Data institutions are organisations that steward data on behalf of others, often towards public, educational or charitable aims. At the Open Data Institute (ODI), we describe ‘stewarding data’ as working to realise the value and limit the harm that data can bring. This includes making important decisions about who has access to data, for what purposes and to whose benefit.
In a previous article on this topic we outlined six vital roles that data institutions play within data ecosystems. We then explored one of those roles in detail by examining how data institutions empower people to play a more active part in stewarding data about themselves.
For this article, we are looking at another stewardship role, which we've defined as 'facilitating safe access to sensitive data'. There is value in increasing access to sensitive data, such as data about health, transport or demographics, but it must be done in ways that respect things like privacy, commercial sensitivity and national security. This post examines the different structures, processes and mechanisms that data institutions rely on to enable access to sensitive data in safe, ethical, equitable ways.
Specifically, we present 18 mechanisms that data institutions use to control:
- who can access the data
- what data can be accessed
- how that data can be accessed
- what that data is used for.
To help explain this, here we’ve introduced a framework for understanding a simplified ‘data-use journey’. We’ve also included a comprehensive case study about UK Biobank which highlights the particular mechanisms used to facilitate safe access to the sensitive data it stewards. We’ve then used this framework and our collection of mechanisms to show how UK Biobank facilitates safe access to sensitive genetic data.
We hope this article will be useful for emerging data institutions, especially those data institutions looking to steward personal data or other forms of sensitive data; other organisations and researchers working on the theory of data stewardship, data sharing and data governance; and organisations working to create an enabling environment for data institutions.
The 'data-use journey'
To better understand how data institutions facilitate safe access to sensitive data, it is important to first understand where data institutions sit within their wider data ecosystems and how they help data get from A to B.
In our work on data institutions, we have found it useful to think in terms of a generic ‘data-use journey’ that starts with the initial collection of data about the world and extends through its eventual use in products and services.
We see data institutions playing crucial roles throughout that journey in order to realise the value of data while limiting harms. We've developed this diagram of a generic data institution to illustrate the five stages of this data-use journey and the general types of activities within each stage. We’re aware, of course, that not all data institutions will follow this exact pattern, but it is a useful framework nonetheless.
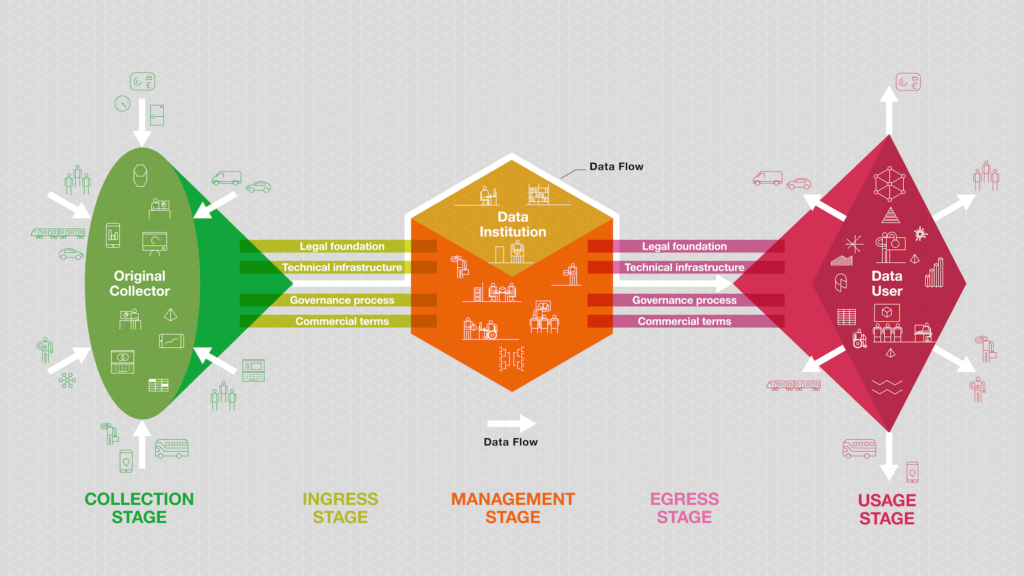
The five stages of the data-use journey are:
- The collection stage: The stage when data about the world is collected, for instance data about people, infrastructure, or commercial markets. This data might be collected by a company or government body through the process of delivering a service; or it might be collected as part of a research study or by organisations gathering information about the world. In some cases this stage is combined with the next stage.
