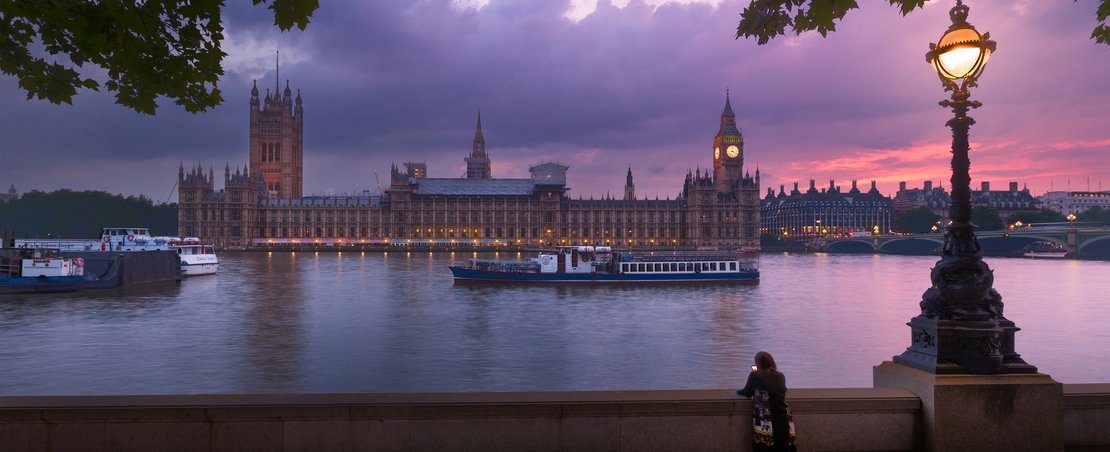
Our Global Head of Policy, Resham Kotecha, has a public appointment as Deputy Chair of the Social Mobility Commission (SMC). Last week, she and her SMC colleagues appeared in front of a House of Lords Special Committee on Social Mobility (you can watch the full evidence session here) and below are the key points on social mobility and data.
In an era where data is more abundant than ever before, the challenge is not just in collecting it but ensuring that it is of high quality, reliable, and usable. The ability of any government to react to changes in circumstances in real-time and to design effective policies depends on reliable and well-integrated data.
The NEET challenge: A case for better data
One pressing example of where better data could support a significant societal challenge is the percentage of young people classified as NEET (Not in Education, Employment, or Training). We know that if people are NEET early in their lives, they are far less likely to enter work, far more likely to have mental health issues later in life, and report being less happy.
In 2021, the NEET rate reached its lowest level in over a decade - a significant achievement - and positive news for society and the economy. However, following the COVID-19 pandemic and other global economic shocks, NEET levels are now at their highest since 2011. The economic consequences of youth unemployment are severe, and the fact that current rates are so high means that we have a generation of young people who will potentially suffer for many years, if not decades.
However, due to a number of different local, devolved, and national government departments collecting and maintaining - but crucially not sharing - data on young people, it is very difficult to have a full sense as to why so many young people are currently NEET. This makes it much harder for policymakers to design effective solutions to support these young people back into education, employment, or training.
Data collection issues and the Labour Force Survey
Accurate data collection remains a significant hurdle. The Labour Force Survey (LFS), for instance, has recently been negatively highlighted for unreliable findings.
A recent case highlighted that a single question received only five responses, shifting the analysis by 30 percentage points - a stark reminder of the importance of data reliability and the need for data assurance so that we know we can trust the data. Post-pandemic, response rates have declined, forcing the Office for National Statistics (ONS) to double outreach efforts, significantly increasing the cost and resources required to collect data. Without robust data, policymakers cannot accurately assess the impact of past policies or determine future needs.
Bridging data gaps and enhancing interoperability
While the UK has rich datasets across various government departments - including the Treasury, Department for Work and Pensions (DWP), and Department for Education (DfE) - these datasets are often siloed and do not communicate effectively.
To get the most out of the different datasets that the government holds, we need to ensure the creation of interoperable systems. This is akin to using an adapter for international travel - a way in which different datasets can “speak” to each other and be brought together. Ensuring interoperability would allow for a more comprehensive understanding of social mobility and economic challenges.
A prime example is the Longitudinal Educational Outcomes (LEO) dataset, which links educational outcomes with labour market performance later in life. This means we can get an idea of how someone’s educational achievements impact their careers later on. However, it does not currently incorporate household income data, which could provide deeper insights into the relationship between socioeconomic background and life opportunities. If we were to link these two datasets with the Parent Pupil Match dataset (PPMD), it would help policymakers better target support for disadvantaged students. If we could also link household economic data with the LEO and PPMD, we would be able to see how the socioeconomic background of someone’s parents/household influenced their educational and labour outcomes
Learning from the US model
Currently, there are limitations in the UK in terms of data relating to income and households. We can, and should, look to the US for inspiration – researchers have been able to produce valuable and granular analyses of social mobility, linking both income and households - and they’ve been able to do this over time and by geography. This was done to great effect by Raj Chetty, who led a number of brilliant studies on social mobility in the US.
