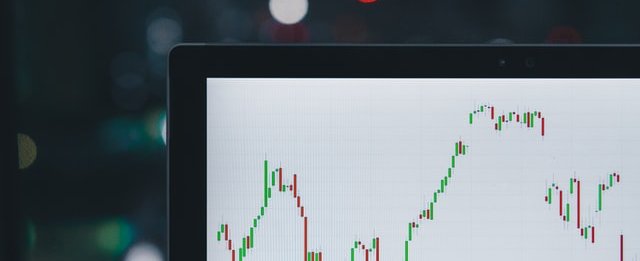
How can we improve AI and data technologies in health so that they benefit everyone?
Artificial intelligence (AI) and data technologies are transforming research and healthcare. According to the World Economic Forum, by 2030 AI will access multiple sources of data to reveal patterns in disease and aid treatment and care, reduce waiting times for patients, improve efficiency in hospitals and health systems, and suggest preventive measures.
The Data for science and health team at Wellcome are interested in enabling these technologies in a way that benefits everyone. But they have identified the following issues are significant blockers to achieving this:
- unrepresentative datasets
- lack of public trust
- health data can be messy and complex
- lack of data science capacity worldwide
Data-science challenge prizes
As part of its programme to address these issues, Wellcome has asked the ODI to undertake some research to identify opportunities for running a series of data-science challenge prizes.
Challenge prizes are a great way to draw attention to an issue and motivate a wide range of people to come up with innovative approaches and solutions. A data-challenge prize asks innovators to find new ways to use data and data science to address a challenge, often combining non-traditional with traditional sources of data and using novel analytical methods to reframe issues and shed new light on seemingly intractable problems. Because combining data sources can provide more complete, more timely, and/or more granular information about an issue, data innovation can open opportunities for more cost-effective interventions, as well as providing entirely new insights that may have been overlooked through traditional approaches.
The ODI’s mission is to create open and trustworthy data ecosystems, which includes enabling the use of data to inspire and fuel innovation. Since the launch of the ODI startup incubator in 2012, we have run 11 different programmes using different innovation approaches: competitions, incubators, challenge series, and accelerators, working across Europe, Central America and the ASEAN region.
Working with Wellcome, we are bringing that experience to see how we can use challenge prizes to make a difference in healthcare. The Wellcome data science challenge prizes will aim to:
- equip and motivate data scientists to innovate with health data for public good in collaboration with health practitioners and patients
- engage the public and patients groups to build trust, understanding and participation in health data innovation
We also think it’s important that these prizes have a longer-term impact, so we’re building in time for improving data quality and access to data, and taking any solutions that arise from the challenges into operation.
What we’ve been doing
The first phase of the project saw us develop a 'theory of change' for the prizes, teasing out exactly what we want to achieve and how. This gave us a framework to think through potential challenge prize topic areas. The key elements of consideration we identified are:
- Compelling health problems which can be addressed through data science – every prize needs a very clear and compelling problem. In this case, it is vital that it is a health problem not a data problem. The problem needs to be broad enough to have space for a variety of approaches and solutions, but specific enough that we can see measurable change through the work. To understand the types of opportunities for using data science in healthcare, we looked to the work of Understanding Patient Data who provide a framework for thinking about the impacts of data in healthcare, supporting:
- individual care
- understanding of disease
- evaluating policy
- planning health services
- diagnosis
- treatment and prevention
- patient safety
- Enable access to existing data – there is a huge amount of health data collected every day around the world, but it is very hard to get access to it, and very hard to use it in combination with other data sources. These challenge prizes are not here to create new datasets but will need to ensure the participants of the challenge can focus on what they can do with the data, not how to get hold of it.
- Supporting the best people to apply – challenge prizes are a fantastic way of drawing attention to an issue and attracting unconventional approaches to them through the draw of a monetary prize and recognition of an award. However, one of the legitimate criticisms of challenge prizes is that they ask participants to do a lot of speculative work for free, without a guarantee they will win the prize and be able to cover the costs of their participation. This can often mean that it is only participants in well funded private sector or academic organisations who can have the leeway to participate. This undermines the core principle of open innovation which aims to support a diverse group of innovative thinkers. We are looking at different kinds of models for challenge awards – not just prizes at the end – to get around this problem.
