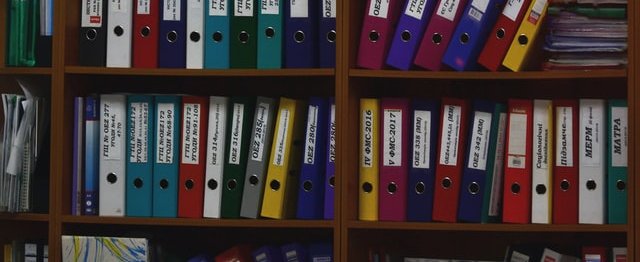
This essay was written by Eleanor Shearer as part of our ODI Writers' Fund for Black History Month 2020.
About Eleanor
Eleanor is a mixed race writer and consultant with a particular interest in racial justice. She has a Master’s degree in Political Theory, where she specialised in the history of Caribbean slavery and the politics of reparations. She now works for a small consultancy that helps public sector organisations harness the power of new technologies such as AI, and in this role she has published a report on racial bias in Natural Language Processing. She also writes fiction, with her first flash fiction piece published in The Fiction Pool this October.
The dividing line: how we represent race in data
The fierce colonial desire to divide and classify, to create hierarchies and produce difference, leaves behind wounds and scars.
Achille Mbembe, ‘Critique of Black Reason’
If knowledge is power, then data may be one of the most potent forces of our age. As advances in technology increase our ability to capture and process information about the world we live in, data can tell a compelling story. In the middle of a pandemic that has disproportionately killed people of colour across the Western world (though that has had a far milder effect in other areas such as the African continent), and at a time of global protest over the treatment of Black people, data has been used time and again to shed light on racial inequality.
At the same time, if we are not careful, the sort of thinking that data encourages – grouping and comparing different parts of the population – can end up replicating racist logics. It is no coincidence, after all, that one of the fathers of social statistics, Francis Galton, was also a famous eugenicist. Race in data treads a delicate line between the history of colonial classification and the need to understand racial injustice. Race is not ‘real’ in the sense that many other things we might capture in data are ‘real’ – such as someone’s age, or where they live. But race still matters. Race is a biological fiction made social fact – it does not exist in the sense that it says anything meaningful about the bodies, minds and genetic make-up of different people, and yet it continues to have a huge effect on people’s lives.
The point of this essay is to encourage a critical approach to the relationship between race and data. It points to three questions that anyone working with data should ask if they are going to be collecting and using data about race.
What are you actually measuring?
Race is a complex, multi-dimensional concept, and it can also intersect with many other features of a person, such as nationality, immigration status and class. Understanding this complexity and being specific about which element(s) of race are being measured will make for better analysis.
What categories are most appropriate?
The boundaries between different racial groups are arbitrary, have shifted throughout history, and can still shift according to context. Broad categories – like ‘BAME’ – might be able to avoid some of the ambiguity of specific distinctions between racial or ethnic groups, but they also come with their own costs if they obscure more than they reveal. There is no right answer in terms of the categories into which data about race should be grouped, but researchers will need to think carefully about the appropriateness of whichever approach they choose.
Should this data be collected at all?
Data about race can be a powerful challenge to racial inequality, but in the wrong hands it can also have fatal consequences. Given the sensitivity of data about race, and the fraying of trust that can occur if particular groups feel profiled and targeted, researchers will need to consider whether they should in fact be collecting data about race at all, and if they are the right people to collect it.
A brief history of race
The racial categories we use today are neither natural nor inevitable. Where we now recognise a fairly small number of races, medieval and early modern authors identified many more. In the Omnium gentium mores written in 1520 by Johann Boemus, the people of Africa, rather than being simply ‘Black’, were divided into many tribes, including Ethiopians, Egyptians, Troglodytes, Cynnamies, Ryzophagi (eaters of roots), Icthiophagi (eaters of fish), and more.
