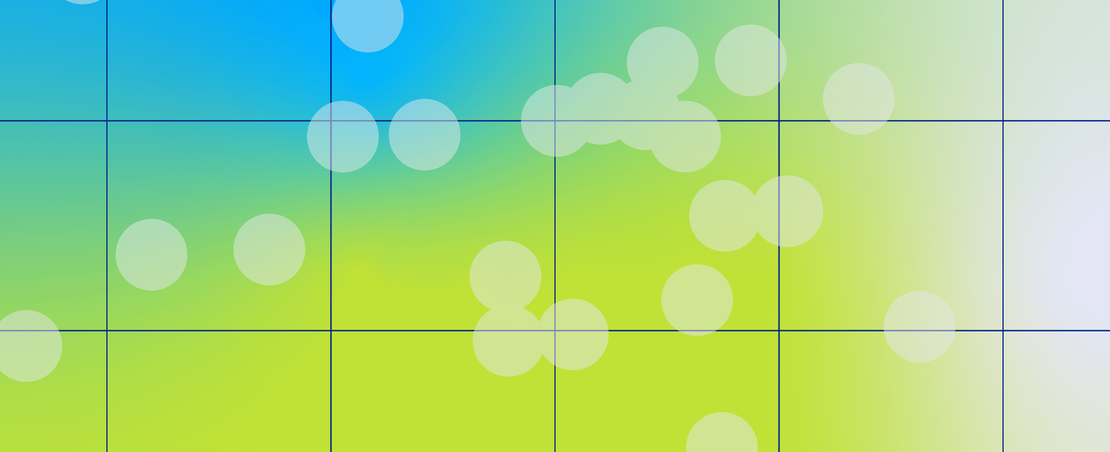
Communities exist in all sorts of shapes and sizes, from small groups who come together at local village halls for chess clubs through to huge communities of sports fans who follow their teams from all over the world. There are also communities who come together with the aim of collecting, maintaining and sharing data, which we call data communities.
There are well known examples, like Wikipedia and OpenStreetMap, which have hundreds of thousands of contributors from around the world. For reference, the English language Wikipedia is home to nearly seven million articles, with edits being made at a rate of 2 edits per second. In 2023 there were nearly a million active editors on Wikipedia.
There are also smaller, more localised examples of data communities, which are focused on local issues. For example, CocoZap, supported by DataLabe in Brazil, enables communities to map out sanitation (or lack thereof) in their favelas, which creates datasets that they can use to campaign for improvements. And Dakshin, based in India, is a community of local fishers who collect data about their expertise on the ocean where they fish, such that they have a seat at the table to discuss their lived realities, and advocate for their communities. There are also examples like the Toilet Map, which is a crowdsourced list of all of the publicly accessible toilets in London, while Habitat Map supports communities to measure air quality in their local area.
The advent of generative AI, and in particular with the launch of ChatGPT in 2022, was a pivotal moment in the development of Wikipedia, and many of the other data communities around the world.
Some are concerned that these tools may spell the end of communities like Wikipedia. There are potential issues in using generative AI in writing posts, or producing new data points due to their well cited ability to hallucinate. Their ability to regurgitate information, without referencing its source, leads to concerns about reductions in traffic to the communities themselves if people start to rely upon AI chatbots for information.
However, there is a huge opportunity for generative AI to support data communities, for example via the creation of new articles or data points, translations into other languages, as well as summarising discussion pages.
Different communities have been impacted in different ways. Recent research suggests that the impact has been limited, both in regards to visits to the online encyclopaedia as well as in terms of the number of edits. While the decline in the use of Stack Overflow has been well documented.
The future of data communities in the era of generative AI is far from certain. At a recent convening of experts around the future of knowledge commons like Wikipedia and Ushahidi in the era of generative AI, a draft research agenda was created, setting out the key themes which require further research and understanding. They found there to be a need to:
- Characterise and monitor the use of AI in the knowledge commons over time
- Develop AI tools for the Knowledge Commons
- Evaluate the effect and impact of deploying AI tools
- Empower Knowledge Commons communities in the AI era.
