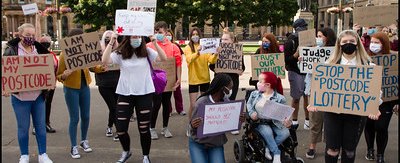
Algorithms increasingly influence our digital lives – from the search results we see to the shows that Netflix recommends to us – but they are also encroaching into our real lives, and being used to make decisions that affect our futures.
This has been brought into sharp relief by the recent furore around the use of algorithms in the grading of GCSE, AS, A-level and BTEC results in the UK, which couldn’t be examined this year due to the Covid-19 crisis. Grade predictions provided by teachers tend to lean in favour of students by giving them the benefit of the doubt, so Ofqual and other qualifications regulators attempted to adjust them to bring them into line with prior results.
But the results were traumatic, apparently favouring private school pupils and with some students finding their A level grades were markedly different from their predicted results and losing hoped-for university places. People took to the streets with “Fuck the Algorithm” chants. The government and Ofqual went from backing their approach as the least worst solution, and insisting that grades needed to stay in line with prior results, to abandoning it and guaranteeing students could use whichever was better of their teacher or algorithm prediction.
For those of us who work in data ethics and responsible AI, this experience will join a litany of touchstone examples – care.data, DeepMind/Royal Free, and Facebook/Cambridge Analytica – that illustrate how public trust in the use of data, algorithms and AI can be irreparably damaged.
We asked experts in data ethics and responsible AI to describe where they think Ofqual and other qualifications regulators went wrong, and what organisations facing similar situations in the future should learn from their experience. Here are their responses.
Carly Kind, Ada Lovelace Institute
The shambolic unveiling of the A-level results algorithm has done immeasurable harm to trust and confidence in the use of algorithmic systems by public bodies. Images of disadvantaged students directing their legitimate protest at an algorithm will not fade quickly in people’s minds.
The question of who is making judgements is important to the public, and trust in decision-makers can be fragile
How should the government go about rebuilding trust in data-driven decision-making? Our recent report Confidence in a crisis? analysed the public’s response to another recent instance of public-sector technology deployment, the NHS contact-tracing app.
Over three weeks of deliberation with 30 members of the public, we distilled four conditions for trustworthy technology deployment that are as applicable to public-sector algorithms as they are to public-sector apps:
- Provide the public with a transparent evidence base. The public would like to see clear and accessible evidence on whether technologies (and algorithms) are effective and accurate, and under what conditions.
- Offer independent assessment and review. The question of who is making judgements is important to the public, and trust in decision-makers can be fragile. Trust can be strengthened with the inclusion of independent reviewers, assessors and evaluators to shape the development and use of algorithmic systems.
- Clarify boundaries on data use, rights and responsibilities. Wanting independent oversight doesn’t negate the desire for clarity on users’ data rights. It must be easy to discover what data would be held, by whom, for what purpose and for how long - and to justify those decisions.
- Proactively address the needs of, and risks relating to, vulnerable groups. People want reassurance that a system is fair for everyone, and that benefits will be distributed equally, not according to a postcode lottery.
The failure of the A-level algorithm highlights the need for a more transparent, accountable and inclusive process in the deployment of algorithms, to earn back public trust.
Jeni Tennison, Open Data Institute
Providing pupils with grades without exam results is an extraordinary ask in extraordinary times. It’s unsurprising that Ofqual and other qualifications regulators turned to data and algorithms to do so given the growing trend in the public sector that has only accelerated under the current government.
But the mess that has unfolded illustrates the limits of data-driven approaches. Data is frequently biased, simplified, and frequently poor quality. That’s hard enough to deal with when data is being used to spot trends for groups, but things can go badly awry when it’s being used to make decisions about individual people’s lives, particularly those that have binary yes/no, pass/fail implications.
...the mess that has unfolded illustrates the limits of data-driven approaches
Once the decision to use an algorithm was made, the approach taken when designing it, and the process around its use, becomes really important. Many choices were made along the way, including about complex, subjective things like the definition of fairness. Reading Ofqual’s technical report, it’s clear they were aware of these challenges, and tried to counter them by doing many of the things experts on algorithmic accountability would recommend, but not to the extent they should have:
- Transparency and engagement: While Ofqual did consult and made some information about the algorithm public, they were only transparent about the details on A-level results day, and even then without code. They should have been open with and engaged extensively throughout the process with education experts, data scientists, and teacher, parent and student groups. This may have alerted them to wider problems earlier, and could have created a community to explore alternatives, and help explain and support any design decisions.
