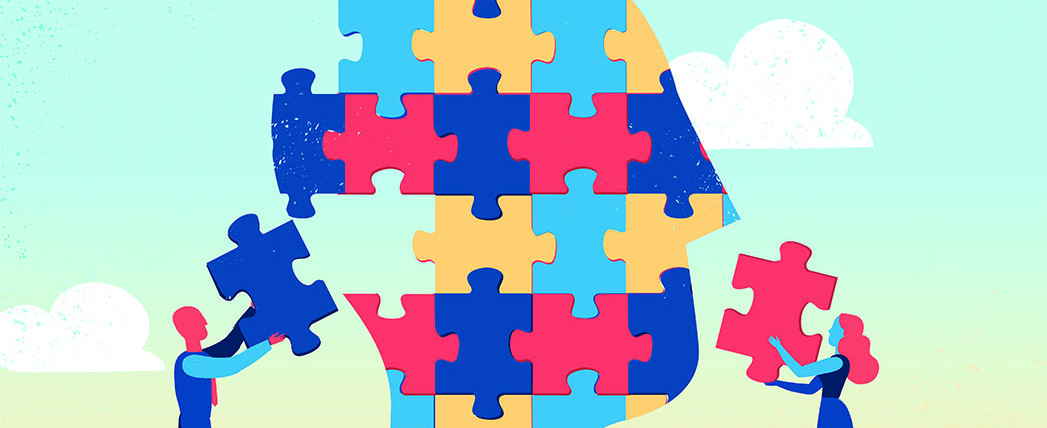
When publishing personal data, is anonymising the data foolproof? Does it absolve you of all responsibility under the data protection regulation? Can people be re-identified? As part of a research project, we are exploring these questions, starting with researching the challenges faced by organisations who might need to anonymise and publish personal data.
Using data effectively – and ethically – can enable innovation, create more efficient services and products, and fuel economic growth and productivity. And for the greatest societal and economic benefits, data should be open as possible while protecting people’s privacy.
When sharing or opening personal data, protecting privacy is critical. While anonymisation in theory protects privacy, the risk of the re-identifying (previously anonymised) data is a key issue to consider.
While the General Data Protection Regulation (GDPR) cites anonymisation as an effective way to manage compliance (‘GDPR does not apply to personal data that has been anonymised’) the techniques involved seem to be laborious and variable depending on the domain, data structure and content.
At the ODI, we are running a project which aims to help organisations reduce the risks of re-identification when sharing or opening data, by providing tools and knowledge to help identify and mitigate those risks.
To help us develop these tools we undertook a round of exploratory, qualitative, small-scale user research to explore organisations’ current practices and perceptions, and to identify any challenges and barriers.
User research: how do organisations understand and manage the risk of re-identification?
As part of the user research, we interviewed six data governance and anonymisation experts from across the public (one participant) and private sectors (five participants). - We selected a small number of interviewees as part of this first exploratory round where we could get a sense of whether the risk of re-identification is perceived as an issue within different organisations.
We also referenced the ODI’s Data Spectrum, which describes the range of data ‘openness’, from closed, to shared, to open - although in this research we only focused on open and shared data.
We wanted to identify whether the point on the spectrum affects the perception an organisation has about the risks of re-identification.
How do organisation understand personal data?
We started by exploring the definition of personal data. Interviewees from both sectors defined personal/sensitive data as ‘data that can identify someone’.
We also wanted to know about organisations’ awareness of the legal requirements around personal data, with a particular focus on GDPR. Both the private and public sectors were familiar with the legal framework in relation to personal data. Interviewees generally had a good understanding of GDPR, and perceived that although ensuring compliance could be daunting, it was also a useful tool to prompt conversations about personal data and the way it should be managed.
How are the risks perceived?
Dealing with personal data comes with different risks. We wanted to know how organisations perceive the risk of a person within a dataset being re-identified.
Interviewees identified various risks around opening/sharing and anonymising data. These ranged from the usability of the data, through to concerns around social scoring.
The range of concerns included:
- Usefulness
- Is it going to be useful if I anonymise data?
- Will I lose the value of the data?
- How will that impact my business value?
- How valuable is the data I hold?
- Data use
- Who is going to use it?
- How/when is going to be used?
- Philosophical perspective
- Users/individuals perspective should be taken into account
- Social impact:
- Some interviewees from the private sectors who are familiar with data science consider the main risk around reidentification to be the social impact, with concerns about the data being used for social scoring or to manipulate behaviour
- A concern was also expressed about transparency, data retention and AI. Specifically, what happens when machine-learning algorithms have been developed, but then the source data is deleted: will the algorithm also be updated, or continue to make decisions based on previous data? And will there be transparency around data sources?
Some of this key insights come from statements such as : “I would say the main risks are not being transparent with the data subjects or the participant” or as another participant noted talking about deep learning models: “All of a sudden you can take mundane data and get deeply personal insights into the mental makeup of customers, and then you can modify their behaviour.”
How do organisations attempt to mitigate those risks?
One of the aims of this research was to understand how organisations have attempted to manage those risks, and to explore current approaches. We identified interviewees used to consider initially:
- How does an algorithm work and how that could increase risk?
- Is my organisation using personal data?
- How does my sector feel about this?
- Am I working with data that needs to be anonymised?
- Is all the collected data needed?
- How linkable are my datasets?
Organisations described different methods of risk mitigation across the stages of the data lifecycle (eg storing, accessing, using and sharing data). While the key mitigation was to follow GDPR guidelines and use legal expertise, interviewees also cited transparency and ethical approaches as ways to mitigate the risks. One participant noted “We have restricted access to all data we process within our organisation to ensure only those who absolutely need to have restricted monitored access” or as another described: “We have a Privacy Impact Assessment processes [...] we have a team of people who manage that and they would work with the data to make sure that is continually monitored and any potential breaches are kind of managed.”
What about anonymisation?
Although there was good awareness of the concept, the opinions about its usefulness differed depending on the background of participants.
There were two key viewpoints: those that define personal or sensitive data as specific data points (such as demographic, ID, postcode) find anonymisation technique effective; those that understand personal data/sensitive in a broader way (more that just IDs or postcodes) see anonymisation as a technique with limitations.
We found that the main constraints and limitations when choosing anonymisation as a risk management method were around cost and technology.
- Tech innovation
- How are organisations dealing with the advances in technology and machine learning techniques?
- If an algorithm has been trained, the information derived from the data is still there even if the data is removed. How do we mitigate that risk?
- Cost
- What are the cost implications of anonymising vs not anonymising?
Next steps
This first round of user research has helped us confirm that re-identification is an issue when it comes to opening or sharing data, and organisations are aware they must mitigate those risks.
The research showed the challenges and constraints organisations face when opening or sharing personal data, in particular in relation to anonymisation methods. We are looking forward to using the research to inform the design of tools and guides to help organisations manage the risk of personal data re-identification.