- The ingress stage: The stage in the data use journey when data enters a data institution. What is 'flowing' at this stage is not necessarily the data itself, but the authority to make decisions about that data. In some cases the data might be transferred into the data institution, but in others the data institution might be linking to datasets that continue to be stored elsewhere.
- The management stage: The stage during which the data institution holds, manages and protects the data, primarily through internal processes.
- The egress stage: The stage in the data-use journey when the data institution makes the data it stewards accessible by outside parties. Similarly to the ingress stage, the data that is made accessible might be stored by the data institution itself, or the data institution might be connecting the holders of data with potential users without the data institution being involved in the technical flow of data.
- The usage stage: The stage when people and organisations use the data from the data institution to deliver services, insights, or products for people and organisations in the world.
The real world is obviously more complex than this. For the sake of simplicity, the diagram shows only one source of data, but some data institutions will collect and use data from multiple sources. Furthermore, in the above diagram, data about the world is first collected by an original collector, but many data institutions, including UK Biobank, collect data directly. In these cases, the two stages of ‘collection’ and ‘ingress’ are combined. Finally, data institutions can, and often do, use data themselves to provide services and products.
But while the diagram is a simplification, we think it is a useful one. It helps to break the data-use journey down into manageable stages. It also aids the examination of the various roles performed by data institutions within those stages in order to help data flow across data ecosystems.
Data institutions, like most organisations, are large and complex, so diagramming every interaction they have with actors in their ecosystems or every internal process they have instituted in order to steward data would be time consuming and difficult. Focusing on one stage of the data-use journey, or one role performed by a data institution within that stage, simplifies the task and enables non-relevant details to be excluded.
For instance, we have found it useful to focus attention on how exactly data gets ‘in’ to a data institution (the ingress stage) and how it gets ‘out’ (the egress stage). When conducting research we look to identify and document four things related to how those data flows are enabled:
- The legal foundation, standing, authority or permission by which data institutions are allowed to collect, ingress, egress, or use data.
- The governance or decision-making processes data institutions put in place to govern when and how to ingress or egress data.
- The technical infrastructure data institutions build to support ingress or egress.
- The commercial terms data institutions put in place that enable or restrict ingress or egress.
There may be other important things to document within the ingress and egress stages and we welcome feedback on whether we have missed anything.
Finally, the diagram demonstrates that many of the roles that data institutions perform are relational and involve working with and alongside other people and organisations to realise the value of data while limiting harms. This is particularly true in the ingress and egress stages. Importing, connecting, sharing and accessing data are, after all, almost always relational activities and involve multiple parties. For instance:
- Contracts that help define the legal foundation for ingressing or egressing data are two-sided and in many cases are hammered out in collaboration between the organisation holding data, the data institution and/or the person or organisation seeking access.
- Technical infrastructure for sharing data requires anyone looking to access the data to integrate with the chosen technical implementation and standards.
- Internal decision-making processes about who to share data with and for what purpose often involve reviewing applications submitted to the data institution by those seeking access.
- Commercial terms are naturally multi-sided since transactions involve more than one party.
The four levers for facilitating safe access to sensitive data
For the rest of this article we will be digging into one of the common roles that data institutions perform: 'facilitating safe access to sensitive data'. This role is, for the most part, performed in the ‘management’ and 'egress' stages of the data-use journey. That means that for the sake of this article we focus on the activities within those stages.
When sharing or providing access to sensitive data, data institutions work to ensure the safety of that data through controlling four things:
- Who: controlling who is allowed to access the data they steward
- What: controlling what data those people are allowed to access
- How: controlling how those people are able to access or interact with that data
- What for: controlling what those people are allowed to use that data for.
These are similar to the 'Five Safes', a widely used data management framework that proposes that access to data should be managed in a way that ensures safety in five 'dimensions': projects, people, settings, data and outputs. Controlling these will, in theory, lead to 'safe use' of data. But the Five Safes were developed with a very specific context and use case in mind: to give researchers safe access to data held by government agencies, often through secure virtual research environments and often with the goal of achieving public benefit. At the ODI, we are interested in exploring and diagramming how data institutions facilitate safe access in more diverse use cases through more diverse means and so have expanded upon the Five Safes to develop our own related, but broader framework built around controlling ‘who’, ‘what’, ‘how’, and ‘what for’.