- Detecting and redressing errors: Any algorithm produces errors; the challenge is to build a surrounding process that identifies and corrects them quickly and with the minimum long-term impact on those affected. The Northern Ireland regulator examined places where algorithmically generated grades diverged substantially from teacher assessments, to proactively understand and correct them before students were affected by them. But in general the confusion, uncertainty and cost of the appeals process created additional stress and harms for students, parents and teachers.
- Monitoring and evaluation: Ofqual’s technical report indicates that they did examine the overall impact of the algorithm on the distribution of grades and equality of impact on students from different genders, ethnicities, socioeconomic backgrounds and so forth. However, this did not detect the problems favouring private-school pupils that were picked up in the press when results were finally issued. It would be good to examine how to carry out these assessments better next time – perhaps using scenario-based assessments to complement statistical analyses.
More broadly, though, the larger lesson is around choosing the right balance between algorithmic and human decision making. Ofqual and other qualifications regulators could have chosen an approach that emphasised helping teachers to work together to standardise grade predictions, rather than placing the majority of the burden of that standardisation on an algorithm. We should not always leap to algorithmic solutions. No matter how well done, they are not magic bullets.
Rachel Coldicutt, independent technologist, formerly Doteveryone
Exams are contentious and unfair for all sorts of reasons that have nothing to do with technology. But most people know how they work, and there is – for better or worse – a broad acceptance of the risk factors involved.
This embarrassing litany of process failure has made thousands of teenagers determined to 'Fuck the algorithm', and frankly – who can blame them?
Automated decisions, meanwhile, concentrate and bring high-definition clarity to unfairness. Bias, prejudice and unfair advantages that are hidden in daily life are given focus and coherence when analysed at speed and at scale.
Meanwhile, a set of other complex systems – including university admissions and job offers – depend on A-level results being mostly uncontested and appearing on time.
And results day is, at the best of times, fraught and emotional. Even more so during a pandemic and a recession, when many certainties have been taken away. It’s the beginning of the future for many 18-year-olds, and a generally hopeful moment for many others.
Dropping an algorithm into an already unfair system with time-sensitive dependencies was always going to require care and diligence, as well as frank and clear public communication – but unfortunately, none of those were present.
Democracy is not a black box. Data-driven government must be accompanied by transparency and openness, and the consequences of this failure to plan ahead and work openly will be felt by many individuals and families, and across the higher education sector for years to come.
But how to do it differently?
- Firstly, the underlying models, assumptions and success criteria could have been made available for external scrutiny and challenge.
- Secondly, the system should have been designed inclusively and robustly tested to make sure it accommodated the outliers and wildcards.
- Thirdly, the real-world consequences of introducing a new, untested model of assessment should have been recognised. The appeals process should have been set up and communicated in advance, and a buffer period imposed on universities confirming places.
- And lastly, there could have been clear, frequent communication.
This embarrassing litany of process failure has made thousands of teenagers determined to 'Fuck the algorithm', and frankly – who can blame them?
Swee Leng Harris, Luminate
The government and public agencies must act in accordance with the law, including when using algorithmic systems to assist or carry out their functions – this principle is at the heart of the rule of law. There was a lack of due consideration by Ofqual of two key aspects of law while developing and deploying the algorithmic grading system.
...government and public agencies must comply with the law and in order to do that they need to understand the applicable laws and how algorithmic systems operate
First, the nature and scope of Ofqual’s public powers and function as defined by law merited deeper consideration in order to answer the question of whether the algorithmic grading system would fulfil Ofqual’s public function in accordance with the law. Ofqual understood the task of standardising grades as necessary for its statutory objective to maintain standards, cited in section 5.1 of their report published 13 August.
But, as argued in Foxglove’s case for Curtis Parfitt-Ford, the algorithmic system graded individuals based on the historic performance of their school and the rank of the individual relative to other students at their school, which was not a reliable indication of an individual student’s knowledge, skills, understanding or achievement as required under the Apprenticeships, Skills, Children and Learning Act 2009. The failure to comply with this statutory objective is illustrated by stories such as Abby from Bosworth who received a U in maths because, historically, someone from her school had received a U. Abby was predicted at B or C, but because of her rank in class the historical model dictated she receive U.