- Enabling multidisciplinary teams – if one of the key challenges we are looking to address is that there is data science capability in the world, but not in the health space, then it is crucial to enable and support people from the data science and health disciplines to work together to share their expertise. Working across disciplines can be hard so providing training and support is key. If done well, could build communities of practice around a specific topic in a specific location.
- Work with patients and the public to shape and run the challenges – to address the aim of developing public trust in health data innovation, we realised that we would need more than the creation of shiny AI applications: we would need to ensure that patient groups, medical practitioners and anyone else who could be a beneficiary of a particular challenge into the design and the delivery of that challenge.
Once we had established our objectives, we needed to find some potential topics. Through desk research, interviews and workshops with experts from across the data, health and development space as well as health practitioners across UK, sub-Saharan Africa and India, we developed a longlist of topics which included topics like:
- Predicting risk for multimorbidities
- Impact of air pollution on children's environmental health
- Diagnosis and treatment of neurodegenerative non communicable diseases (such as Alzheimers or Parkinson’s)
- Access to services in rural areas in order to support better maternal early child health
Where we are now
Each item on the long list was scored against a series of criteria including the relevance and appeal of the problem, the availability of data, the scale of potential impact, how measurable the impact might be and any legal or ethical barriers. These scores were then discussed with Wellcome and four key topics were identified for the team to continue researching.
- Addressing depression and anxiety for young people in the UK Depression and anxiety are among the largest contributors to the global burden of ill mental health. 75% of mental illnesses start before the age of 18, with consequences that are often lifelong. Many of the children and adolescents who are at risk of developing anxiety and depressive disorders do not seek out any form of support, and for those that do, interventions are often ineffective, or a short term solution.By combining the use of cohort data with real world evidence, a data science challenge prize could enable the creation of new insights about anxiety and depression in young people. Areas of focus could include better detection of early signs of anxiety and depression, better planning of school based mental health and well being interventions, and improved medical insights into the relationship between various symptoms for both anxiety and depression.
- Improving snakebite prediction in India Snake bites kill more than 120,000 people and leave 400,000 people with life changing disabilities every year globally, which is equivalent to prostate or cervical cancer. Epidemiological data of snakebite incidence is not readily available. Health systems could optimise the distribution of antivenom and other resources if they had better prediction of the incidence of snakebites, risk of envenoming and risk of mortality.Using data science to improve the epidemiology of snakebite could directly support the healthcare system better plan and respond to instances of snakebite, for example with better antivenom distribution and management, ultimately leading to a reduction of snakebite mortality and morbidity in India.
- Remote sensing for public health in slums and informal settlements Over 55% of the world’s population live in urban areas, a proportion that is expected to increase to 68% by 2050, 90% of which is predicted to be in Lower and Middle Income Countries. In sub-Saharan Africa, 62% of the urban population lives in slums with an increased risk of infectious diseases due to lack of services and infrastructure. There is significant data available that can provide area level insights for public health indicators such as solid waste, but the people involved with urban health indicator development tend to have health and medical backgrounds and are unaware of, or are untrained in the use of, the newer types of data that can measure neighbourhood-level phenomena (for example, satellite imagery, social media).A data science challenge has the potential to enable access to a combination of geospatial, earth observation and big data in tandem with epidemiological data. Bringing together data scientists, urban health experts and community organisers to use this data to create models and tools to better monitor public health risk factors (eg: solid waste and pollution). This could be useful for community groups, city planners, public service delivery, national policy, academia and the development community.
- Drug resistant infections in Africa Antimicrobial resistance is one of the major public health concerns of this century. Antibiotics have been a staple of modern medicine's toolkit to fight illnesses, but antibiotic treatment has become less reliable as antimicrobial resistance has evolved in many bacterial pathogens, causing the need for costly new treatments to be developed, while antimicrobial resistant organisms continue to take thousands of lives per year.Building on the AMR Register, a pilot project ODI delivered in partnership with Wellcome’s Drug Resistant Infections team which gathered and openly publishing data on industry sponsored human antimicrobial surveillance programmes, this data challenge would aim to develop data science capability in Kenya, Malawi and Uganda to address specific issues in those regions. For example, in some areas, antibiotics are available over the counter with no information for the public on which drugs are effective.
Next steps
From now until the end of May, we’ll be continuing to work on these four topics to understand the data landscape, identify potential partners and participants, and make recommendations on how a data science challenge could work for those problems in those areas.
If you have any feedback, suggestions or would like to get involved, please contact us.