It was over the 18th Century that the modern idea of race began to form. Classifications of the world’s population began to coalesce around a finite – and small – number of categories. For example, according to Swedish naturalist Carl von Linné, writing in 1735, there were only four races: European, American, Asian and African. This new idea of race at once divided – proposing sharp differences between the civilised Europeans and the savage people they conquered, killed and enslaved – and united – bringing together millions of people across entire continents under a single label like Black.
This new approach to race coincided with the expansion of European colonialism and the Trans-Atlantic slave trade. Slavery, by design, stripped African people of their names, clothes, and traditions, and those enslaved often had to adopt new creole languages to be able to communicate with each other. Meanwhile, in the New World, previously recognised differences between the different Native American tribes collapsed into the general epithet of ‘savage’ to justify conquest. The growth of scientific racism also hardened the idea of difference between groups, that the white race was somehow genetically superior to all other races.
After the atrocities committed by the Nazis in the middle of the 20th Century, scientific racism and its strictly biological account of race has fallen out of fashion (although it has not disappeared). Modern accounts of race tend to focus more on culture and identity than on genetics. For example, the UK Census, rather than measuring race, measures ethnicity, which they define as ‘more subjective – it relates to a shared history and culture, language, religion and traditions, as well as skin colour.’
A history of race, and all the ways it has changed over time, reminds us that we are dealing with a concept that is fluid rather than fixed. But it should also caution us to remember that race has multiple meanings, and there are multiple, often overlapping, ways of dividing the world into different races. It is precisely this ambiguity that makes the codification of race in data so challenging.
One concept, many dimensions
Race does not refer to a single characteristic, but instead to many different parts of a person’s appearance, ancestry and identity. Most obviously, race is about phenotype – the different visual features we associate with race. Skin colour, hair texture, facial features, and more, will all play a role when we make an assessment of someone’s race. However, phenotype alone does not constitute race.
Race is also about ancestry, although crudely so – more metaphorical ancestry than genetic, as when Africans were assumed to be the descendants of Ham. In the face of growing mixed-race populations in colonies, some light enough to ‘pass’ for white, Europeans had to come up with a justification for their superiority that did not rest on phenotype alone. ‘One-drop’ laws across the Caribbean and the American South suggested that having even ‘one drop’ of Black blood was enough to classify someone as Black.
Race, in the modern sense, rests heavily on self-identification. Censuses and surveys today typically require someone declaring their own race rather than having an interviewer or researcher assume it based on appearance. Race as self-identification depends on the complex interplay of phenotype and family. Those that look white may choose to identify as Black based on having Black ancestry, just as the old ‘One-drop’ laws might classify them as such, and someone mixed-race might choose to identify as Black or Asian based on their appearance. However, self-identification has its limits, as the outcry over phenotypically and ancestrally White Jessica Krug and Rachel Dolezal ‘passing’ as Black attests. We still have a sense that self-identification must depend on something ‘real’, whether that is ancestry or appearance; identity alone cannot trump race’s other dimensions.
Causation and conceptual confusion
Once we understand the history of race, as a term that has meant everything from someone’s genes to their sense of their own identity, we are better equipped to see flaws in data analysis that uses race as a variable. There are two issues that researchers should seek to avoid: treating race as a cause, and treating the various dimensions of race as interchangeable.
Treating race as the cause of any inequalities that emerge in data can end up reinforcing the biological account of race. This is particularly a problem in medical research, where numerous studies show differential health outcomes based on race. In her book Superior: The Return of Race Science, Angela Saini documents the persistent search in the US for the ‘Black gene’ that might be behind higher rates of hypertension in the African American community. In one particularly bizarre strand of research, based on a 1725 engraving of a white Englishman licking an enslaved African, the hypothesis is that slaves with an ability to retain salt better were more likely to survive the journey to the Americas. Eating too much salt can make hypertension worse, and so if African Americans today retain this higher ability to retain salt, this might make them more at risk.
However well-meaning in their attempts to address a serious health problem amongst African Americans, such research perpetuates the idea that race is biological – that Black bodies are just different to white ones. Instead of looking for a particular gene, researchers would be better placed to look at experiences of discrimination, employment patterns, and many other factors that could be an underlying cause of particular inequalities. Race is ‘real’ in the sense that it has structured our societies in particular ways, but any analysis that stops with race and does not go deeper into these social structures will most likely be unable to challenge racial inequality.