In this article we present 18 different mechanisms, structures and processes that we have identified during our research that data institutions use to facilitate safe access to sensitive data – that is, to control ‘who’, ‘what’, ‘how’ and ‘what for’. These mechanisms are often used in combination and, depending on the use case or sensitivity of the data, more or fewer mechanisms can be used to ensure that data is accessed and used safely.
First we discuss the different mechanisms that data institutions use to control who is allowed to access the data they steward and what they are allowed to use that data for. These tend to go hand in hand and data institutions often use similar mechanisms to control both so we discuss them together. In general, these are legal, commercial and decision-making mechanisms and processes.
We then discuss the different mechanisms that data institutions use to control what form of data people are able to access and how they are able to access it. We discuss these together since they also tend to go hand in hand and data institutions often use similar mechanisms to control both. Generally, these mechanisms and processes are more technical in nature.
Controlling 'who' gets access to data and 'what for'
A common approach to controlling who gets access to data stewarded by a data institution is to restrict access to members of a certain group. This group could be fairly small and circumscribed or more general. Strava Metro, for instance, only works with organisations that ‘plan, own, or maintain active transportation infrastructure’. The UK Data Service, on the other hand, works to provide access to ‘researchers, students and teachers from all sectors’.
Data institutions can also restrict access to those with a certain goal, purpose or intended outputs. Here again, some data institutions set fairly narrow requirements while others are less strict. Social Science One exclusively provides access to a dataset of publically shared Facebook URLs for projects aimed at studying ‘the impact of social media on democracy and elections’. By way of contrast, ADRUK works with projects working more generally to ‘produce valuable insights into UK society’.
Data institutions must authenticate membership in a group, assess a project’s aims and ensure that those who are provided access to sensitive data are capable of and committed to keeping any data they access safe. This is where the mechanisms for controlling 'who' gets access to data and 'what for' come in. As mentioned above, these tend to be legal, commercial and decision-making mechanisms and processes. An organisation might use technology to facilitate applications, coordinate decision making, and authenticate or authorise people after a successful application, but that is not the focus of this section.
We have found it useful to separate this process into three generalised, overlapping steps of application, review, agreement.
Application
The applications required by data institutions to gain access to data range from fairly simple and passive to more complex and involved using more or less of the mechanisms below. For example many open data publishers like Open Targets and UK Data Service make data available without the need to register or apply.
Registration
Some data institutions simply require prospective users to register a few important details before being allowed to access data. Convex, a ‘mobility data exchange’, allows people and non-commercial organisations to access certain types of data for free, only requiring an online registration form with details like name, email address and type of organisation. Other data institutions require potential users to submit details to confirm their identities and bona fides.
Application
Other data institutions ask prospective users to submit more detailed applications that not only request basic details, but require prospective users to lay out their case for why they should be allowed access. Often data institutions even ask for evidence of relevant skills, expertise, competencies or permissions. Clinical Study Data Request (CSDR), for instance, requires potential users to submit a research proposal describing their research background, funding, rationale, approach to statistical analysis, intended outcomes and plans for publication. In addition, prospective users are asked to provide details about the project team, including evidence of relevant education and professional qualifications.
Review
Data institutions tend to use a few basic mechanisms for reviewing, assessing and vetting the registration forms, applications, evidence and research proposals submitted by prospective users. These can be loosely separated into internal review processes and external or independent review processes. Depending on the setup, a data institution might use a combination of internal and external review.
Internal review
Depending on the complexity of the registration or application, internal reviews can be fairly quick and simple or long and complex. On the longer, more complex side of the review spectrum, NHS Digital has a team of case officers that review applications as part of its Data Access Request Service and, when necessary, schedules follow-up appointments with applicants to discuss remaining tasks, capture outstanding details and help applicants to resubmit their modified applications.
External review
External reviews tend to be used to bring in external expertise, views and insights – whether technical or subject-matter expertise or insights from those people or communities who are likely to be most affected by the use of data stewarded by the data institution. For instance, proposals for access to data held by Secure Anonymised Information Linkage (SAIL) Databank ) are reviewed by an independent Information Governance Review Panel composed of ‘representatives from various organisations and sectors’ including medical associations, government departments, health boards and members of the public. Similarly, INSIGHT has set up a Data Trust Advisory Board whose role is to scrutinise users’ requests for access and ensure that ‘the views of patients and the public are represented where it matters – at the heart of the decision-making process’.