Wikipedia is different from many other data communities around the world. It is enormous in size, has well established rules and norms, as well as significant funding to sustain its future. But what about other communities like Wikipedia - how have they been impacted by the developments in generative AI? Are there differences across different types of community, geographies or size of community?
At the ODI and Aapti Institute, we’re interested in the different ways that people and communities can be more involved in the data economy. Data communities are one way that people can participate, and have more agency and control over how the data ecosystem reflects their lived reality and interests.
This long read explores what data communities are, how different data communities are being impacted by generative AI, and looking forward, how they can thrive in the era of generative AI.
What are data communities?
So what are data communities? In Should you believe Wikipedia?, Amy Bruckman argues that community refers to "a category of associations of groups of people”, and the strength of connection of the community relies upon the social capital between the members of the community. Operating in a digital space enables these social ties to become more persistent and pervasive, making it easier to connect people. Communities usually operate in third spaces, neutral areas that are neither work nor home, and many online websites enable this environment.
Data communities refer to communities which are connected by working with data. This could be through the generation of new data points, or the collective stewardship of data. Data communities come in lots of shapes and sizes, and some examples include efforts to collect data about butterflies in their back gardens, young runners capturing data about air quality in West African cities or local communities mapping a new road on OpenStreetMap.
How does generative AI impact data communities?
Generative AI is a category of AI-based systems that generate new outputs based on insights and patterns identified during training. ChatGPT, which generates text, is perhaps the most well known example of generative AI, but generative AI systems can create new data in the form of images, audio and more.
This section details more broadly some of the different ways that data generated by communities can be affected by this technology. These concerns are becoming increasingly widespread, with organisations like GovLab and OpenFuture looking at commons based approaches to the development and governance of AI. There are three ways which we see generative AI interacting with data communities:
- In the training data for AI models. While Wikipedia is one of the most widely used datasets for training generative AI models, other communities' datasets, like Reddit, StackOverflow, Zooniverse have been used for training AI models. Additionally, other communities' data could be collected through wider scraping practices in datasets such as common crawl.
- As a tool for the data community, for example via supporting the generation of new data labels, providing summaries of key discussion points, translations between community members, or assisting with mapping.
- In wider social norms around how people access and share data. Generative AI models are altering how we interact with the Internet, and the websites that we visit. They may become all encompassing tools, which mean we never need to visit other data communities’ websites for our recipes, solutions to coding problems, or discussion boards. It is worth noting that recent research showed that few people use generative AI tools like ChatGPT regularly at the moment, and those who do are primarily younger people (18-24). Therefore this trend may not currently occur, but in the future is likely to be a larger issue. Examples such as the decline in users of Stack Overflow due to the quality of code generators demonstrates the potential unintended consequences these generative AI tools can have on communities.
Data communities as a source of training data for AI models
Although most generative AI developers do not usually disclose the datasets the models were trained on, there is broad consensus that these tools are typically trained on datasets created by crawling the internet for publicly available data. This includes data and content protected by intellectual property rights and there are a number of cases around the legality of the use of such data to train generative AI models. Many artist communities are also building tools that “poison” AI models. Nightshade, is one such example that turns any image into a data sample that is unsuitable for model training. It makes it such that models training on these images without consent will see the models learn unpredictable behaviours that deviate from expected norms. Glaze is another example which looks to protect against style mimicry, by altering artwork such that it appears to be a drastically different style to an AI model while appearing unchanged to the human eye.
Certain communities, such as ones in Singapore, are outright preventing access to their data citing the extractive practices of most large AI models, while others are finding other ways of making the data accessible. Te Hiku Media, which has created a vast database of the Maori language, has developed a special licence that allows access to the database only if an organisation agrees to respect Māori values and share benefits derived from its use back to the Māori people.
The last few months have seen companies that build generative AI models sign agreements with large online platforms, including data communities such as Stack Overflow and Reddit, for the use of their data to train these models. In the case of Stack Overflow, many users have altered or deleted their posts and comments in protest, arguing that this steals the labour of the users who contributed to the platform. This has resulted in users being banned by Stack Overflow.
