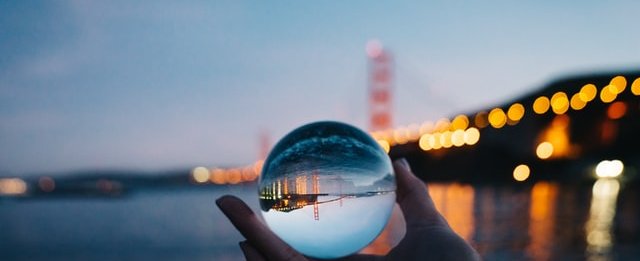
By Joe Paul, a science communications student at Imperial College London. Joe has been working with the ODI over the summer and autumn of 2020.
------
As computational modelling becomes more pervasive and influential in informing public policy, the importance of transparency for public accountability also grows. Increased sharing can also bring enormous benefits on a much smaller scale, allowing knowledge and skills to spread between teams and organisations. This article explores the opportunities that lie in open modelling.
On 23 March 2020, the UK government announced a strict nationwide lockdown in response to the Covid-19 pandemic, restricting personal freedoms to an extent unseen for a generation. This decision was supposedly strongly influenced by a computer model written by one person 13 years before the pandemic, a model comprised of ‘thousands of lines of undocumented [code]’ that was not available for public scrutiny.
Since then, the model’s code has been made public, allowing anyone to experiment, improve upon and flag problems, as well as opening up the expert advice that influenced the UK government’s decisions to closer scrutiny. This is an example of the benefits of increased sharing in computer modelling. But what even is an open model?
Why should we make our models more open?
Computational models are simplified imitations of the real world which allow us to explore situations that are impractical or impossible to explore otherwise. They are used in diverse situations, from informing homelessness policy to predicting leaks in water supply systems or helping farmers choose which crops to sow.
The benefits and limits of open modelling vary from context to context – for example, it may not be possible to share the full input data for a model used in medical research due to privacy concerns. Generally, however, there are benefits to be gained from making a model more open, even just increased within an organisation, as explained below:
- Improvements and error finding: When outsiders gain access to a model, they are able to test, modify, reapply or even improve upon a model. Opening a model to wider review permits the chance that someone will spot a bug in the code, will see systematic oversight in the data being used, or will see other areas for improvement.
- Adaptations: Adapting and applying modelling techniques to different contexts allows modelling teams from different organisations and disciplines to learn new skills. The UK government’s Blackett review on computational modelling argues that this cross-pollination of ideas is hugely beneficial to the collective improvement of modelling skills across a healthy broader modelling culture.
- Improved trust: Greater transparency leads to improved trust between organisations, consumers and members of the public. If someone can see the reasoning and evidence that goes into a decision, there is a higher chance that the decision will be trusted.
- Democratic accountability: The increasing use of computer models to inform public policy means that there is an aspect of democratic accountability to consider. It is important that models that are used to inform decisions made by elected officials and taxpayer-funded authorities that have wide-reaching consequences are made available for public scrutiny, explanations and further development. For example, see Jeni Tennison’s explainer on a much-criticised algorithm used to grade A-level and GCSE students in England, in the absence of exams.
- Greater interpretability: When a model is open to more eyes, more ways of explaining its function may emerge, perhaps making it easier to explain and understand, and ultimately encouraging its use within an organisation.
How can a model be made more open?
Although giving outsiders a copy of the code is a necessary first step in making a model more ‘open’, more work is usually required to make the model understandable and useful to those who have not worked with it, and so reap the full benefits of increased sharing.
- Code: the code may have to be written and organised in such a way that it meets certain conventions or standards that are established within a particular field, to make it more interpretable to those that did not write it.
- Documentation: the model will likely need its documentation to be expanded – information about the purpose and scope of the model, as well as details about the input and output data, is vital in understanding how to use and interpret it. This extra documentation may consist of, for example, regular work notes as it is developed, operating manuals, or a published research paper.
- Data: It may be beneficial to publish both input and output data along with the model, along with explanations of how to interpret both. Published data should also adhere to conventions and standards, for example, the FAIR principles (Findable, Accessible, Interoperable, Reusable). The ODI works to make data publishing easier and more impactful, and has created guides on data publishing standards and data licensing.
- Input Data: providing access to the input data used with the model will allow others to see how the model was used to reach certain conclusions. Although some data has limits as to how public it can be made, it is still important to give details about the sources and types of input data to ensure the model can be properly understood.
- Output Data: The output data is data generated by the model, and may be very different from the input data. For example, the input data for a model of a water supply system may come from sensors detecting water pressure or noise, while the output data will give predictions for the locations of leaks. It is therefore very important to give guidance for any users of the model as to how to interpret data they produce by running the model.
Many of the steps in making a model more open are also general good practice for model-making. If a model is able to be published openly, chances are it is more interpretable by other members of the same organisation, and will be more easily enhanced or modified in future.
Further guidance
The ODI provides guides and toolkits on how to share your work and data more generally, and here is a specific guide on how to make Covid-19 models and data more open.