Audit
Some data institutions perform audits or checks of a prospective user's facilities or competencies as part of the review process. NHS Digital, for example, checks that potential users have appropriate safeguards in place so that they can be trusted to ‘store and handle the data safely and securely’. Even after terms have been agreed and access to data has been arranged, some data institutions continue to monitor users to ensure that they are abiding by the terms of their agreement. Where necessary, NHS Digital carries out post-audit reviews to ‘ensure that organisations abide by the terms and conditions set by NHS Digital and data is kept safe and secure.’
Training
Before granting approval, some data institutions require prospective users to complete training courses or provide proof of accreditation or certification in relevant areas. These training courses can be specific to the data institution or more generalised, and are often aimed at ensuring prospective users understand the importance of using data safely, ethically and responsibly and are equipped with the requisite skills to do so. The UK Data Service, for instance, requires potential users to complete the ‘Safe Researcher Training’ course which concludes with a mandatory online assessment. SAIL, on the other hand, accepts a range of recognised information governance courses completed in the last three years.
Agreement
Once the applications have been reviewed and the prospective users granted approval, the next general step is to get everything agreed and confirmed.
Contract
Contracts, data sharing agreements and licences can be used to define – in legally binding terms – who can access the data stewarded by the data institution and what they are allowed to use that data for, and sometimes even specify what they can access and how. They are some of the main tools for open data publishers to ensure that the data they publish is used safely and responsibly.
Many data institutions, such as CSDR have standard templates for data sharing agreements. Some organisations offer different types of contracts depending on who is accessing the data or what they plan to do with that data. OpenCorporates, for instance, has different contracts and restrictions on use for people working on projects that will ‘contribute back to the open-data community’ versus those working on ‘commercial or proprietary applications.’
Pricing
Data institutions can also use commercial terms and pricing models to control access to data. These terms can be used to control not only who accesses the data and for what purpose, but also the type of data and how regularly it can be accessed. This is common to the marketplace approach of organisations like Convex, Dawex and Harbr but is also used by other data institutions. OpenCorporates, for instance, offers a range of different commercial plans with different access permissions. Some organisations, like Clinical Practice Research Datalink charge a fee for accessing data only to ‘recoup the cost of delivering research services’.
How UK Biobank controls who gets access to data and what for
To see these mechanisms in context, please refer to the diagram at the bottom of this article or download the diagram here.
Note: This diagram of how UK Biobank controls who gets access to data and what for, is based on its procedures as of July 2021. However it plans to change over to a new system by 2022.
UK Biobank provides access to ‘bona fide researchers’ from academic institutions, charities, government bodies or commercial companies, so long as their research is health-related and ‘in the public interest’. UK Biobank uses a collection of legal, commercial and decision-making mechanisms to ensure that the data it stewards is used safely by those provided access. The process follows this general pattern:
Submit registration form (via the Access Management System) including details such as name, email address, phone number, CV, PubMed references, Any complaints over previous three years and details of place of work.
Agree to terms and conditions for use of Access Management System.
Internal review of registration form conducted by ‘UK Biobank Access Management Team’ to confirm details and applicant’s status as a ‘bona fide researcher’.
Submit application form for access to data (via the Access Management System) including details such as research questions(s), background, scientific rationale, intended methods, type and size of datasets needed, ‘lay summary’ and expected value or impact of research.
Pay Access Fee(s) to cover ‘the incremental costs of servicing an access application’ and supplying required data.
Internal review of application form conducted by Access Management Team and, for research involving recontact with participants or ‘potentially contentious research’, additional review by UK Biobank Access Sub Committee.
Sign and submit the Material Transfer Agreement (via the Access Management System)
Controlling 'what' data people get access to and 'how'
In addition to controlling who is allowed to access the data they steward and what they are allowed to use that data for, data institutions can control what form of data people are able to access and how they are able to access it. We discuss these together here because they are often intertwined and data institutions tend to use similar mechanisms to control both. Whereas the previous section was primarily about legal, commercial and decision-making mechanisms, the mechanisms used to control 'what' and 'how' are generally more technical in nature. Just as the mechanisms in the previous section can be used in combination with others, the technical mechanisms presented here can be combined where necessary.
Minimising sensitivity
Much of the data that data institutions steward contains some degree of sensitive information. Even data commonly seen as non-sensitive may contain information that could reveal sensitive details if combined with other data. But ‘sensitive’ is difficult to define. Different people, organisations and companies will have different views on what is sensitive within a dataset. And yet, there is also value and utility in sensitive datasets so different people, organisations and companies will have different views on how much risk is acceptable when sharing sensitive data.