The agreements to use data communities as source of training data have been signed mostly with large communities - given the quantum of data typically required to train generative AI models. OpenAI’s attorneys have noted that it is impossible to licence all training data, and it is possible that data from smaller, less-resourced data communities are used to train generative AI models, regardless of an agreement with the community.
Generative AI tools to support data communities
Gen AI is not the first AI tool to be used by data communities. Various AI tools are being used by data communities in a variety of ways, and have been for many years - especially for automation. As mentioned above, Wikipedia editors have been using bots since 2002 for purposes including translation and editing. Ushahidi, an open source software application which utilises user-generated reports to collate and map data, is using AI for translation, geolocation and form assignment. Users on platforms like Zooniverse, a citizen science web portal, use AI tools for purposes such as image processing as well as complex large-scale data analysis. Generative AI tools in a similar vein show promise in providing efficiency gains and improving functionality and accessibility. Some data communities are already exploring the potential uses of tools like ChatGPT to complement user efforts. For example, researchers have explored the use of ChatGPT to enrich maps in OpenStreetMaps by improving the accuracy of mapping suggestions by nearly 30%. Wikipedia has developed a plugin that allows ChatGPT to search for and summarise the most up-to-date information on Wikipedia in answer to general knowledge queries.
Generative AI tools and engagement in data communities
A potentially critical impact of generative AI tools is their effect on engagement in data communities. Many generative AI tools and assistants have become popular sources for information and serve as self-contained portals for knowledge gathering, potentially eliminating the need to visit other websites. This has raised the question of whether these tools are negatively impacting engagement on online data communities, many of which they are trained on. ChatGPT’s growth has given rise to various discussions that allude to the existential threat it poses for Wikipedia. This is not the first instance of a new digital tool surfacing a threat to Wikipedia. Google search potentially served as a threat to Wikipedia, but studies have since shown that Google search and Wikipedia have a mutually beneficial relationship, with only Google’s Knowledge Graph potentially surfacing a threat. In 2022 Google became one of the first customers of Wikimedia’s enterprise service.
Recent studies that explore engagement in data communities such as Wikipedia, StackOverflow and Reddit paint a more complicated picture when it comes to the effect of ChatGPT. Burtch, Lee & Chen analysed the effect of ChatGPT on engagement on Stack Overflow and Reddit. Their analysis revealed that there was a significant decline in engagement on Stack Overflow. The decline in engagement also seemed to be with newer user accounts, indicating that more junior and less experienced users were choosing to engage more with ChatGPT than Stack Overflow. Furthermore, the decline in engagement was also higher on topics related to open-source tools and general-purpose programming languages as compared to proprietary and closed technologies, with the former having more public sources of training data. This decline in engagement on Stack Overflow however was contrasted with no impact on engagement on subreddits related to analogous subject matter.
In the context of Wikipedia, Reeves, Yin & Simperl’s study found an increase in page views and visiting users on a majority of Wikipedia language editions. Of note, however, is that there was a larger increase in engagement for languages from countries where ChatGPT was unavailable, indicating that ChatGPT potentially led to a lower increase in engagement with Wikipedia language editions for which ChatGPT was available. There was no impact of ChatGPT on the number of edits or editors. The Wikimedia Foundation also noted no noticeable shift away from current trends for getting to Wikipedia content, even with the emergence of ChatGPT.
The idea that many individuals are relying on generative AI tools as a source of knowledge gathering in lieu of other data communities does not seem to apply as a blanket statement. Rather, contemporary research indicates that the generative AI tools are having a differential impact based on the nature of knowledge sought to be gathered / acquired. That said, the decrease in engagement in a data community like Stack Overflow has concerning consequences not just for the community itself, but also for generative AI tools that use such a community as a source of training data. The lack of decline in engagement on analogous subreddits has led to the conclusion that a social characteristic can make data communities more resilient to generative AI’s impact on engagement. However, it is to be acknowledged that reduction in engagement seen with Stack Overflow has largely not been mirrored in other data communities.