Besides treating race as a cause, another major issue in data analysis that uses race as a variable is a lack of conceptual clarity. The different elements of race, from self-identification to ancestry to appearance. These different elements may overlap, but they also may not, with the result that data about race might refer to subtly different phenomena.
This may not seem important, but it can have an effect on data analysis. For example, one study found that estimates of income inequality between white and ‘brown’ (mixed race) Brazilians differ depending on the way the data about race is collected. Using official statistics, based on self-identification, produces a smaller estimate of the pay gap than using observed race, where interviewers classified participants.
Another study found – perhaps unsurprisingly – that observed race mattered more than self-classification when it came to increased risk of arrest. Police were more likely to arrest those that they perceived as Black, whether or not that is how they actually identified, and identifying Black but not being perceived as such did not lead to increased risks of arrest. These findings remind us that there is no such thing as ‘data about race’, only data about different elements of race. Using the wrong sort of data could lead to misleading or inaccurate findings.
These challenges lead us to the first question researchers should be asking: what are you actually measuring? By being specific about the element of race under consideration, researchers can avoid the conceptual messiness of using race with no definition at all. A good example of this in practice is Joy Buolamwini and Timnit Gebru’s paper on racial bias in facial recognition. Here, the authors talk in terms of skin colour rather than race. They explain that this choice is due to the fact that facial recognition systems may perform well on light-skinned people of colour, and so any analysis that considers the accuracy of a system on, say, Black people as a whole might end up masking how poorly the system fares on darker skin.
Being specific can also help to avoid the pitfalls of biological essentialism. If research is measuring whether being perceived as Black makes a difference to health outcomes, this is a measure of the experience of discrimination more than it is about particular genes. Decomposing race into its various parts is a reminder that we are dealing with a messy social construct, not neat biological categories.
However, before even reaching the data analysis stage, there is another thorny issue with which researchers must grapple. As we have seen, throughout history many different taxonomies of humanity have been proposed, drawing boundaries in different places and producing different numbers of racial groups. In the following sections, we will consider this second question: what categories are most appropriate?
Lumping together
‘How many Black people are in the current Cabinet?’ Sky News’ Sophy Ridge asked Matt Hancock during an interview in June.
‘Well, there are many people from a Black and Minority Ethnic background,’ came Hancock’s reply. And he is right – the current UK Cabinet includes Rishi Sunak, Priti Patel, Alok Sharma and Suella Braverman, all from British Asian backgrounds. However, it contains no Black people.
Hancock’s evasive answer hints at a problem with broad racial categories such as ‘BAME’. Sometimes, they obscure more than they illuminate. They assume that all the various groups contained within them are interchangeable, at least for the purposes of whatever analysis being conducted – that having a high-proportion of BAME employees is evidence of being diverse, for example, even if some groups are still highly underrepresented. In some ways, these composite categories are the ultimate culmination of the colonial logic, that the differences within categories matters less than what they share – that they are not white.
However, this may be exactly the point. Many umbrella terms for non-white racial groups were originally intended to foster political solidarity and to highlight a common experience of racism. In the UK in the late 1970s, the term ‘politically Black’ arose to cover the various ethnic minorities who had often moved to the UK from the Commonwealth after the Second World War, including West Indians, Pakistanis, Bangladeshis and Indians. It was an attempt to foster a critical racial consciousness among disparate groups and build a movement to challenge racism. Similarly, the term ‘Asian American’ – not as broad as BAME, but still encompassing a huge breadth of identities – has activist origins. It was coined by graduate students Emma Gee and Yuji Ichioka in 1968, as a way to bring different peoples of Asian descent together.