One of the main ways that data institutions seek to strike the right balance between risk and utility is by reducing how much sensitive data the users are allowed to access or transfer to other systems.
Modified data
When data institutions modify data to limit sensitivity or the risk of re-identification they often do so by removing sensitive information and/or adding noise to mask that information. There are so many different technical processes for limiting sensitivity that we will not attempt to provide a comprehensive review or differentiate them here. For a detailed description see the Information Commissioner’s Office anonymisation code of practice. The important thing, for our purposes, is whether the data has undergone some form of technical or statistical process to limit the amount of sensitive or identifiable information contained within it. If it has, then we will refer to it as modified; if it hasn't, then we'll refer to it as unmodified.
INSIGHT, for example, works to ensure that ‘only safe, anonymised data’ is made available to researchers, by which they mean that ‘any identifying information (such as name or address) is removed before researchers can access it.’ In contrast, NHS Digital’s coronavirus dashboard utilises 'disclosure control', for example changing ‘small number counts (where the true value is less than 3) are replaced with ‘< 3. Social Science One has instead used a suite of technologies referred to as differential privacy to ‘introduce statistical noise and censoring into datasets (or in results from those datasets) in order to prevent reidentification of any given individual who may be represented in the data.’
Unmodified data
For our purposes, data that is unmodified in this context has not undergone a process aimed at removing sensitive information or adding statistical noise. This does not necessarily mean the data is 'untouched', as some management, cleaning, or curating is to be expected. Sometimes, the data that a data institution stewards contains very little sensitive information to begin with and can be published openly with little modification.
For data with higher levels of sensitive information, leaving sensitive information in that dataset can often increase the potential value for users, but it also increases the risks. Because of this, data institutions that facilitate access to unmodified datasets will often do so only for certain uses and where they can be confident that the access conditions they have put in place are enough to limit the risk of re-identification. The Driver and Vehicle Licensing Agency (DVLA), for instance, provides certain companies access to a modified ‘anonymised data set’ which includes the make and model of a vehicle and its partial postcode. This data is most often used for advertising purposes. However organisations that offer vehicle checking services to the public need access to more granular detail than this anonymised dataset. For these companies, DVLA provides access to the unmodified ‘bulk data set’ which contains 47 different information fields about a vehicle.
Insights
Rather than provide access to modified or unmodified data, some data institutions provide users with access to insights, trends or signals drawn from the data. This has the advantage of providing actionable insights for users while limiting the risk of re-identification since users never directly access the data. This task of analysing datasets to derive insights and share these insights with interested parties is one of the six roles of data institutions discussed in our previous article. HiLo Maritime Risk Management, for example, has access to the ‘full internal data of shipping companies’ and uses ‘big data predictive analytics'’ to provide subscribers with ‘regular, easy to understand risk analysis to help them prevent maritime incidents’. Similarly, Driver’s Seat Cooperative works to ‘pool and analyze driving data to deliver unique insights’ that help their customers ‘understand shared mobility and logistics in their community’. Interestingly, whereas HiLo currently provides insights only back to members that contribute data, Driver’s Seat provides insights to contributing members as well as to local authorities and transport organisations on a paid basis.
Individual and aggregate datasets
Data institutions can also control whether data users get access to individual datasets or aggregate datasets – for example datasets about multiple people at a population or geographic level. The process of aggregating datasets is in itself a way of modifying data to limit sensitivity. Often this is related to data about people, but it can also be used to limit access to sensitive information about physical assets, entities or business processes. Data institutions like Driver's Seat and Strava Metro give local authorities and transportation organisations access to data about their members at an aggregate, population level. Data institutions like UK Biobank can give access to aggregate datasets as well as individual-level data, depending on the requirements of the user. In the case of UK Biobank, the individual-level data is modified through other means so that users are provided access to anonymised, individual-level data.
Technical access
Data institutions can also control ‘how’ people get access to data, often through utilising different technologies to mediate, to varying degrees, how users are able to interact with the data. Usually, the more sensitive the data being made available, the more mediated the form of access. We have arranged these different technical approaches from least amount of mediation and control through to the most, but there are undoubtedly overlaps and these technologies can be, and often are, used in combination.