In addition to the nature of the data community, another big factor that affects engagement impact is language. While ChatGPT works well with English and a few other globally dominant languages, it performs poorly at generating in non-Latin script languages. This is especially evident in the case of low-resource languages, i.e., languages which do not appear online as much. Beyond “hallucinating”, ChatGPT was found to make up words in low-resource languages. The current lack of reliability of generative AI systems in the context of low-resource languages is likely to translate into greater resilience in engagement for data communities that operate in these languages. This does not mean that communities of low-resource languages are not using ChatGPT based systems. Jugalbandi is one such example. It is a free and open platform that combines ChatGPT and Indian language translation models under the Bhashini mission to power conversational AI solutions. One of the applications of Jugalbandi is Whatsapp and Telegram bots that help Indian citizens understand their rights and the application process for over 50 government schemes in local languages. The impact of this remains to be evaluated.
How can generative AI work for data communities?
Generative AI tools have the potential to improve data communities more widely. As prior examples show, they could refine the quality of user generated content, improve accessibility to data communities through translations, summarising long articles or discussions and text to voice generation, and create efficiency gains through different types of automation. However, generative AI also poses challenges. The increasing use of generative AI tools has, in certain contexts, led to a reduction in engagement with data communities - a phenomenon that has negative implications for the communities as well as the tools themselves. The lack of reliability of generative AI tools in the context of low-resource languages risks further widening digital inequalities, as those populations are further excluded from accessing and using technology.
Addressing some of these challenges requires maintaining a strong, accountable relationship between generative AI companies and data communities - examples of this include Wikipedia’s enterprise service and Zooniverse’s consultancy service, both of which provide data and services to AI companies. Building reliable, responsible generative AI models requires access to clean, diverse, human generated data. Supporting data communities that create this data is a strong starting point. Going beyond, AI companies can also explore ways to encourage users of data communities to train and refine generative AI tools - allowing for the community to have a sense of involvement and agency in the development of these tools. in a manner that is not viewed as extractive of their labour.
More broadly, the design and development of generative AI systems must lean on tenets of participatory governance. Companies like OpenAI and Meta already have initiatives that seek to include communities in decision-making around generative AI. However, there is a need to ensure that such initiatives include communities from early stages of planning, and ensure continuous and meaningful engagement to ensure effective participation. Policies employed by data communities that govern the collection, sharing and use of their data can also serve as a blueprint for how large datasets (used for AI training) are governed. The licence developed by Te Hiku Media (referenced above) and Karya’s Public License are examples of how data can be shared to train AI systems in ways that ensure direct benefit to the communities. While there are some examples of best practices in this context, it remains an active area of research.
Data communities themselves can also take the lead in making generative AI tools work for them. Large communities, such as Wikipedia, can theoretically leverage their commons-based ecosystem to create generative AI models with builders who place an emphasis on the creation of responsible and fair systems. However, doing so is not straightforward. Training AI models, especially large ones, is very expensive. Associated with this high cost is access to compute power (in the form of GPUs) - which is difficult and expensive. Private organisations and governments are looking to address this by making GPUs easily accessible at low costs. There is a trend towards using smaller models that require much less data and compute as an alternative, and even involving communities in the curation of these small scale datasets.
With this said, it is also important to acknowledge that there are limitations to participatory governance and the inclusion of data communities in the development of generative AI systems. Participation requires capacity on various fronts - time, resources and knowledge - that many data communities do not necessarily possess.
Next steps
A first step to empowering data communities in the era of generative AI is to comprehensively understand the extent of generative AI’s impact on them. While there are numerous contemporary research efforts, there is a need for further exploration on the nuances of the impact of generative AI on data communities. There remain open questions around the impact of generative AI tools on the engagement with communities in low-resource language contexts. And broader longitudinal studies will be required to understand the longer term effects.
We are seeking to continue to explore how generative AI is altering the ways communities function online. We’re particularly interested in how these tools can practically enable data communities to focus on the activities they want to, by enabling them to be more efficient, as well as the long term unintended impacts of generative AI on communities. Finally, we’re seeking to learn more about the different strategies communities are putting in place to protect themselves against the advances of generative AI, including participatory governance models, licences and more.
The data created by communities is a critical part of enabling communities to be seen in a digitised world, but is also important for solving societal challenges and for technological development. If you’d like to learn more about this work, or to collaborate with us on this topic, please contact [email protected].