Chetty has used anonymised data from federal income tax returns to map trends in intergenerational mobility, both geographically and over time. The US has demonstrated the power of large-scale, anonymised data integration.
Implementing a similar approach in the UK - linking PAYE data from HMRC with school records - could significantly enhance policy effectiveness by identifying those who truly need support and showing policymakers how much of a difference household income makes to a child’s life chances.
Challenges in evaluating policy effectiveness
Policymakers often implement new initiatives without designing them for proper evaluation at the start - rather they attempt to evaluate them retrospectively with incomplete or proxy data.
Programmes like Sure Start were later assessed based on proximity to a Sure Start centre rather than comprehensive pre-collected data on participants' backgrounds or level of interaction with the centre.
Similarly, government efforts to shift Civil Service jobs outside London have not been thoroughly examined to determine whether they create new opportunities for underserved communities or merely relocate existing roles for advantaged employees.
Higher education data also reveals hidden inequalities and the fact that for disadvantaged young people, “choices” are not really choices at all. Over half of university students in the UK attend an institution within 55 miles of their home, largely due to the cost of living and needing to be able to commute from home. Disadvantaged students are more likely to make university choices based on gography rather than academic fit, affecting their long-term outcomes. Collecting more granular data on these decisions would enable better interventions, and the government could design better support packages for disadvantaged young people.
The role of AI and emerging technologies in data utilisation
AI has enormous and exciting potential in data analysis, but it is only as good as the quality of the data that it relies on. Biases in data collection, and gaps in data, can skew AI-driven insights, as can data biases. These issues all underscore the need for diverse perspectives in data collection, maintenance, assurance and governance.
AI can also democratise education, providing personalised tutoring for struggling students - but we need to address digital access barriers and incorporate data literacy into our education system.
While the potential of AI is very exciting, it also presents risks. Algorithms shape social discourse and have the potential to amplify misinformation or harmful ideologies. Without critical engagement with emerging technologies, young people, especially the most vulnerable and least advantaged, may be disproportionately affected by misleading narratives.
Building trust and ensuring data safeguards
Public trust is critical if we are to truly leverage the potential of data and AI. Communities - particularly minoritised ones - must feel confident that data collected about them will be used ethically, transparently, and in a way that benefits them. This requires clear communication and education about how data is used, strong legal safeguards to prevent misuse, and easy and accessible mechanisms for individuals to be able to understand and challenge how their data is handled. Without these foundations, trust will be undermined, individuals may feel misrepresented, and we will lose the benefit of data and AI to address NEETs and other pressing societal issues.
When it comes to definitions for data categorisation, people often misdefine themselves - intentionally or not - due to stigma, misunderstanding, or a lack of clarity. A young person juggling caring responsibilities, or mental health challenges may not identify as NEET, even if they technically qualify. People often conflate their family stories with their own, so people who have grown up in professional households often classify themselves as a lower class than they are because of their family roots and the stories they have heard about the challenges their parents had growing up. Poor definitions and miscategorisation can obscure the true nature of disadvantage and can lead to misguided policy responses. Reflecting the complexity of people’s lives requires high-quality and precise data, alongside empathetic and inclusive approaches to data design and interpretation.
Shifting family patterns and rising childcare costs also reveal how data and policy often lag behind social reality.
In 2020, for the first time in the UK, the average age of a first-time mother rose above 30. This rise in age for first babies is due to a number of factors - partly due to career considerations but significantly by the soaring cost of childcare across the UK. The UK now has the highest childcare costs as a share of household income in the OECD, and outside of London and the South East, there are many childcare ‘deserts’. Women from lower-income households face a further financial challenge - benefit and welfare systems can unintentionally disincentivise returning to work, particularly when childcare costs exceed earnings.
Without targeted data to identify these systemic traps, we might presume explanations for why some young women are NEET that mean the policy solutions are the wrong ones, when in reality, they cannot access or afford childcare. Policies can risk reinforcing cycles of exclusion and can lead to money spent in the wrong way. A joined-up approach to data must consider these real-world dynamics while also sharing data across local and national authorities to enable smarter welfare design that recognises the barriers many women face in re-entering work or education.
Treating data as public infrastructure
In order for data to drive meaningful change, the government must treat it as a form of public infrastructure, ensuring high-quality, well-integrated, and widely accessible datasets. This requires both political will and the resources to sit alongside it, as well as long-term cross-party commitments. By improving data interoperability, ensuring robust evaluation of policies, and leveraging AI responsibly, we can create a more informed, responsive, and equitable society where the circumstances of your birth do not determine your future.