. Investigating AI datasets is essential for reaching a greater understanding of their capabilities and limitations, identifying biases, and assessing potential harms. This includes questioning the volume of data required and considering environmental impacts.
With the scale of datasets used, there is also concern about potential ‘model collapse’ where AI models are trained on synthetic data rather than human-generated data and therefore become divorced from ‘real’ data and ‘real’ events to the point of unusability.
Governance and accountability in AI
There is a real and urgent requirement for robust governance models in AI. There may be a need to have public audits of datasets or mandatory reports of training data sources – as included in the EU AI Act. In the USA, the FTC's demand for transparency in OpenAI's data sources may also signal a shift towards greater accountability. Accountability is often considered a cornerstone of the safe development of AI and is also included in the OECD’s AI principles: ‘Actors should be accountable for the proper functioning of AI systems and for the respect of the above principles, based on their roles, the context, and consistent with the state of art.’
Future direction
As well as building understanding and exploring accountability and governance in data-centric AI, the ODI's research aims to investigate other intricacies in the relationship between AI and data, focusing on a number of key gaps in existing research. These are set out in our short paper and include:
- Investigating data – in harmony with other considerations like better and more safe model design, focusing on the data – and the models – opens up the opportunity to analyse the data sources, spot and test for bias, and identify data quality or collection issues.
- Making data AI-ready – whether in response to issues highlighted or as a systematic attempt to prevent AI harm – steps can be taken to ensure data is ready for application in AI systems.
- Setting frameworks and benchmarks for AI safety.
- When and if we should stop developing AI if risks become too big or uncontrollable.
- All of the focus – so far – has been on improving the data within current development models. There also needs to be opportunities to learn from these investigations to change how the development takes place.
If you’d like to discuss our Data-centric AI research and what we have planned, get in touch.