As acts of solidarity, these terms may be useful. However, for analysis of inequality, without disaggregated data they might well be so broad as to be misleading. For example, data that shows Asian Americans achieving some of the highest SAT scores on average might obscure serious educational disadvantages faced by Laotian Americans. Even labels that might seem more specific, such as ‘Black’, might need to be disaggregated for data analysis – in the UK, there is a significant educational attainment gap between students of Black African and of Black Caribbean descent. Ideally, researchers need to collect and analyse data with far more precise categories than ‘BAME’ or ‘Asian’, and only use these categories in their final analysis if doing so does not obscure any important differences within the groups in question.
Splitting apart
If broad labels can be misleading, more specific racial categories can have their own challenges, too. Given that racial boundaries are never fixed, adding more and more categories can risk alienating those who feel that they belong to multiple groups. Researchers should be willing to accommodate such multiple or overlapping identification in their analysis if they are going to use a large number of different options for race.
We can see the issues around overlapping identification playing out in the consultations by the UK’s Office for National Statistics (ONS) for the 2021 Census. When ONS consulted the Sikh community on adding a ‘Sikh’ tick-box option to the Census, found that some participants ticked both the ‘Indian’ and ‘Sikh’ boxes when both were presented, viewing both aspects of their identity as important. Ticking multiple boxes is not currently an option in Censuses, meaning that this response would not be recognised, and so ONS decided against including a ‘Sikh’ option in 2021.
ONS also consulted on whether to include ‘Jewish’ as a tick-box option for ethnicity in 2021. Their findings do not mention whether they consulted Black Jews specifically, but it is interesting when testing this option, it was either included in the white or the ‘other section’ of the census. Either of these options, but especially presenting Jewish as a White identity, would exclude Black Jews, a minority who often already feel as though their identities are erased. The findings of the ONS consultations show us that having larger numbers of racial categories is not always the answer if these categories are not flexible enough to allow for multiple identification.
The price of visibility
The findings of the ONS’s consultations for the 2021 Census throw up another issue relating to collecting data about race and ethnicity. The ONS found that both Jewish and Somali participants expressed suspicion about why data about their ethnicity was needed and pointed to histories of discrimination against their communities. Their anxieties point to the dark side of collecting data about race – that it will be used to target particular racial groups. These anxieties remind us of another question that researchers should be asking – should this data be collected at all?
The French and the German governments do not collect any data about race. In both states, the horrors inflicted on minority populations by the Nazis are still within living memory, and rested on an extreme logic of racial and ethnic classification and extermination. However, without data it can be hard to compile evidence of racial inequality. There is a long tradition of data analysis being linked to anti-racism, starting with W. E. B. DuBois’ data visualisations that told the story of racial inequality in the US. The organisation Data for Black Lives cites DuBois as an inspiration for their work using data science to empower Black people.
The limits of the French and German model have been tested this summer, as Black Lives Matter protests spread all over the world. In France, government spokeswoman Sibeth Ndiaye suggested that collecting racial data could allow policymakers to ‘measure and look at reality as it is,’ although an adviser to Macron indicated this was not something the President would be pursuing. Meanwhile, in Germany, the Afrozensus was an online survey run between July and September 2020 to gather data about the discrimination faced by Germans of African descent.
Afrozensus received funding from the German state, but ultimately was responsible for its own data management and storage. Like Data for Black Lives, it was not a government operation. It may be the case that, for many marginalised groups, historic mistrust of a state that has mistreated them mean that community-based organisations are better placed to gather data about race.
Conclusion
Data can and should be an important tool in the anti-racist struggle. However, we must all be careful not to return to colonial logics of classification and control. Researchers must use data about race sensitively and in full awareness of how the concept came to be and the elements that make it up. This essay has put forward three questions they should be asking; there are likely many more, because race and racism are not straightforward. However, from this foundation, we can begin to build a better approach to data and race.
Ultimately, data analysis always involves an element of generalisation. We are all individuals, and are not reducible to our gender, race, sexuality, income, or any of the other categories into which we might be sorted. However, lazy generalisations, especially where race is concerned, will do little to advance racial equality. If we are not careful, data can divide and sort us in exactly the sort of essentialising ways that the colonial idea of race supported. But if researchers ask the right questions, and know their history, we can use data to advocate for racial justice.