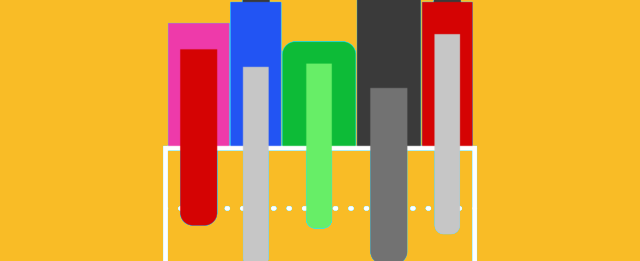
The research stream of the ODI’s ongoing Luminate-funded Covid-19 project began in earnest recently, and we wanted to share this work note about what we are doing
Initial aim
We are looking at what data is already being published in relation to Covid-19. In particular, looking at and thinking about standards, data quality and consistency, with the aim of finding specific examples that highlight bigger issues around Covid-19 data.
We are examining various sources of data – mainly using the valuable resources of the Coronavirus Tech Handbook and GovLab’s Data4Covid19 repository.
Dataset lists
As we research these dataset lists (which often link to other dataset lists), we are finding that some are extremely clear and easy to browse while others are more scattered and less structured. Well-organised repositories have filtering, tags, search, file types, authors, and metadata, while more casual ones often just have URLs with a one-sentence description of the data.
This question of looking for information within collections of datasets links to some previous work the ODI did in partnership with the University of Southampton about dataset search. In a paper, Dataset search: a survey, the authors outline the problem.
'Currently, there is a disconnect between what datasets are available, what dataset a user needs, and what datasets a user can actually find, trust and is able to use. Dataset search is largely keyword-based over published metadata, whether it is performed over web crawls or within organizational holdings. There are several problems with this approach.'
….
'In other words, in a dataset search context, approaches need to consider additional aspects such as data provenance, annotations, quality, granularity of content, and schema to effectively evaluate a dataset’s fitness for a particular use.'
Rather than simply create more lists of data, we are considering how to get data to where it's needed – to the people who need it. In our shared document, Covid-19: Helping data get to where it’s needed, we state:
'Information requirement gathering: Different people and organisations have different requirements for data. These need to be clearly articulated, in particular to stop people from asking for data that is interesting rather than data that helps inform decision making. This requires critical user research and design skills (because showing mock-ups is more helpful than asking abstract questions) to help identify what people really need to know. These should generate information requirements in a standard form that data acquisition people can use.'
When creating and publishing datasets, we are asking more user-centred questions, such as:
- Who needs this data and what do they require it for?
- What problems can this dataset solve?
- How can the data be designed and presented to people such that it best helps meet their requirements?
- Can we use more user-experience methods like user interviews and usability testing when developing datasets?
Mobility datasets
To make these questions practical, we have chosen to focus on mobility data. Datasets about how people are moving through cities during lockdown have been published in the last few months in reaction to the global pandemic. Big companies such as Apple, Google, Facebook and TomTom have all released some mobility data – some more openly than others. This makes it suitable for further research.
Cities and urban areas are now sites of mass data collection with the volumes collected from various types of sensors far eclipsing what used to be possible. Broadly, data about how people move through cities (and it is mainly cities at the moment) – whether that is walking, or using public transport, cars or bikes – should be helpful to anybody who wants to understand the different effects of the ongoing coronavirus pandemic on human movement. As governments seek to loosen the lockdowns that have been in place across the world, this data can be a valuable tool for measuring, analysing and estimating the impacts of different measures.
It would be beneficial, for example, to track whether Boris Johnson’s announcement around easing lockdown and calling for certain trades to return to work (10 May 2020), was matched by an increase in footfall, car usage and public transport use the following day. This data can also be used to estimate the effects of further eases in restrictions.
Another potential use could be city planners. It appears that during the pandemic bike usage has increased, and many are speculating that this boom will continue as long as some social distancing measures are in place. A city planner could analyse data about the number of cyclists, or distances being cycled, in order to assess whether new bike cycling infrastructure, such as bike lanes, are necessary.
At the moment, we’re unsure of how good the data being published is and how useful it is to the people who could benefit from it.
So the purpose of this piece of research is to answer three questions:
- What is the purpose of publishing mobility data?
- Who sets this purpose? Who will find mobility data useful?
- Is the mobility data currently published fit for this purpose?
We are also considering the privacy and data protection implications of different types of mobility data, such as mechanisms to reduce the likelihood of people being re-identified from data and how to protect people's privacy. The final thing that we plan to look into is the need for collaborative research so that the data being collected, used and shared matches the uses and purposes of that data.
We are right at the beginning of this research. In line with our working-in-the-open principles, we will be publishing regular progress updates like this. As well as our focus on dataset search and mobility, we will also be publishing a blog post about models, so watch this space.
If you would like to share any thoughts or suggestions please do get in touch.