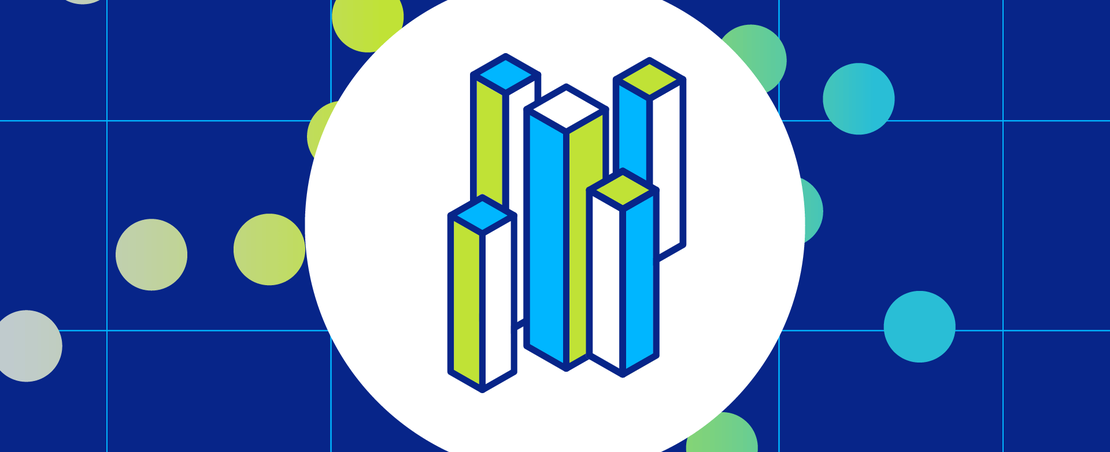
After decades of increased data collection, there is a greater demand for data privacy now more than ever. Privacy enhancing technologies (PETs) could facilitate greater sharing of sensitive data in ways that ensure data protection and privacy. Federated learning is one technology that is approaching a stage of relative maturity, in terms of awareness and practical application. It can be used to train machine learning (ML) models in a distributed manner, while keeping raw sensitive data safe in its original locations. In this report, we provide a comprehensive account of federated learning. We cover its primary distinguishing characteristics and the promise that it holds both for commercial use cases (within a single company or for collaboration across multiple companies) and organisations interested in using federated learning for public, charitable and educational purposes.
We think this guidance will be particularly useful to:
- Organisations that hold sensitive data
- Ecosystems of organisations that hold sensitive data
- Policymakers, funders and other enabling actors supporting data use for social impact
In this report we address the following questions:
- What is the current status of federated learning technology, including key opportunities, real-world test cases and obstacles to adoption?
- What are the unique value drivers of federated learning that make it suitable for some use cases and less suitable for others?
- What are the key technical and organisational considerations that need to be taken into account when deploying federated learning?
Read the report
Federated learning - an introduction