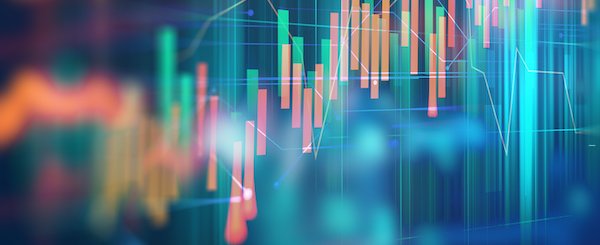
As the UK government enforced national lockdowns to counter Covid-19 from March 2020, it knew that these measures would also severely impact the economy. Responding to the need for data about the economic impact, the Office for National Statistics (ONS) released the first weekly ‘Coronavirus, the UK economy and society, faster indicators’ report two weeks after the first lockdown was announced on 23 March 2020, and continued to publish weekly updates, providing data on areas such as business impacts, high-demand products, workforce and supply chains.
Over the last few years, the ONS has done much work in trialing faster methods and data sources to measure the economy. This was initially recommended in 2016 in the Independent Review of UK Economic Statistics , and in 2019 the Data Science Campus first trialled indicators that used sources such as shipping data and value added tax (VAT) returns. This work was ramped up as the Covid-19 pandemic hit, with the ONS publishing real-time weekly indicators on the UK economy – using data sources such as retail footfall, credit card data and flight information.
To learn more about these real-time indicators, we interviewed Andrew Walton and Issie Davies at the ONS.
How are indicators created?
Deciding which indicators will be used to demonstrate the state of the economy is hard – and sometimes a case of prioritising existing indicators rather than identifying new ones.
When approaching the task, Davies explains that they started with long wish lists, and then whittled them down according to relevance to the economy. The team was acutely aware of the changing priorities and demands dictated by the fast-paced real-time economic factors as the government responded to the pandemic and made decisions across multiple sectors. A good example of data which rapidly became important is that relating to aeroplane flights, which became paramount when international travel started again after restrictions were lifted.
Davies adds: “We try to be as reactive to the scenario of the time, in that moment. And if that means our priorities shift to get what’s most topical out there then we certainly do that.
“But I'd say that’s more a reflection of what indicators do or don’t go in, more than anything else.”
We also meet regularly with a cross-government group who play a key part in the quality assurance of our data
Acquiring the data
It’s helpful to understand the infrastructure behind the data; who supplies it; and how the ONS extracts and receives it. Davies notes “some indicators are delivered to us while others are collected by the team” and lists a host of different methods: direct delivery from suppliers, such as the Bank of England CHAPS data, extraction via APIs, data scraping of publicly available sources. The ONS may use data agreements, open data, dashboards, survey results, depend upon agreed regular deliveries or look to specific external sources.
Transforming data
As a ‘data intermediary’ – a body which sits between data providers, data users and other stakeholders in the sharing and use of data – the team will sometimes apply transformations to the data, but in other cases, this is not necessary. We asked about the range of work involved.
Davies agrees it is variable. “It depends on what sort of infracture we have underneath each indicator as well. So for many of the indicators developed by the Data Science Campus, for example shipping and traffic, these will take far more time to process because we’ve developed those pipelines ourselves and it's something we’re producing from beginning to end. In contrast to something like CHAPS, where we essentially get delivered the publishable series.”
We are looking now at a model of fortnightly major releases with full commentary but more frequent data drops in between
Who uses the indicators?
Davies noted that the audience is broad: ranging from ONS analysts, government, academics, and the private sector, through to the media and the general public. “We also meet regularly with a cross-government group who play a key part in the quality assurance of our data and make requests based on the emerging requirements,” she says. “I don't think there’s a week that goes by where we don’t see our data or one of our key headlines featured somewhere either on the news or on a published article somewhere”.
How fast is fast enough?
The real-time indicators bulletins are released on a weekly basis. But could this data be released even more frequently?
Walton says that they are now working towards a model where data will be released more frequently, as the balance is altered between the full (5,000-word) bulletin and the speed of releasing datasets. “So we are looking now at a model of fortnightly major releases with full commentary but more frequent data drops in between,” he says.
Testing indicators
New sources of data can provide useful information but they could also be unhelpful. We asked if they have a testing system in place to check if the indicators contain reliable signals about the economy.
ONS is linked to the Economic Statistics Centre of Excellence and Walton notes that they work with these and other academics to test which indicators are adding value and which are ‘just noise’ when it comes to making predictions about movement in the economy. And he adds that this ongoing resource makes new indicators evidence-led as far as possible: “When we’re considering a new indicator we’re using some of that information to work out, in a more structured manner, whether it is an indicator worth pursuing”.
Lessons for faster data
Looking beyond the economy, and at any area looking to harness new data ecosystems for faster measurements, we can apply the following lessons from the ONS’s work:
- Consider how timescales affect data use – The pace of data shapes the pace at which people can make decisions. This can be timescales the data measures (for example weekly, hourly); how often it's released; or how long it takes to publish.
- Data should be as fast as users need it, no more – Faster data is only as helpful as the speed at which people want to make decisions. Faster data may provide more noise than signal.
- New data sources may need work to extract value – People trialing faster data sources need to be prepared to put in the work to extract valuable information.
- Set up a testing framework for this new data source – Big and fast datasets may be impressive but they need to be regularly tested to see if they actually provide useful signals.