Second, in their impact assessment, Ofqual failed to understand Article 22 of the GDPR [General Data Protection Regulation] on automated decision making and how it applied to the algorithmic grading system. Ofqual have not published their Data Protection Impact Assessment (DPIA), but let’s assume that they did one as required by Article 35 GDPR (as I have argued elsewhere, we should require that all government DPIAs be published). Instead they have published a Privacy Impact Statement (PIS), a simplified version of the data privacy impact assessment Ofqual says it did. The difference in language matters here: DPIAs are required to assess impact on all rights and freedoms, not just privacy and the GDPR.
In any case, the PIS asserts that Article 22 does not apply as there was human intervention in the inputs for the algorithmic grading system and signing off on final grades. Pointing to human involvement in inputs misunderstands what automated decision making is: once the inputs were provided the algorithmic system seems to have operated automatically to grade individuals, which is automated decision making. The human intervention in the signing off of grades could have been sufficient to preclude Article 22 from applying if there was meaningful human intervention – if all of the algorithmic awards were signed off without change then this would suggest that there was no meaningful human intervention and Article 22 applied.
Furthermore, Ofqual’s PIS asserts that the algorithmic grading system profiled centres and not individuals such that Article 22 did not apply, but this misunderstands how the system worked. As set out in the Foxglove/Parfitt-Ford claim, the algorithmic grading system profiled individuals based on the historic performance of their school and relative academic performance.
Notably, Ofqual did look at the equalities implications of the algorithmic grading system in section 10 of their 13 August report. There will be different views on whether this analysis accurately applied the Equalities Act and therefore whether the conclusions were correct, but Ofqual certainly thought in detail about their Public Sector Equality Duty.
The broader lessons to be learned from this incident are simple: government and public agencies must comply with the law and in order to do that they need to understand the applicable laws and how algorithmic systems they plan to use operate. This includes not only understanding the technology and data science, but also the system as a whole including the role of people and organisations interacting with the technology, in order to understand the social impact of a system and whether it complies with the law.
Cori Crider, Foxglove
This fiasco was political, not technical. Consider the likely response had the government stated its objectives in plain English months ago, like this:
To avoid a one-time uptick in grade inflation during the pandemic, we’ve decided to substitute teachers’ grades with a statistical prediction based on your school’s historic performance. This will limit inflation, but lead to individually unfair results, such as downgrading bright students in large subjects and struggling schools.
Had the government been open about what it had prioritised – and who it had decided to leave behind – the result might well have been better, by forcing a course correction before it was too late.
For decisions that affect life chances, are algorithmic systems democratically acceptable at all?
Instead, ironically, the government’s choice will now increase grade inflation (because in the wake of the backlash, all algorithmic upgrades are being retained).
What should we learn from this?
No more permissionless systems. No one bothered to explain algorithmic grading to people – no one sought real public assent before doing it. None should suggest the Ofqual ‘consultation’ process offers an excuse. Policy consultations of this kind are tracked and engaged with by a rarefied cohort of experts. But it was not the public’s job to submit statistical analyses to the Ofqual consultation website. For decisions which affect the life chances of thousands, meaningful democratic engagement is not a step you can skip.
Don’t be afraid of scrutiny. As it happens, senior professors from the Royal Statistical Society did spot risks early and offered to assist. As the price of admission, Ofqual demanded a five-year silence about flaws in the algorithm. Understandably, those experts refused. This was a missed opportunity.
State your purpose honestly. Decision-makers seemingly hoped that if grades were handed down like an edict, either few would notice, or people would acquiesce. (In a way one can see how the error was made: many permissionless algorithmic systems have been rolled out in British public life in recent years, generally to manage people with less political clout than the nation’s parents and students.) That has backfired spectacularly. If you seek to hide a contestable policy choice behind a technical veneer, you risk being caught.
Don’t assume the algorithm. Those of us in the tech justice (or ‘tech ethics’) fields would do well to reflect on this moment. Too often we have limited ourselves to debating the ‘how’ of algorithmic sorting: how to reduce bias, increase transparency, open appeal routes, and so on. Procedural protections can matter and, if systems cannot be avoided, set a floor of fairness. But they elide a first-order question: for decisions that affect life chances, are algorithmic systems democratically acceptable at all? We have rejected the algorithm for this year’s grades. Is one appropriate for visas? Policing? Benefits? Only when that has been answered do subsidiary questions about ‘fairness, accountability, and transparency’ in algorithms arise. More of us should engage with this core democratic question.