Direct transfer
Some data institutions make data available to users through direct transfer or download. This can be a one-off download or a regular, recurring transfer. One of the benefits of this approach is that it enables users to process, analyse and use the data directly; one of the main limitations of this approach is that once the data institution has transferred the data to the user, they have fewer mechanisms for ensuring that the data is used safely and in line with the agreed terms. As such, direct transfer is often used for data with lower levels of sensitive information, or in situations where the data institution is confident the other control mechanisms they have put in place are enough to ensure that the user will use the data safely. The Department for Environment, Food and Rural Affairs, for instance, makes a wide range of datasets available ‘for download in commonly used formats’ through its Data Services Platform. Open data organisations like 360Giving also make data available for direct download.
Data stream
Suggestion: Data streams and APIs expose a formatted version of data over an internet connection, which is usually intended to be processed regularly, usually in an automated way by software such as a user’s app, website or script. Because the data is selected and formatted before it is shared the data fields can be modified, filtered or otherwise restricted depending on what is known about the user and the query parameters they supply. The underlying dataset in its entirety does not need to be shared with the user.
Many organisations that provide direct transfer of certain datasets also provide data streams or APIs to enable access to different data, for different purposes. Companies House, for instance, allows users to download certain datasets directly but is also currently developing an API. Similarly, OpenCorporates provides users with API access to the data it stewards. However, as explained on its website, for some users ‘only access to complete datasets will do’ since directly downloading data offers benefits such as ‘detailed analysis [and] integration with internal or third-party data’.
Interface
Some data institutions make data available via some form of web interface, allowing users to run their own user-defined queries against a restricted subset of data fields. Users define their parameters or submit their query and results are delivered on screen, sent by email, or made available for download.
Some interfaces, like Strava Global Heatmap and NHS Digital’s Activity in NHS hospitals dashboard include built-in visualisation tools, and some, like Uber Movement and the NHS Pathways dashboard, enable direct download of datasets after users have defined their parameters. In many cases these interfaces are openly and freely accessible. They are also often used in combination with the two forms of access described above. OpenTargets is a good example. Its platform is a ‘freely available resource’ and data is available ‘through an intuitive user interface, an API, and data downloads’.
Secure virtual research environment
Some data institutions require users to access data in a secure, online research environment where they can more closely monitor and control what data users interact with and how. These are similar to web interfaces and dashboards in that they enable users to interact with data and include built-in visualisation tools, but many, like the Data Access Environment run by NHS Digital, include built-in analytical tools and software. Some, like the Secure e-Research Platform used by SAIL, even provide users with access to powerful cloud computing and enable data institutions to monitor or audit a researcher's use of data.
For data institutions, one of the main benefits of secure virtual research environments is that the data they steward does not need to be transferred beyond their control. Indeed, according to NHS Digital, its Data Access Environment is useful because it ‘reduces the need for [data] to leave NHS Digital’. Once users have finished analysing and visualising the data, many secure virtual research environments like the Clinical Trial Data Transparency System used by CSDR (and hosted by SAS), also include features designed to limit the risk of re-identification by controlling what types of data or insights researchers are able to export or take with them as outputs.
Secure physical research environment
Some data institutions provide access to sensitive data via secure physical research environments. These are similar to secure virtual research environments but are physical locations where researchers can access datasets directly, often via a secure connection to a local network. Data institutions tend to use these physical environments to provide access to extremely sensitive data and/or for datasets that are simply too large to be accessed through a virtual environment. For instance, users are able to access data held by the UK Data Service through its Secure Lab either through their own computers or at a UK Data Service ‘Safe Room’, ‘depending on how restrictive (and sensitive) the data are’.
How UK Biobank controls what data people get access to and how
To see these mechanisms in context, please refer to the diagram at the bottom of this article or download the diagram here.
Note: This diagram of how UK Biobank controls who gets access to data and what for, is based on its procedures as of July 2021. However it plans to change over to a new system by 2022. This new system will utilise a virtual research environment similar to the ones currently used by NHS Digital and Sail Databank.
UK Biobank uses a collection of different technical mechanisms to ensure that the data they steward is used safely by those provided access. The process follows this general pattern:
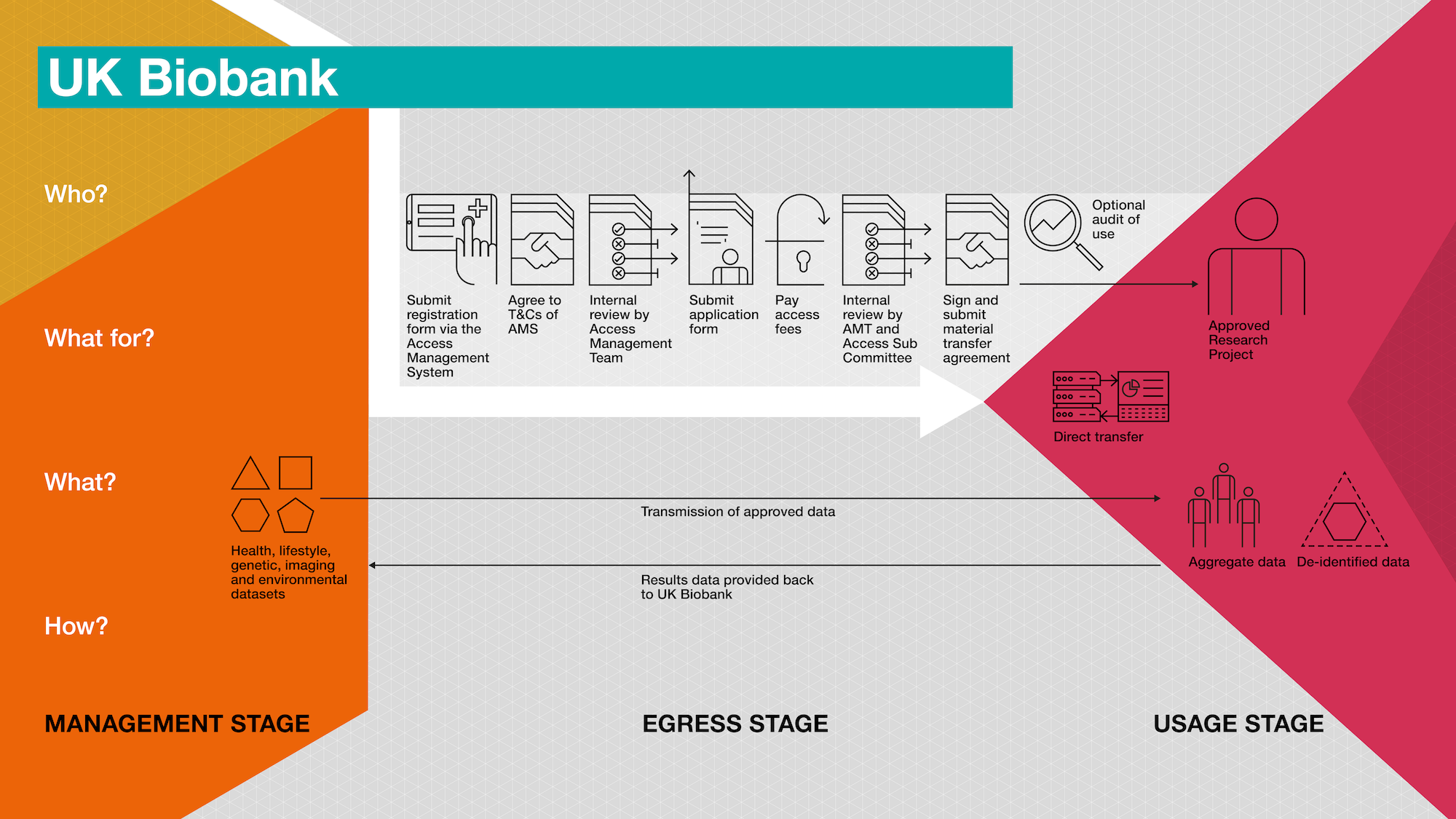
Looking ahead
We think that breaking down real-world data institutions into structures and component parts in the way we've done here is useful because it helps to isolate the essential things that help data institutions perform the role of facilitating safe access to sensitive data.
For people designing and building data institutions, we hope this article and the approach outlined within it helps clarify the different design options available when seeking to facilitate safe access to sensitive data. This article has focused on the egress stage, but in future work we would like to examine the different design options available to data institutions in other parts of the data use journey – in particular the ingress and management stages.
For policymakers, funders and other researchers, we intend for this article to help compare and contrast data institutions and better understand how to create an enabling environment for them.
In the coming months, we will continue to add data institutions to a Data Institutions Register that we are building, and welcome help to do so. There is a big world out there and we know we haven’t come close to identifying many of the different data institutions in it.
We would love to work through this process with interested people and organisations, so please get in touch if you are interested in collaborating. We also welcome feedback on what we could do differently and things we have missed.